
BOOKS - Privacy-Preserving Machine Learning: A use-case-driven approach to building a...

Privacy-Preserving Machine Learning: A use-case-driven approach to building and protecting ML pipelines from privacy and security threats
Author: Srinivasa Rao Aravilli
Year: May 24, 2024
Format: PDF
File size: PDF 14 MB
Language: English
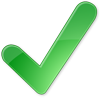
Year: May 24, 2024
Format: PDF
File size: PDF 14 MB
Language: English
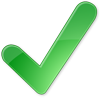
Book Description: PrivacyPreserving Machine Learning: A Use-case driven approach to building and protecting ML pipelines from privacy and security threats In today's digital age, technology has become an integral part of our lives, and machine learning (ML) has revolutionized the way we approach problem-solving in various industries. However, as technology evolves, so do the risks associated with it. With the increasing use of ML in various applications, there is a growing concern about data privacy and security breaches. As a result, organizations must ensure that their ML pipelines are built and protected from potential threats to maintain the trust of their customers and stakeholders. This book, "PrivacyPreserving Machine Learning provides a comprehensive guide on how to build and protect ML pipelines from privacy and security threats while ensuring compliance with privacy regulations such as CCPA and GDPR. The Need for Privacy Preservation in ML The need for privacy preservation in ML stems from the fact that large amounts of sensitive data are being analyzed to predict various insights. This data can include personal information, financial records, health records, and other confidential information that must be protected. Moreover, the lack of in-depth expertise in privacy-preserved ML makes it challenging for software engineers, data scientists, and ML engineers to analyze this data without compromising its privacy.
КонфиденциальностьСохранение машинного обучения: Подход, основанный на сценарии использования, к построению и защите конвейеров ML от угроз конфиденциальности и безопасности В современную цифровую эпоху технологии стали неотъемлемой частью нашей жизни, а машинное обучение (ML) произвело революцию в подходе к решению проблем в различных отраслях. Однако по мере развития технологий растут и риски, связанные с ними. С ростом использования ML в различных приложениях растет беспокойство по поводу конфиденциальности данных и нарушений безопасности. В результате организации должны гарантировать, что их конвейеры ML построены и защищены от потенциальных угроз, чтобы поддерживать доверие своих клиентов и заинтересованных сторон. Эта книга «КонфиденциальностьСохранение машинного обучения» содержит исчерпывающее руководство по созданию и защите конвейеров ML от угроз конфиденциальности и безопасности, обеспечивая при этом соблюдение правил конфиденциальности, таких как CCPA и GDPR. Необходимость сохранения конфиденциальности в ML Необходимость сохранения конфиденциальности в ML проистекает из того факта, что большие объемы конфиденциальных данных анализируются для прогнозирования различных инсайтов. Эти данные могут включать личную информацию, финансовые записи, медицинские записи и другую конфиденциальную информацию, которая должна быть защищена. Кроме того, отсутствие глубоких знаний в области ML с сохранением конфиденциальности затрудняет анализ этих данных инженерами по программному обеспечению, специалистами по обработке данных и инженерами ML без ущерба для их конфиденциальности.
ConfidentialitéPréserver le Machine arning : une approche basée sur un scénario d'utilisation pour construire et protéger les convoyeurs ML contre les menaces à la vie privée et à la sécurité À l'ère numérique moderne, la technologie est devenue une partie intégrante de nos vies et le Machine arning (ML) a révolutionné l'approche de résolution de problèmes dans diverses industries. Cependant, à mesure que la technologie progresse, les risques qui y sont associés augmentent. Avec l'utilisation croissante de ML dans diverses applications, l'inquiétude concernant la confidentialité des données et les violations de la sécurité augmente. En conséquence, les organisations doivent s'assurer que leurs convoyeurs ML sont construits et protégés contre les menaces potentielles afin de maintenir la confiance de leurs clients et des intervenants. Ce livre « PrivacySaving Machine arning » fournit des conseils exhaustifs sur la façon de créer et de protéger les convoyeurs ML contre les menaces à la vie privée et à la sécurité, tout en veillant au respect des règles de confidentialité telles que la CCPA et le RGPD. Nécessité de préserver la confidentialité dans les LM La nécessité de préserver la confidentialité dans les LM découle du fait que de grandes quantités de données confidentielles sont analysées pour prédire les différents insignes. Ces données peuvent comprendre des renseignements personnels, des dossiers financiers, des dossiers médicaux et d'autres renseignements confidentiels qui doivent être protégés. En outre, le manque de connaissances approfondies dans le domaine de la LM, tout en préservant la confidentialité, rend difficile l'analyse de ces données par les ingénieurs logiciels, les professionnels du traitement des données et les ingénieurs de la LM, sans compromettre leur confidentialité.
PrivacidadPreservación del aprendizaje automático: Un enfoque basado en escenarios de uso para construir y proteger los transportadores ML de amenazas a la privacidad y la seguridad En la era digital actual, la tecnología se ha convertido en una parte integral de nuestras vidas, y el aprendizaje automático (ML) ha revolucionado el enfoque para resolver problemas en diferentes industrias. n embargo, a medida que la tecnología avanza, también aumentan los riesgos asociados con ella. Con el uso creciente de ML en varias aplicaciones, crece la preocupación por la privacidad de los datos y las violaciones de seguridad. Como resultado, las organizaciones deben garantizar que sus transportadores ML están construidos y protegidos contra posibles amenazas para mantener la confianza de sus clientes y partes interesadas. Este libro «PrivacidadPreservación del aprendizaje automático» proporciona una guía exhaustiva para crear y proteger los transportadores ML contra amenazas a la privacidad y la seguridad, al tiempo que garantiza el cumplimiento de las reglas de privacidad como CCPA y GDPR. La necesidad de mantener la confidencialidad en ML La necesidad de mantener la privacidad en ML se deriva del hecho de que grandes cantidades de datos confidenciales se analizan para predecir diferentes puntos de vista. Estos datos pueden incluir información personal, registros financieros, registros médicos y otra información confidencial que se debe proteger. Además, la falta de conocimientos profundos sobre ML con privacidad hace que sea difícil para los ingenieros de software, técnicos de procesamiento de datos e ingenieros de ML analizar estos datos sin comprometer su privacidad.
Manter a aprendizagem de máquinas: Abordagem baseada em cenários de uso para construir e proteger as linhas de montagem ML contra ameaças de privacidade e segurança Na era digital moderna, a tecnologia tornou-se parte integrante das nossas vidas, e a aprendizagem de máquinas (ML) revolucionou a abordagem de problemas em vários setores. No entanto, à medida que a tecnologia avança, os riscos associados também crescem. Com o aumento do uso de ML em vários aplicativos, a preocupação com a privacidade de dados e as falhas de segurança aumentam. Como resultado, as organizações devem garantir que suas linhas de montagem ML são construídas e protegidas contra potenciais ameaças para manter a confiança dos seus clientes e interessados. Este livro «A Preservação da Aprendizagem de Máquinas» fornece um guia abrangente para criar e proteger as linhas de montagem ML contra ameaças de privacidade e segurança, garantindo que as regras de privacidade como CCPA e GDPR sejam respeitadas. A necessidade de preservar a privacidade no ML A necessidade de preservar a privacidade no ML decorre do fato de que grandes quantidades de dados confidenciais são analisados para prever vários insights. Estes dados podem incluir informações pessoais, registos financeiros, registros médicos e outras informações confidenciais que devem ser protegidas. Além disso, a falta de conhecimento profundo do ML, mantendo a privacidade, dificulta a análise desses dados por engenheiros de software, especialistas em processamento de dados e engenheiros ML, sem comprometer sua privacidade.
Conservazione dell'apprendimento automatico: l'approccio basato su scenari di utilizzo alla costruzione e alla protezione delle reti di montaggio ML contro le minacce alla privacy e alla sicurezza Nell'era digitale moderna, la tecnologia è diventata parte integrante della nostra vita, mentre l'apprendimento automatico (ML) ha rivoluzionato l'approccio alla risoluzione dei problemi in diversi settori. Tuttavia, con l'evoluzione della tecnologia, anche i rischi associati aumentano. Con l'aumento dell'utilizzo di ML in diverse applicazioni, cresce la preoccupazione per la privacy dei dati e le violazioni della sicurezza. Di conseguenza, le organizzazioni devono garantire che le loro linee di montaggio ML sono costruite e protette da potenziali minacce per mantenere la fiducia dei clienti e delle parti interessate. Questo libro Salva apprendimento automatico fornisce una guida completa per la creazione e la protezione delle reti di montaggio ML contro le minacce alla privacy e alla sicurezza, garantendo al contempo il rispetto delle regole di privacy come CCPA e GDPR. La necessità di mantenere la privacy in ML La necessità di mantenere la privacy in ML deriva dal fatto che grandi quantità di dati sensibili vengono analizzati per prevedere diversi insight. Questi dati possono includere informazioni personali, documenti finanziari, cartelle cliniche e altre informazioni riservate da proteggere. Inoltre, la mancanza di conoscenze approfondite in materia di privacy rende difficile l'analisi di questi dati da parte di ingegneri software, esperti di elaborazione dati e tecnici ML senza compromettere la loro privacy.
DatenschutzMaschinelles rnen bewahren: Anwendungsfallbasierter Ansatz zum Aufbau und Schutz von ML-Pipelines vor Datenschutz- und cherheitsbedrohungen Im heutigen digitalen Zeitalter ist Technologie zu einem festen Bestandteil unseres bens geworden, und Machine arning (ML) hat den Problemlösungsansatz in verschiedenen Branchen revolutioniert. Mit fortschreitender Technologie wachsen jedoch auch die damit verbundenen Risiken. Mit der zunehmenden Verwendung von ML in verschiedenen Anwendungen wächst die Sorge um Datenschutz und cherheitsverletzungen. Infolgedessen müssen Organisationen sicherstellen, dass ihre ML-Pipelines aufgebaut und vor potenziellen Bedrohungen geschützt sind, um das Vertrauen ihrer Kunden und Stakeholder zu erhalten. Dieses Buch „PrivacyKeeping Machine arning“ bietet eine umfassende Anleitung zum Aufbau und Schutz von ML-Pipelines vor Datenschutz- und cherheitsbedrohungen und stellt gleichzeitig die Einhaltung von Datenschutzbestimmungen wie CCPA und DSGVO sicher. Die Notwendigkeit, die Privatsphäre in ML zu wahren Die Notwendigkeit, die Privatsphäre in ML zu wahren, ergibt sich aus der Tatsache, dass große Mengen an sensiblen Daten analysiert werden, um verschiedene Erkenntnisse vorherzusagen. Diese Daten können persönliche Informationen, Finanzunterlagen, Krankenakten und andere sensible Informationen enthalten, die geschützt werden müssen. Darüber hinaus erschwert der Mangel an fundiertem ML-Wissen unter Wahrung der Vertraulichkeit die Analyse dieser Daten durch Softwareingenieure, Datenspezialisten und ML-Ingenieure, ohne ihre Vertraulichkeit zu beeinträchtigen.
PrywatneUczenie maszynowe: Podejście do budowania i ochrony rurociągów ML przed zagrożeniami dla prywatności i bezpieczeństwa W nowoczesnej erze cyfrowej technologia stała się integralną częścią naszego życia, a uczenie maszynowe (ML) zrewolucjonizowało sposób, w jaki podchodzimy do problemów w różnych branżach. Jednak wraz z rozwojem technologii, podobnie jak ryzyko z nią związane. Wraz ze wzrostem wykorzystania ML w różnych aplikacjach wzrasta zaniepokojenie prywatnością danych i naruszeniami bezpieczeństwa. W rezultacie organizacje muszą zapewnić, że ich rurociągi ML są budowane i chronione przed potencjalnymi zagrożeniami, aby utrzymać zaufanie swoich klientów i zainteresowanych stron. Ta książka, „Prywatne uczenie maszynowe”, zapewnia kompleksowy przewodnik po budowie i ochronie rurociągów ML przed zagrożeniami prywatności i bezpieczeństwa przy jednoczesnym egzekwowaniu zasad ochrony prywatności, takich jak CCPA i RODO. Konieczność zachowania poufności w ML Konieczność zachowania poufności w ML wynika z faktu, że analizowane są duże ilości poufnych danych, aby przewidzieć różne spostrzeżenia. Dane te mogą obejmować dane osobowe, dokumentację finansową, dokumentację medyczną i inne informacje szczególnie chronione. Ponadto brak dogłębnej wiedzy ML przy zachowaniu poufności utrudnia inżynierom oprogramowania, badaczom danych i inżynierom ML analizę tych danych bez naruszania ich prywatności.
שימור מכונות למידה: שימוש במקרה גישה לבניית והגנה על צינורות ML מאיומי פרטיות וביטחון בעידן הדיגיטלי המודרני, הטכנולוגיה הפכה לחלק בלתי נפרד מחיינו, ולימוד מכונה (ML) חולל מהפכה בדרך בה אנו מתקרבים לבעיות ברחבי התעשיות. עם זאת, ככל שהטכנולוגיה מתפתחת, כך גם הסיכונים הקשורים אליה. עם השימוש הגובר ב-ML ביישומים שונים, ישנה דאגה גוברת בנוגע לפרטיות מידע ופרצות אבטחה. כתוצאה מכך, על הארגונים לוודא שצינורות ה-ML שלהם בנויים ומוגנים מפני איומים פוטנציאליים כדי לשמור על אמון הלקוחות ובעלי המניות שלהם. ספר זה, ”sserving Machine arning”, מספק מדריך מקיף לבניית והגנה על צינורות ML מפני פרטיות ואיומים ביטחוניים תוך אכיפת כללי פרטיות כגון CCPA ו-GDPR. הצורך לשמור על סודיות באם-אל הצורך לשמור על סודיות באם-אל נובע מהעובדה שכמות גדולה של נתונים סודיים מנותחת כדי לחזות תובנות שונות. מידע זה עשוי לכלול מידע אישי, רישומים פיננסיים, רישומים רפואיים ומידע רגיש נוסף שיש להגן עליו. בנוסף, היעדר ידע ML מעמיק תוך שמירה על סודיות מקשה על מהנדסי תוכנה, מדעני נתונים ומהנדסי ML לנתח נתונים אלה מבלי לפגוע בפרטיותם.''
PrivacyPreserving Machine arning: A Use Case Approach to Building and Protecting ML Pipelines from Privacy and Security Threats Modern dijital çağda, teknoloji hayatımızın ayrılmaz bir parçası haline geldi ve makine öğrenimi (ML), endüstrilerdeki sorunlara yaklaşım biçimimizde devrim yarattı. Ancak, teknoloji geliştikçe, onunla ilişkili riskler de artar. Çeşitli uygulamalarda ML kullanımının artmasıyla birlikte, veri gizliliği ve güvenlik ihlalleri konusunda artan bir endişe var. Sonuç olarak, kuruluşlar, müşterilerinin ve paydaşlarının güvenini korumak için ML boru hatlarının inşa edilmesini ve potansiyel tehditlerden korunmasını sağlamalıdır. Bu kitap, "PrivacyPreserving Machine arning", CCPA ve GDPR gibi gizlilik kurallarını uygularken ML boru hatlarını gizlilik ve güvenlik tehditlerinden korumak ve korumak için kapsamlı bir rehber sunar. ML'de gizliliği koruma ihtiyacı ML'de gizliliği koruma ihtiyacı, çeşitli içgörüleri tahmin etmek için büyük miktarda gizli verinin analiz edilmesinden kaynaklanmaktadır. Bu veriler kişisel bilgileri, finansal kayıtları, tıbbi kayıtları ve korunması gereken diğer hassas bilgileri içerebilir. Ayrıca, gizliliği korurken derinlemesine ML bilgisi eksikliği, yazılım mühendislerinin, veri bilimcilerinin ve ML mühendislerinin gizliliklerinden ödün vermeden bu verileri analiz etmelerini zorlaştırmaktadır.
PrivacyPreserving Machine arning: A Use Case Approach to Building and Protection ML Pipelines from Privacy and Security Receiving في العصر الرقمي الحديث، أصبحت التكنولوجيا جزءًا لا يتجزأ من حياتنا، وأحدث التعلم الآلي (ML L L L L L L L L LE E E E E E EAR) ثورة في طريقة التعامل مع المشاكل عبر الصناعات. ومع ذلك، مع تطور التكنولوجيا، تتطور المخاطر المرتبطة بها. مع تزايد استخدام ML في مختلف التطبيقات، هناك قلق متزايد بشأن خصوصية البيانات وانتهاكات الأمان. نتيجة لذلك، يجب على المؤسسات التأكد من بناء خطوط أنابيب ML الخاصة بها وحمايتها من التهديدات المحتملة للحفاظ على ثقة عملائها وأصحاب المصلحة. يوفر هذا الكتاب، «PrivacyPreserving Machine arning»، دليلاً شاملاً لبناء وحماية خطوط أنابيب ML من تهديدات الخصوصية والأمان مع تطبيق قواعد الخصوصية مثل CCPA و GDPR. تنبع الحاجة إلى الحفاظ على السرية في ML من حقيقة أنه يتم تحليل كميات كبيرة من البيانات السرية للتنبؤ برؤى مختلفة. قد تتضمن هذه البيانات معلومات شخصية وسجلات مالية وسجلات طبية ومعلومات حساسة أخرى يجب حمايتها. بالإضافة إلى ذلك، فإن الافتقار إلى معرفة ML المتعمقة مع الحفاظ على السرية يجعل من الصعب على مهندسي البرمجيات وعلماء البيانات ومهندسي ML تحليل هذه البيانات دون المساس بخصوصيتهم.
PrivacyPreserving Machine arning: 개인 정보 보호 및 보안 위협으로부터 ML 파이프 라인을 구축하고 보호하기위한 사용 사례 접근 현대 디지털 시대에 기술은 우리 삶의 필수 부분이되었으며 머신 러닝 (ML) 은 우리가 산업 전반에 문제기. 그러나 기술이 발전함에 따라 기술과 관련된 위험도 커집니다. 다양한 응용 프로그램에서 ML 사용이 증가함에 따라 데이터 개인 정보 보호 및 보안 침해에 대한 우려가 커지고 있습니다 결과적으로 조직은 고객과 이해 관계자의 신뢰를 유지하기 위해 ML 파이프 라인을 잠재적 위협으로부터 구축하고 보호해야합니다. 이 책 "PrivacyPreserving Machine arning" 은 CCPA 및 GDPR과 같은 개인 정보 보호 규칙을 시행하면서 ML 파이프 라인을 개인 정보 보호 및 보안 위협으로부터 구축하고 보호하는 포괄적 인 안내서를 제공합니다. ML에서 기밀을 유지해야 할 필요성 ML에서 기밀을 유지해야 할 필요성은 다양한 통찰력을 예측하기 위해 많은 양의 기밀 데이터가 분석된다는 사실에서 비롯됩니다. 이 데이터에는 개인 정보, 재무 기록, 의료 기록 및 기타 보호해야하는 민감한 정보가 포함될 수 있습니다. 또한 기밀성을 유지하면서 심층적 인 ML 지식이 부족하여 소프트웨어 엔지니어, 데이터 과학자 및 ML 엔지니어가 개인 정보를 손상시키지 않으면서이 데이터를 분석하기가 어렵습니다.
Privacy機械学習の維持:プライバシーとセキュリティの脅威からMLパイプラインを構築し保護するためのユースケースアプローチ現代のデジタル時代において、テクノロジーは私たちの生活の不可欠な部分となり、機械学習(ML)は私たちが業界全体の問題にアプローチする方法に革命をもたらしました。しかし、テクノロジーが進化するにつれて、それに伴うリスクも増大します。さまざまなアプリケーションでのMLの使用の増加に伴い、データプライバシーとセキュリティ侵害に対する懸念が高まっています。その結果、組織は、顧客やステークホルダーの信頼を維持するために、潜在的な脅威からMLパイプラインを構築し、保護する必要があります。この本「PrivacyPreserving Machine arning」は、CCPAやGDPRなどのプライバシー規則を適用しながら、プライバシーとセキュリティの脅威からMLパイプラインを構築し保護するための包括的なガイドを提供します。MLの機密性を維持する必要性MLの機密性を維持する必要性は、さまざまな洞察を予測するために大量の機密データが分析されることに由来します。このデータには、個人情報、財務記録、医療記録、および保護されなければならないその他の機密情報が含まれる場合があります。さらに、機密性を維持しながら詳細なML知識がないため、ソフトウェアエンジニア、データサイエンティスト、MLエンジニアはプライバシーを侵害することなくこのデータを分析することが困難になります。
隱私保護機器學習:一種基於用例的方法,用於構建和保護ML流水線免受隱私和安全威脅在當今的數字時代,技術已成為我們生活中不可或缺的一部分,機器學習(ML)徹底改變了解決不同行業問題的方法。但是,隨著技術的發展,與它們相關的風險也在增加。隨著各種應用程序中ML使用量的增加,人們越來越擔心數據隱私和安全漏洞。因此,組織必須確保其ML流水線已建成並免受潛在威脅,以保持客戶和利益相關者的信任。本書「隱私保護機器學習」提供了全面的指南,用於創建和保護ML流水線免受隱私和安全威脅,同時確保遵守CCPA和GDPR等隱私規則。在ML中保持隱私的需要在ML中保持隱私的需要源於這樣一個事實,即對大量敏感數據進行分析以預測不同的洞察力。這些數據可能包括個人信息、財務記錄、病歷和其他需要保護的敏感信息。此外,由於缺乏對保密性ML的深入了解,因此軟件工程師,數據處理專家和ML工程師很難在不損害其隱私的情況下分析這些數據。
