
BOOKS - Learning Kernel Classifiers: Theory and Algorithms (Adaptive Computation and ...

Learning Kernel Classifiers: Theory and Algorithms (Adaptive Computation and Machine Learning)
Author: Ralf Herbrich
Year: December 7, 2001
Format: PDF
File size: PDF 2.7 MB
Language: English
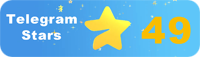
Year: December 7, 2001
Format: PDF
File size: PDF 2.7 MB
Language: English
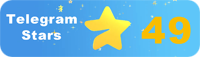
Learning Kernel Classifiers Theory and Algorithms Adaptive Computation and Machine Learning As technology continues to evolve at an unprecedented pace, it is essential for us to understand the process of technological development and its impact on humanity. In this article, we will explore the need and possibility of developing a personal paradigm for perceiving the technological process of modern knowledge and how it can be the basis for the survival of humanity and the unification of people in a warring state. The book "Learning Kernel Classifiers: Theory and Algorithms" provides a comprehensive overview of kernel classification methods, which have emerged as a major topic within the field of machine learning. The kernel technique extends the linear classifier, a well-established and widely studied model, to a wide range of nonlinear pattern recognition tasks such as natural language processing, machine vision, and biological sequence analysis. This book offers a detailed introduction to learning theory, including VC and PAC Bayesian theory, data-dependent structural risk minimization, and compression bounds. It highlights the interaction between theory and algorithms, explaining how learning algorithms work and why they are essential for modern knowledge. The book covers various algorithmic advances, including kernel perceptron learning, kernel Fisher discriminants, support vector machines, relevance vector machines, Gaussian processes, and Bayes point machines. Each chapter includes examples, complete pseudo code of the algorithms presented, and an extensive source code library in MATLAB that allows readers to experiment with the techniques described in the book.
arning Kernel Classifiers Theory and Algorithms Adaptive Computation and Machine arning Поскольку технология продолжает развиваться беспрецедентными темпами, для нас важно понимать процесс технологического развития и его влияние на человечество. В этой статье мы исследуем необходимость и возможность выработки личностной парадигмы восприятия технологического процесса современного знания и того, как оно может быть основой выживания человечества и объединения людей в воюющем государстве. В книге «arning Kernel Classifiers: Theory and Algorithms» представлен всесторонний обзор методов классификации ядра, которые стали основной темой в области машинного обучения. Метод ядра распространяет линейный классификатор, устоявшуюся и широко изученную модель, на широкий спектр задач нелинейного распознавания образов, таких как обработка естественного языка, машинное зрение и анализ биологической последовательности. Эта книга предлагает подробное введение в теорию обучения, включая байесовскую теорию VC и PAC, минимизацию структурных рисков, зависящих от данных, и границы сжатия. Он выделяет взаимодействие между теорией и алгоритмами, объясняя, как работают алгоритмы обучения и почему они необходимы для современных знаний. Книга охватывает различные алгоритмические достижения, включая перцептронное обучение ядра, дискриминанты Фишера ядра, векторные машины поддержки, векторные машины релевантности, гауссовы процессы и точечные машины Байеса. Каждая глава включает примеры, полный псевдокод представленных алгоритмов и обширную библиотеку исходного кода в MATLAB, которая позволяет читателям экспериментировать с техниками, описанными в книге.
Apprentissage Kernel Classifiers Theory and Algorithms Adaptive Computation and Machine arning Alors que la technologie continue d'évoluer à un rythme sans précédent, il est important pour nous de comprendre le processus de développement technologique et son impact sur l'humanité. Dans cet article, nous explorons la nécessité et la possibilité d'élaborer un paradigme personnel de la perception du processus technologique de la connaissance moderne et comment elle peut être la base de la survie de l'humanité et de l'unification des gens dans un État en guerre. livre « arning Kernel Classifiers : Theory and Algorithms » donne un aperçu complet des méthodes de classification du noyau, qui sont devenues un thème majeur dans le domaine de l'apprentissage automatique. La méthode du noyau étend le classificateur linéaire, un modèle établi et largement étudié, à un large éventail de tâches de reconnaissance d'images non linéaires, telles que le traitement du langage naturel, la vision automatique et l'analyse de la séquence biologique. Ce livre propose une introduction détaillée à la théorie de l'apprentissage, y compris la théorie bayésienne du VC et du PAC, la minimisation des risques structurels dépendants des données et les limites de compression. Il met en évidence l'interaction entre la théorie et les algorithmes, expliquant comment fonctionnent les algorithmes d'apprentissage et pourquoi ils sont nécessaires aux connaissances modernes. livre couvre diverses réalisations algorithmiques, y compris l'apprentissage perceptronique du noyau, les discriminants de Fisher du noyau, les machines vectorielles de support, les machines vectorielles de pertinence, les processus gaussiens et les machines à points de Bayes. Chaque chapitre contient des exemples, un pseudo-code complet des algorithmes présentés et une vaste bibliothèque de code source dans MATLAB qui permet aux lecteurs d'expérimenter les techniques décrites dans le livre.
arning Kernel Classifiers Theory and Algorithms Adaptive Computation and Machine arning A medida que la tecnología continúa evolucionando a un ritmo sin precedentes, es importante que comprendamos el proceso de desarrollo tecnológico y su impacto en la humanidad. En este artículo exploramos la necesidad y la posibilidad de generar un paradigma personal para percibir el proceso tecnológico del conocimiento moderno y cómo puede ser la base de la supervivencia de la humanidad y la unión de los seres humanos en un Estado en guerra. libro arning Kernel Classifiers: Theory and Algorithms ofrece una visión general completa de los métodos de clasificación del núcleo, que se han convertido en el tema principal en el campo del aprendizaje automático. método kernel extiende el clasificador lineal, un modelo establecido y ampliamente estudiado, a una amplia gama de tareas de reconocimiento de imágenes no lineales, como el procesamiento del lenguaje natural, la visión automática y el análisis de la secuencia biológica. Este libro ofrece una introducción detallada a la teoría del aprendizaje, incluyendo la teoría bayesiana de VC y PAC, minimizando los riesgos estructurales dependientes de los datos y los límites de compresión. Destaca la interacción entre teoría y algoritmos, explicando cómo funcionan los algoritmos de aprendizaje y por qué son necesarios para el conocimiento moderno. libro cubre diversos avances algorítmicos, incluyendo el aprendizaje perceptrónico del núcleo, los discriminadores del núcleo Fisher, las máquinas vectoriales de soporte, las máquinas vectoriales de relevancia, los procesos gaussianos y las máquinas puntuales de Bayes. Cada capítulo incluye ejemplos, un pseudocódigo completo de los algoritmos presentados y una extensa biblioteca de código fuente en MATLAB que permite a los lectores experimentar con las técnicas descritas en el libro.
arning Kernel Classifiers Theory and Algorithms Adaptative Computation and Machine arning Como a tecnologia continua a evoluir a um ritmo sem precedentes, é importante compreender o processo de desenvolvimento tecnológico e o seu impacto na humanidade. Neste artigo, exploramos a necessidade e a possibilidade de estabelecer um paradigma pessoal para a percepção do processo tecnológico do conhecimento moderno e como ele pode ser a base da sobrevivência da humanidade e da união das pessoas num Estado em guerra. O livro «arning Kernel Classifiers: Theory and Algorithms» apresenta uma revisão completa dos métodos de classificação de núcleo que se tornaram o tema principal do aprendizado de máquinas. O método de núcleo estende o classificador linear, um modelo estabelecido e amplamente estudado, para uma ampla gama de tarefas de reconhecimento de imagem não linear, como processamento de linguagem natural, visão de máquina e análise de seqüência biológica. Este livro oferece uma introdução detalhada à teoria da aprendizagem, incluindo a teoria Baiesa VC e PAC, minimizando os riscos estruturais que dependem dos dados e os limites de compressão. Ele destaca a interação entre a teoria e os algoritmos, explicando como funcionam os algoritmos de aprendizagem e por que eles são essenciais para o conhecimento moderno. O livro abrange vários avanços algoritmicos, incluindo o treinamento de percurso do núcleo, os discriminadores do núcleo Fischer, as máquinas de suporte vetoriais, as máquinas vetoriais de relevância, os processos gaúchos e as máquinas pontuais de Bayes. Cada capítulo inclui exemplos, um pseudo-código completo dos algoritmos apresentados e uma vasta biblioteca de código fonte no MATLAB, que permite aos leitores experimentar técnicas descritas no livro.
arning Kernel Classifiers Theory and Algorithms Adatted Computation and Machine arning Poiché la tecnologia continua a crescere a un ritmo senza precedenti, è importante per noi comprendere il processo di sviluppo tecnologico e l'impatto che ha sull'umanità. In questo articolo stiamo esplorando la necessità e la possibilità di sviluppare un paradigma personale della percezione del processo tecnologico della conoscenza moderna e di come essa possa essere la base della sopravvivenza dell'umanità e dell'unione delle persone in uno stato in guerra. Il libro «arning Kernel Classifiers: Theory and Algorithms» fornisce una panoramica completa dei metodi di classificazione del nucleo che sono diventati il tema principale dell'apprendimento automatico. Il metodo del nucleo estende il classificatore lineare, un modello definito e molto studiato, ad una vasta gamma di attività di riconoscimento non lineare delle immagini, come l'elaborazione del linguaggio naturale, la visione automatica e l'analisi della sequenza biologica. Questo libro offre un'introduzione dettagliata alla teoria dell'apprendimento, tra cui la teoria bayesiana VC e PAC, la riduzione dei rischi strutturali che dipendono dai dati e i limiti di compressione. Evidenzia l'interazione tra teoria e algoritmi, spiegando come funzionano gli algoritmi di apprendimento e perché sono necessari per le conoscenze moderne. Il libro comprende diversi progressi algoritmici, tra cui l'apprendimento percettonico del nucleo, i discriminatori di Fisher kernel, vettoriali macchine di supporto, vettoriali di rilevanza, processi gaussiani e macchinari a punti di Bayes. Ogni capitolo include esempi, uno pseudo-codice completo degli algoritmi presentati e una vasta libreria di codice sorgente in MATLAB, che consente ai lettori di sperimentare le tecniche descritte nel libro.
arning Kernel Classifiers Theorie und Algorithmen Adaptive Datenverarbeitung und maschinelles rnen Da sich die Technologie in einem beispiellosen Tempo weiterentwickelt, ist es für uns wichtig, den technologischen Entwicklungsprozess und seine Auswirkungen auf die Menschheit zu verstehen. In diesem Artikel untersuchen wir die Notwendigkeit und die Möglichkeit, ein persönliches Paradigma für die Wahrnehmung des technologischen Prozesses des modernen Wissens zu entwickeln und wie es die Grundlage für das Überleben der Menschheit und die Vereinigung der Menschen in einem kriegführenden Staat sein kann. Das Buch „arning Kernel Classifiers: Theory and Algorithms“ bietet einen umfassenden Überblick über Kernel-Klassifikationstechniken, die zu einem Hauptthema im Bereich des maschinellen rnens geworden sind. Die Kernel-Methode erweitert den linearen Klassifikator, ein etabliertes und weit verstandenes Modell, auf eine breite Palette von nichtlinearen Mustererkennungsaufgaben wie natürliche Sprachverarbeitung, maschinelles Sehen und biologische Sequenzanalyse. Dieses Buch bietet eine detaillierte Einführung in die rntheorie, einschließlich Bayes'Theorie von VC und PAC, Minimierung datenabhängiger struktureller Risiken und Kompressionsgrenzen. Er hebt die Wechselwirkung zwischen Theorie und Algorithmen hervor und erklärt, wie rnalgorithmen funktionieren und warum sie für modernes Wissen unerlässlich sind. Das Buch deckt eine Vielzahl von algorithmischen Fortschritten ab, darunter Perceptron Core Training, Fisher Core Diskriminants, Vector Support Machines, Vector Relevance Machines, Gauß'sche Prozesse und Bayes'sche Punktmaschinen. Jedes Kapitel enthält Beispiele, einen vollständigen Pseudocode der vorgestellten Algorithmen und eine umfangreiche Quellcodebibliothek in MATLAB, die es den sern ermöglicht, mit den im Buch beschriebenen Techniken zu experimentieren.
Nauka Klasyfikatorów jądra Teoria i algorytmy Adaptacyjne obliczenia i uczenie maszynowe Ponieważ technologia nadal ewoluuje w bezprecedensowym tempie, ważne jest dla nas zrozumienie procesu rozwoju technologicznego i jego wpływu na ludzkość. W tym artykule badamy potrzebę i możliwość rozwijania osobistego paradygmatu postrzegania technologicznego procesu współczesnej wiedzy i tego, jak może ona być podstawą do przetrwania ludzkości i zjednoczenia ludzi w stanie wojennym. Książka „Nauka klasyfikatorów jądra: teoria i algorytmy” zawiera kompleksowy przegląd metod klasyfikacji jądra, które stały się głównym tematem w dziedzinie uczenia maszynowego. Metoda jądra rozszerza klasyfikator liniowy, ugruntowany i szeroko badany model, do szerokiej gamy nieliniowych zadań rozpoznawania wzorców, takich jak przetwarzanie języka naturalnego, widzenie maszynowe i analiza sekwencji biologicznej. Ta książka oferuje szczegółowe wprowadzenie do teorii nauki, w tym teorii Bayesian VC i PAC, minimalizując ryzyko strukturalne zależne od danych i granice kompresji. Podkreśla interakcje między teorią a algorytmami, wyjaśniając, jak działają algorytmy uczenia się i dlaczego są one niezbędne dla obecnej wiedzy. Książka obejmuje różne postępy algorytmiczne, w tym naukę jądra percepcyjnego, dyskryminujące jądra Fishera, maszyny wspomagające wektor, maszyny mające znaczenie dla wektorów, procesy Gaussa i maszyny punktowe Bayesa. Każdy rozdział zawiera przykłady, kompletny pseudokod złożonych algorytmów oraz obszerną bibliotekę kodów źródłowych w MATLAB, która pozwala czytelnikom eksperymentować z technikami opisanymi w książce.
למידת תורת קלסיסי קרנל ואלגוריתמים חישוב אדפטיבי וטכנולוגיה למידת מכונה ממשיך להתפתח בקצב חסר תקדים, חשוב לנו להבין את תהליך ההתפתחות הטכנולוגית ואת השפעתה על האנושות. במאמר זה, אנו בוחנים את הצורך והאפשרות לפתח פרדיגמה אישית לתפיסה של התהליך הטכנולוגי של הידע המודרני וכיצד הוא יכול להיות הבסיס להישרדות האנושות ולאיחוד של אנשים במצב לוחמני. הספר arnel Kernel Classifiers: Theory and Algorithms מספק סקירה מקיפה של שיטות סיווג גרעין, שהפכו לנושא מרכזי בתחום למידת מכונה. שיטת הליבה מרחיבה את הסיווג הליניארי, מודל מבוסס וחקר נרחב, למגוון רחב של משימות זיהוי תבניות לא לינאריות כגון עיבוד שפה טבעית, ראיית מכונה וניתוח רצף ביולוגי. ספר זה מציע הקדמה מפורטת לתורת הלמידה, כולל תאוריית ה-VC של בייסיאן ותורת ה-PAC, מזעור סיכונים מבניים תלויי נתונים, וגבולות דחיסה. הוא מדגיש את יחסי הגומלין בין תיאוריה ואלגוריתמים, ומסביר כיצד אלגוריתמים לומדים עובדים ומדוע הם חיוניים לידע הנוכחי. הספר עוסק בהתקדמויות אלגוריתמיות שונות, כולל למידת גרעין תפיסתי, מפלות גרעין פישר, מכונות תמיכה וקטוריות, מכונות וקטור רלוונטיות, תהליכים גאוסיאנים, ומכונות Bayes point. כל פרק כולל דוגמאות, פסאודו-קוד מלא של אלגוריתמים מוגשים וספריית קוד מקור נרחבת ב-MATLAB המאפשרת לקוראים להתנסות בטכניקות המתוארות בספר.''
Öğrenme Çekirdeği Sınıflandırıcıları Teori ve Algoritmalar Uyarlanabilir Hesaplama ve Makine Öğrenimi Teknoloji benzeri görülmemiş bir hızda gelişmeye devam ederken, teknolojik gelişme sürecini ve insanlık üzerindeki etkisini anlamamız önemlidir. Bu makalede, modern bilginin teknolojik sürecinin algılanması için kişisel bir paradigma geliştirmenin gerekliliğini ve olasılığını ve bunun insanlığın hayatta kalması ve insanların savaşan bir durumda birleşmesi için nasıl bir temel olabileceğini araştırıyoruz. "Öğrenme Çekirdeği Sınıflandırıcıları: Teori ve Algoritmalar" kitabı, makine öğrenimi alanında önemli bir konu haline gelen çekirdek sınıflandırma yöntemlerine kapsamlı bir genel bakış sunmaktadır. Çekirdek yöntemi, yerleşik ve yaygın olarak çalışılan bir model olan doğrusal sınıflandırıcıyı, doğal dil işleme, makine görüşü ve biyolojik dizi analizi gibi çok çeşitli doğrusal olmayan desen tanıma görevlerine genişletir. Bu kitap, Bayesian VC ve PAC teorisi de dahil olmak üzere öğrenme teorisine ayrıntılı bir giriş sunar, veriye bağlı yapısal riskleri ve sıkıştırma sınırlarını en aza indirir. Teori ve algoritmalar arasındaki etkileşimi vurgular, öğrenme algoritmalarının nasıl çalıştığını ve neden mevcut bilgi için gerekli olduklarını açıklar. Kitap, perceptronic kernel learning, Fisher kernel discriminants, vektör destek makineleri, vektör alaka düzeyi makineleri, Gauss süreçleri ve Bayes nokta makineleri dahil olmak üzere çeşitli algoritmik gelişmeleri kapsamaktadır. Her bölüm örnekler, gönderilen algoritmaların tam sahte kodu ve MATLAB'da okuyucuların kitapta açıklanan teknikleri denemelerine olanak tanıyan kapsamlı bir kaynak kod kütüphanesi içerir.
تعلم نظرية مصنفات النواة والخوارزميات الحساب التكيفي والتعلم الآلي مع استمرار تطور التكنولوجيا بوتيرة غير مسبوقة، من المهم بالنسبة لنا أن نفهم عملية التطور التكنولوجي وتأثيرها على البشرية. في هذه المقالة، نستكشف الحاجة وإمكانية تطوير نموذج شخصي لتصور العملية التكنولوجية للمعرفة الحديثة وكيف يمكن أن تكون الأساس لبقاء البشرية وتوحيد الناس في دولة متحاربة. يقدم كتاب "arning Kernel Classifiers: Theory and Algorithms'نظرة عامة شاملة على طرق تصنيف النواة، والتي أصبحت موضوعًا رئيسيًا في مجال التعلم الآلي. توسع طريقة النواة المصنف الخطي، وهو نموذج راسخ ومدرس على نطاق واسع، إلى مجموعة واسعة من مهام التعرف على الأنماط غير الخطية مثل معالجة اللغة الطبيعية ورؤية الآلة وتحليل التسلسل البيولوجي. يقدم هذا الكتاب مقدمة مفصلة لنظرية التعلم، بما في ذلك نظرية Bayesian VC و PAC، وتقليل المخاطر الهيكلية المعتمدة على البيانات، وحدود الضغط. يسلط الضوء على التفاعل بين النظرية والخوارزميات، موضحًا كيف تعمل خوارزميات التعلم ولماذا هي ضرورية للمعرفة الحالية. يغطي الكتاب العديد من التطورات الخوارزمية، بما في ذلك تعلم النواة الإدراكية، وتمييزات نواة فيشر، وآلات دعم الناقلات، وآلات ارتباط المتجهات، والعمليات الجاوسية، وآلات نقطة بايز. يتضمن كل فصل أمثلة، ورمز زائف كامل للخوارزميات المقدمة، ومكتبة شفرة مصدرية واسعة النطاق في MATLAB تسمح للقراء بتجربة التقنيات الموصوفة في الكتاب.
학습 커널 분류 이론 및 알고리즘 적응 형 계산 및 기계 학습은 기술이 전례없는 속도로 계속 발전함에 따라 기술 개발 과정과 인류에 미치는 영향을 이해하는 것이 중요합니다. 이 기사에서 우리는 현대 지식의 기술 과정에 대한 인식과 그것이 인류의 생존과 전쟁 상태에있는 사람들의 통일의 기초가 될 수있는 방법에 대한 개인적인 패러다임을 개발할 필요성과 가능성을 탐구합니다. "arning Kernel Classifiers: Theory and Algorithms" 책은 머신 러닝 분야의 주요 주제가 된 커널 분류 방법에 대한 포괄적 인 개요를 제공합니다. 커널 방법은 확립되고 널리 연구 된 모델 인 선형 분류기를 자연어 처리, 기계 비전 및 생물학적 서열 분석과 같은 광범위한 비선형 패턴 인식 작업으로 확장합니다. 이 책은 베이지안 VC 및 PAC 이론, 데이터 의존적 구조적 위험 최소화 및 압축 범위를 포함한 학습 이론에 대한 자세한 소개를 제공합니다. 그는 이론과 알고리즘의 상호 작용을 강조하여 학습 알고리즘의 작동 방식과 현재 지식에 필수적인 이유를 설명합니다. 이 책은 퍼셉 트로닉 커널 학습, 피셔 커널 판별 장치, 벡터 지원 기계, 벡터 관련성 기계, 가우시안 프로세스 및 베이 즈 포인트 기계를 포함한 다양한 알고리즘 발전을 다룹니다. 각 장에는 예제, 제출 된 알고리즘의 완전한 의사 코드 및 MATLAB의 광범위한 소스 코드 라이브러리가 포함되어있어 독자가 책에 설명 된 기술을 실험 할 수 있습니다.
arning Kernel Classifiers理論とアルゴリズム適応計算と機械学習テクノロジーがかつてないペースで進化し続ける中で、技術開発のプロセスとその人類への影響を理解することは重要です。この記事では、現代の知識の技術的プロセスの認識のための個人的なパラダイムを開発する必要性と可能性を探り、それがどのように人類の生存と戦争状態での人々の統一の基礎となることができます。「arning Kernel Classifiers: Theory and Algorithms」は、機械学習の分野で主要なトピックとなっているカーネル分類方法の包括的な概要を提供しています。カーネル法は、確立され、広く研究されているモデルである線形分類器を、自然言語処理、マシンビジョン、生物学的シーケンス解析などの幅広い非線形パターン認識タスクに拡張する。本書では、ベイジアンVC理論やPAC理論を含む学習理論の詳細な紹介、データ依存構造リスクの最小化、圧縮限界を紹介します。彼は理論とアルゴリズムの相互作用を強調し、学習アルゴリズムがどのように機能するか、そしてそれらが現在の知識に不可欠である理由を説明している。この本は、知覚カーネル学習、フィッシャーカーネル判別、ベクトルサポートマシン、ベクトル関連マシン、ガウス過程、ベイズポイントマシンなど、さまざまなアルゴリズムの進歩をカバーしています。各章には、例、提出されたアルゴリズムの完全な擬似コード、および読者が本に記載されている技術を試すことができるMATLABの広範なソースコードライブラリが含まれています。
學習內核分類理論和算法自適應計算和機器學習隨著技術繼續以前所未有的速度發展,了解技術發展過程及其對人類的影響對我們來說很重要。本文探討了現代知識技術過程感知的必要性和可行性,以及它如何成為人類生存和人類在交戰國團結的基礎。「學習內核分類:理論和算法」一書全面概述了核心分類方法,這些方法已成為機器學習領域的主要主題。內核方法將線性分類器(已建立和廣泛研究的模型)擴展到廣泛的非線性模式識別任務,例如自然語言處理,機器視覺和生物序列分析。本書詳細介紹了學習理論,包括貝葉斯的VC和PAC理論,最大限度地減少了數據依賴性結構風險和壓縮邊界。他強調了理論與算法之間的相互作用,解釋了學習算法的工作原理以及為什麼它們對於現代知識至關重要。該書涵蓋了各種算法進步,包括感知器內核學習,內核費舍爾判別器,支持矢量機,相關性矢量機,高斯過程和點貝葉斯機。每個章節包括示例,完整的演示算法偽代碼以及MATLAB中廣泛的源代碼庫,使讀者可以嘗試書中描述的技術。
