
BOOKS - Why Data Science Projects Fail The Harsh Realities of Implementing AI and Ana...

Why Data Science Projects Fail The Harsh Realities of Implementing AI and Analytics, without the Hype
Author: Douglas Gray, Evan Shellshear
Year: 2025
Pages: 223
Format: PDF | EPUB
File size: 10.1 MB
Language: ENG
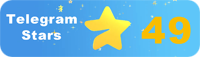
Year: 2025
Pages: 223
Format: PDF | EPUB
File size: 10.1 MB
Language: ENG
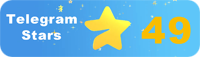
Why Data Science Projects Fail: The Harsh Realities of Implementing AI and Analytics without the Hype As the field of Artificial Intelligence (AI), Data Science, and analytics continues to evolve, it is crippling itself with exaggerated promises of unrealistic technologies, simplifications of complex projects, and marketing hype. This has led to an erosion of trust in one of our most critical approaches to making decisions - data-driven decision making. In their book, "Why Data Science Projects Fail two experts in the field aim to counter the AI hype with a dose of realism, highlighting the harsh realities of implementing AI and analytics without the hype. The authors firmly believe in the power of mathematics, computing, and analytics, but they also recognize that false expectations set by practitioners and leaders can lead to a stunning 80% or more of analytics projects failing, costing enterprises and society hundreds of billions of dollars and leading to nonexperts abandoning one of the most important datadriven decision-making capabilities altogether. For the first time, business leaders, practitioners, students, and interested laypeople will learn what really makes a Data Science project successful.
Почему проекты Data Science терпят неудачу: суровые реалии внедрения ИИ и аналитики без ажиотажа Поскольку сфера искусственного интеллекта (ИИ), Data Science и аналитики продолжает развиваться, она калечит себя преувеличенными обещаниями нереалистичных технологий, упрощениями сложных проектов и маркетинговой шумихой. Это привело к ослаблению доверия к одному из наших наиболее важных подходов к принятию решений - принятию решений на основе данных. В своей книге «Why Data Science Projects Fail» два эксперта в этой области стремятся противостоять ажиотажу на ИИ с помощью дозы реализма, подчеркивая суровые реалии внедрения ИИ и аналитики без ажиотажа. Авторы твердо верят в силу математики, вычислений и аналитики, но они также признают, что ложные ожидания, установленные практиками и лидерами, могут привести к тому, что ошеломляющие 80% или более аналитических проектов потерпят неудачу, что обойдется предприятиям и обществу в сотни миллиардов долларов и приведет к тому, что неэксперты полностью откажутся от одной из самых важных возможностей принятия решений на основе данных. Впервые бизнес-лидеры, практики, студенты и заинтересованные непрофессионалы узнают, что действительно делает проект Data Science успешным.
Pourquoi les projets Data Science échouent : les dures réalités de la mise en œuvre de l'IA et de l'analyse sans excitation Alors que le domaine de l'intelligence artificielle (IA), de la Data Science et de l'analyse continue d'évoluer, il se ternit de promesses exagérées de technologies irréalistes, de simplifications de projets complexes et de bruits marketing. Cela a affaibli la crédibilité de l'une de nos approches les plus importantes en matière de prise de décisions, à savoir la prise de décisions fondées sur les données. Dans leur livre Why Data Science Projects Fail, deux experts du domaine cherchent à contrer l'excitation sur l'IA par une dose de réalisme, en soulignant les dures réalités de l'introduction de l'IA et de l'analyse sans excitation. s auteurs croient fermement au pouvoir des mathématiques, de l'informatique et de l'analyse, mais ils reconnaissent également, que les fausses attentes établies par les praticiens et les dirigeants peuvent conduire à cela, que 80 % ou plus des projets d'analyse échoueront, ce qui coûtera des centaines de milliards de dollars aux entreprises et à la société, que les non-experts abandonneront complètement l'une des plus importantes possibilités de prise de décisions fondées sur les données. Pour la première fois, les chefs d'entreprise, les praticiens, les étudiants et les non-professionnels intéressés apprennent ce qui fait vraiment le succès du projet Data Science.
Por qué fracasan los proyectos de Data Science: las duras realidades de la implementación de la IA y los analistas sin emoción A medida que el campo de la inteligencia artificial (IA), Data Science y los analistas siguen evolucionando, se mutila con promesas exageradas de tecnologías poco realistas, simplificaciones de proyectos complejos y marketing Es una mierda. Esto ha debilitado la confianza en uno de nuestros enfoques más importantes para la toma de decisiones: la toma de decisiones basada en datos. En su libro «Why Data Science Projects Fail», dos expertos en este campo buscan contrarrestar la emoción sobre la IA con una dosis de realismo, destacando las duras realidades de la implementación de la IA y los analistas sin revuelo. autores creen firmemente en el poder de las matemáticas, la computación y la analítica, pero también reconocen, que las falsas expectativas establecidas por los profesionales y líderes pueden llevar a que un asombroso 80% o más de los proyectos analíticos fracasarían, lo que costaría cientos de miles de millones de dólares a las empresas y a la sociedad y llevaría a que los no expertos abandonarían por completo una de las oportunidades más importantes para tomar decisiones basadas en datos. Por primera vez, líderes empresariales, profesionales, estudiantes y no profesionales interesados aprenderán lo que realmente hace que el proyecto Data Science sea un éxito.
Perché i progetti di Data Science falliscono: le dure realtà di implementazione dell'IA e gli analisti non esaltati Poiché l'intelligenza artificiale (IA), Data Science e gli analisti continuano a svilupparsi, si sta corrompendo con le promesse esagerate di tecnologie irrealistiche, le semplificazioni di progetti complessi e il rumore del marketing. Ciò ha ridotto la fiducia in uno dei nostri approcci decisionali più importanti, quello di prendere decisioni basate sui dati. In Why Data Science Progetti Fail, due esperti in questo campo cercano di contrastare l'eccitazione dell'intelligenza artificiale con una dose di realismo, sottolineando la dura realtà dell'implementazione dell'IA e degli analisti senza esasperazione. Gli autori credono fermamente nel potere della matematica, del calcolo e degli analisti, ma riconoscono anche. che le false aspettative stabilite da pratici e leader possono portare a: che l'80% o più dei progetti analitici falliranno, costando centinaia di miliardi di dollari alle imprese e alla società e portando a: che i non esperti abbandoneranno completamente una delle più importanti opportunità decisionali basate sui dati. Per la prima volta, i leader aziendali, i professionisti, gli studenti e i non professionisti interessati scopriranno cosa rende il progetto Data Science un vero successo.
Warum Data-Science-Projekte scheitern: Die harten Realitäten von KI und Analytics ohne Hype Da sich der Bereich der Künstlichen Intelligenz (KI), Data Science und Analytics immer weiter entwickelt, lähmt er sich mit übertriebenen Versprechungen unrealistischer Technologien, Vereinfachungen komplexer Projekte und Marketing-Hype. Dies hat zu einem schwindenden Vertrauen in einen unserer wichtigsten Entscheidungsansätze geführt - die datengesteuerte Entscheidungsfindung. In ihrem Buch „Why Data Science Projects Fail“ versuchen zwei Experten auf diesem Gebiet, dem KI-Hype mit einer Dosis Realismus entgegenzuwirken, indem sie die harten Realitäten der Einführung von KI und Analytics ohne Hype hervorheben. Die Autoren glauben fest an die Macht der Mathematik, Berechnung und Analyse, aber sie erkennen auch, dass falsche Erwartungen, die von Praktizierenden und Führern aufgestellt werden, dazu führen können, dass erstaunliche 80% oder mehr der Analyseprojekte scheitern werden, was Unternehmen und die Gesellschaft Hunderte von Milliarden Dollar kosten und dazu führen wird, dass Nicht-Experten auf eine der wichtigsten datengestützten Entscheidungsmöglichkeiten komplett verzichten. Zum ersten Mal erfahren Führungskräfte, Praktiker, Studenten und interessierte Laien, was ein Data Science-Projekt wirklich erfolgreich macht.
Dlaczego projekty Data Science nie powiodły się: trudne realia AI i analityki bez emocji Jako dziedzina sztucznej inteligencji (AI), Data Science i analytics nadal ewoluuje, kalekuje się z przesadnych obietnic nierealistycznych technologii, uproszczenia złożonych projektów i marketingu hype Doprowadziło to do osłabienia zaufania do jednego z naszych najważniejszych podejść decyzyjnych - procesu decyzyjnego opartego na danych. W swojej książce „Why Data Science Projects Fail”, dwaj eksperci w tej dziedzinie mają na celu przeciwdziałanie hipe AI z dawką realizmu, podkreślając surowe realia adopcji AI i analityki bez hype. Autorzy mocno wierzą w moc matematyki, obliczeń i analityki, przyznają jednak, że fałszywe oczekiwania praktyków i liderów mogą prowadzić do niepowodzenia oszałamiającego 80% lub więcej projektów analitycznych, kosztowanie przedsiębiorstw i społeczeństwa setkami miliardów dolarów, co doprowadziłoby do tego, że osoby niebędące ekspertami całkowicie porzuciłyby jedną z najważniejszych możliwości podejmowania decyzji opartych na danych. Po raz pierwszy liderzy biznesu, praktycy, studenci i zainteresowani świeccy dowiedzą się, co naprawdę czyni projekt Data Science sukcesem.
''
Veri Bilimi projeleri neden başarısız oluyor: Yapay zeka ve analitiğin heyecansız sert gerçekleri Yapay zeka (AI), Veri Bilimi ve analitik alanı gelişmeye devam ederken, gerçekçi olmayan teknolojilerin abartılı vaatleri, karmaşık projelerin basitleştirilmesi ve pazarlama aldatmacasıyla kendini sakatlıyor. Bu, en önemli karar verme yaklaşımlarımızdan biri olan veriye dayalı karar verme sürecine olan güvenin zayıflamasına yol açmıştır. "Why Data Science Projects Fail" (Veri Bilimi Projeleri Neden Başarısız Olur) adlı kitaplarında, alandaki iki uzman, AI hype'a karşı bir miktar gerçekçilik ile karşı koymayı, AI'nın benimsenmesinin sert gerçeklerini ve hype olmadan analitiği vurgulamayı amaçlıyor. Yazarlar matematik, hesaplama ve analitiğin gücüne kuvvetle inanırlar. Ancak, uygulayıcılar ve liderler tarafından belirlenen yanlış beklentilerin, analitik projelerin %80 veya daha fazlasının şaşırtıcı bir şekilde başarısız olmasına yol açabileceğini de kabul ediyorlar. İşletmelere ve topluma yüz milyarlarca dolara mal olan ve uzman olmayanlara yol açan, en önemli veri odaklı karar verme fırsatlarından birini tamamen terk edecektir. İlk kez, iş liderleri, uygulayıcılar, öğrenciler ve ilgilenen meslekten olmayan insanlar, Veri Bilimi projesini gerçekten başarılı kılan şeyleri öğrenecekler.
لماذا تفشل مشاريع علوم البيانات: الحقائق القاسية للذكاء الاصطناعي والتحليلات دون إثارة مع استمرار تطور مجال الذكاء الاصطناعي (AI) وعلوم البيانات والتحليلات، فإنه يشل نفسه بوعود مبالغ فيها من التقنيات غير الواقعية وتبسيط المشاريع المعقدة وضجيج التسويق. وقد أدى ذلك إلى إضعاف الثقة في أحد أهم مناهجنا لصنع القرار - صنع القرار القائم على البيانات. في كتابهما «لماذا تفشل مشاريع علوم البيانات»، يهدف خبيران في هذا المجال إلى مواجهة ضجيج الذكاء الاصطناعي بجرعة من الواقعية، مما يسلط الضوء على الحقائق القاسية لتبني الذكاء الاصطناعي والتحليلات دون ضجيج. المؤلفون يؤمنون بقوة بقوة الرياضيات والحوسبة والتحليلات لكنهم يقرون أيضًا بأن التوقعات الخاطئة التي وضعها الممارسون والقادة يمكن أن تؤدي إلى فشل 80٪ أو أكثر من المشاريع التحليلية، تكلف الشركات والمجتمع مئات المليارات من الدولارات وتؤدي إلى أن يتخلى غير الخبراء تمامًا عن واحدة من أهم فرص صنع القرار القائمة على البيانات. لأول مرة، سيتعلم قادة الأعمال والممارسون والطلاب والأشخاص العاديون المهتمون ما الذي يجعل مشروع علوم البيانات ناجحًا حقًا.
為什麼數據科學項目失敗:引入AI和分析而沒有炒作的嚴峻現實隨著人工智能(AI),數據科學和分析領域的不斷發展,它通過誇大不現實技術的承諾,復雜項目的簡化以及營銷炒作而削弱了自己。這削弱了人們對我們最重要的決策辦法之一----數據決策的信心。在他們的著作《為什麼數據科學項目失敗》(Why Data Science Projects Fail)中,該領域的兩位專家試圖通過一劑現實主義來抵制AI的炒作,強調引入AI和分析師的嚴峻現實,而沒有炒作。作者堅信數學,計算和分析的力量,但他們也承認, 從業者和領導者提出的錯誤期望可能會導致、 超過80%的分析項目將失敗,使企業和社會損失數千億美元,並導致、 非專家將完全放棄最重要的數據驅動決策機會之一。商業領袖、從業人員、學生和感興趣的非專業人士首次了解了真正使Data Science項目取得成功的原因。
