
BOOKS - PROGRAMMING - Probability for Machine Learning Discover How To Harness Uncert...

Probability for Machine Learning Discover How To Harness Uncertainty With Python
Author: Джейсон Браунли
Year: 2020
Pages: 319
Format: PDF
File size: 10 MB
Language: ENG
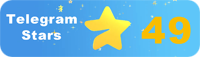
Year: 2020
Pages: 319
Format: PDF
File size: 10 MB
Language: ENG
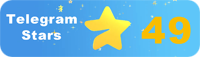
As the world changes at an everincreasing pace we need to stay ahead of the curve and continuously adapt our thinking technologies and tools to keep up with the rate of innovation This book takes you on a journey of discovery to help you stay relevant in the field of machine learningProbability for Machine Learning is a mustread for anyone looking to build a foundation in this critical area. Probability for Machine Learning: Harnessing Uncertainty with Python In today's fast-paced technological landscape, machine learning has become an integral part of various industries and applications. However, to truly master this field, one needs to understand the fundamental principles of probability. Probability for Machine Learning: Harnessing Uncertainty with Python provides a comprehensive guide to grasping the basics of probability and its application in machine learning. The book offers a detailed explanation of probability concepts, making it accessible and easy to understand for those who may not have a strong background in mathematics. The Need for Probability in Machine Learning Machine learning is built upon the principles of probability, and without a solid understanding of these principles, it becomes challenging to develop effective algorithms and models. Probability is essential for understanding how machines learn from data and make predictions or decisions. It is crucial to grasp the uncertainty aspects of machine learning, as it helps to develop robust models that can adapt to changing data distributions and avoid overfitting. By studying probability, one can gain a deeper understanding of the underlying mechanisms of machine learning and develop more accurate predictive models.
Поскольку мир меняется постоянно растущими темпами, нам нужно идти впереди и постоянно адаптировать наши технологии мышления и инструменты, чтобы идти в ногу со скоростью инноваций. Эта книга проведет вас в путешествие открытий, чтобы помочь вам оставаться актуальными в области машинного обучения. Вероятность для машинного обучения - это чистота для всех, кто хочет построить основу в этой критической области. Вероятность для машинного обучения: использование неопределенности с помощью Python В современном быстро развивающемся технологическом ландшафте машинное обучение стало неотъемлемой частью различных отраслей и приложений. Однако, чтобы по-настоящему освоить это поле, нужно понимать фундаментальные принципы вероятности. Probability for Machine arning: Harnessing Uncertainty with Python предоставляет исчерпывающее руководство по усвоению основ вероятности и её применению в машинном обучении. Книга предлагает подробное объяснение вероятностных концепций, делая его доступным и простым для понимания для тех, кто, возможно, не имеет сильных знаний в математике. Потребность в вероятности в машинном обучении Машинное обучение построено на принципах вероятности, и без твердого понимания этих принципов разработка эффективных алгоритмов и моделей становится сложной задачей. Вероятность необходима для понимания того, как машины учатся на данных и принимают прогнозы или решения. Крайне важно понять аспекты неопределенности машинного обучения, поскольку это помогает разрабатывать надежные модели, которые могут адаптироваться к изменяющимся распределениям данных и избегать переобучения. Изучая вероятность, можно получить более глубокое понимание основных механизмов машинного обучения и разработать более точные прогностические модели.
Alors que le monde évolue à un rythme sans cesse croissant, nous devons aller de l'avant et adapter constamment nos technologies de pensée et nos outils pour suivre le rythme de l'innovation. Ce livre vous guidera dans un voyage de découverte pour vous aider à rester pertinent dans le domaine de l'apprentissage automatique. La probabilité pour l'apprentissage automatique est la pureté pour tous ceux qui veulent construire une base dans ce domaine critique. Probabilité pour le Machine arning : exploiter l'incertitude avec Python Dans le paysage technologique en évolution rapide d'aujourd'hui, le Machine arning fait désormais partie intégrante de diverses industries et applications. Cependant, pour vraiment maîtriser ce domaine, vous devez comprendre les principes fondamentaux de probabilité. Probability for Machine arning : Harnessing Uncertainty with Python fournit un guide complet sur la façon d'assimiler les bases de la probabilité et de l'appliquer à l'apprentissage automatique. livre offre une explication détaillée des concepts probabilistes, le rendant accessible et facile à comprendre pour ceux qui n'ont peut-être pas de connaissances solides en mathématiques. besoin de probabilité pour l'apprentissage automatique L'apprentissage automatique est basé sur les principes de probabilité, et sans une bonne compréhension de ces principes, le développement d'algorithmes et de modèles efficaces devient difficile. La probabilité est nécessaire pour comprendre comment les machines apprennent sur les données et prennent des prévisions ou des décisions. Il est essentiel de comprendre les aspects de l'incertitude de l'apprentissage automatique, car cela aide à développer des modèles fiables qui peuvent s'adapter à l'évolution des distributions de données et éviter de se réapprendre. En étudiant la probabilité, il est possible de mieux comprendre les mécanismes sous-jacents de l'apprentissage automatique et de développer des modèles prédictifs plus précis.
A medida que el mundo cambia a un ritmo cada vez mayor, necesitamos ir por delante y adaptar constantemente nuestras tecnologías de pensamiento y herramientas para mantener el ritmo de la innovación. Este libro le guiará en un viaje de descubrimiento para ayudarle a mantenerse actualizado en el campo del aprendizaje automático. La probabilidad de aprendizaje automático es pureza para cualquiera que quiera construir una base en este campo crítico. Posibilidad de aprendizaje automático: aprovechar la incertidumbre con Python En el panorama tecnológico en rápida evolución de hoy, el aprendizaje automático se ha convertido en una parte integral de diferentes industrias y aplicaciones. n embargo, para dominar realmente este campo, es necesario entender los principios fundamentales de la probabilidad. Probability for Machine arning: Harnessing Uncertainty with Python proporciona una guía exhaustiva para asimilar las bases de probabilidad y su aplicación en el aprendizaje automático. libro ofrece una explicación detallada de los conceptos probabilísticos, haciéndolo accesible y fácil de entender para aquellos que pueden no tener un conocimiento fuerte en matemáticas. Necesidad de probabilidad en el aprendizaje automático aprendizaje automático se basa en los principios de probabilidad, y sin una comprensión sólida de estos principios, el desarrollo de algoritmos y modelos eficaces se convierte en una tarea difícil. La probabilidad es necesaria para entender cómo las máquinas aprenden de los datos y toman predicciones o decisiones. Es fundamental comprender los aspectos de la incertidumbre del aprendizaje automático, ya que ayuda a desarrollar modelos robustos que puedan adaptarse a las cambiantes distribuciones de datos y evitar el re-aprendizaje. Al estudiar la probabilidad, es posible obtener una comprensión más profunda de los mecanismos básicos del aprendizaje automático y desarrollar modelos predictivos más precisos.
Como o mundo está mudando a um ritmo constante de crescimento, precisamos ir à frente e adaptar constantemente nossas tecnologias de pensamento e ferramentas para seguir em linha com a velocidade da inovação. Este livro vai levá-lo a uma viagem de descobertas para ajudá-lo a permanecer atualizado no aprendizado de máquinas. A possibilidade de aprender a máquina é limpa para todos os que querem construir uma base nesta área crítica. Probabilidade para o aprendizado de máquina: uso da incerteza com Python No atual panorama tecnológico em rápida evolução, o aprendizado de máquinas tornou-se parte integrante de vários setores e aplicativos. No entanto, é preciso compreender os princípios fundamentais da probabilidade para realmente aprender este campo. Professity for Machine arning: A Harnessing Uncertainty with Python fornece um guia completo para aprender quais são as suas probabilidades e aplicá-las na aprendizagem de máquinas. O livro oferece uma explicação detalhada para os conceitos prováveis, tornando-o acessível e fácil de compreender para aqueles que podem não ter conhecimento forte em matemática. Necessidade de probabilidade de aprendizado de máquina A aprendizagem de máquinas é baseada nos princípios de probabilidade e, sem uma compreensão firme desses princípios, desenvolver algoritmos e modelos eficientes torna-se um desafio. A probabilidade é necessária para entender como as máquinas aprendem com dados e tomam previsões ou decisões. É fundamental compreender os aspectos da incerteza do aprendizado de máquina, pois ajuda a desenvolver modelos confiáveis que possam adaptar-se à variável distribuição de dados e evitar o reaproveitamento. Ao estudar a probabilidade, é possível ter uma compreensão mais profunda dos principais mecanismos de aprendizagem de máquinas e desenvolver modelos mais precisos.
Poiché il mondo sta cambiando a un ritmo sempre crescente, dobbiamo stare davanti e adattare costantemente le nostre tecnologie di pensiero e gli strumenti per stare al passo con la velocità dell'innovazione. Questo libro vi condurrà in un viaggio di scoperte per aiutarvi a rimanere aggiornati nel campo dell'apprendimento automatico. La probabilità di apprendimento automatico è la purezza di tutti coloro che vogliono costruire una base in questo ambito critico. Possibilità di apprendimento automatico: sfruttando l'incertezza con Python Nel panorama tecnologico in continua evoluzione, l'apprendimento automatico è diventato parte integrante di diversi settori e applicazioni. Tuttavia, per imparare davvero questo campo, bisogna comprendere i principi fondamentali della probabilità. Probability for Machine arning: Harnessing Uncertainty with Python fornisce una guida completa per l'apprendimento della probabilità e la sua applicazione nell'apprendimento automatico. Il libro offre una spiegazione dettagliata dei concetti probabilistici, rendendolo disponibile e facile da comprendere per coloro che potrebbero non avere una forte conoscenza in matematica. La necessità di apprendimento automatico L'apprendimento automatico è basato sui principi di probabilità, e senza una chiara comprensione di questi principi, lo sviluppo di algoritmi e modelli efficaci diventa una sfida. La probabilità è necessaria per capire come le macchine imparano dai dati e prendono previsioni o decisioni. È fondamentale comprendere l'incertezza dell'apprendimento automatico, perché aiuta a sviluppare modelli affidabili in grado di adattarsi ai cambiamenti nella distribuzione dei dati ed evitare la riqualificazione. Studiando le probabilità, è possibile comprendere meglio i principali meccanismi di apprendimento automatico e sviluppare modelli predittivi più precisi.
Da sich die Welt in einem ständig wachsenden Tempo verändert, müssen wir vorangehen und unsere Denktechnologien und Werkzeuge ständig anpassen, um mit der Geschwindigkeit der Innovation Schritt zu halten. Dieses Buch wird e auf eine Entdeckungsreise mitnehmen, um Ihnen zu helfen, im Bereich des maschinellen rnens relevant zu bleiben. Die Wahrscheinlichkeit für maschinelles rnen ist die Reinheit für jeden, der eine Basis in diesem kritischen Bereich aufbauen möchte. Wahrscheinlichkeit für maschinelles rnen: Unsicherheit nutzen mit Python In der heutigen schnelllebigen Technologielandschaft ist maschinelles rnen zu einem integralen Bestandteil verschiedener Branchen und Anwendungen geworden. Um dieses Feld wirklich zu beherrschen, muss man jedoch die grundlegenden Prinzipien der Wahrscheinlichkeit verstehen. Probability for Machine arning: Harnessing Uncertainty with Python bietet eine umfassende Anleitung zum Erlernen der Grundlagen der Wahrscheinlichkeit und ihrer Anwendung im maschinellen rnen. Das Buch bietet eine detaillierte Erklärung der probabilistischen Konzepte, so dass es zugänglich und leicht verständlich für diejenigen, die möglicherweise nicht über starke Kenntnisse der Mathematik. Das Bedürfnis nach Wahrscheinlichkeit im maschinellen rnen Maschinelles rnen basiert auf Wahrscheinlichkeitsprinzipien, und ohne ein solides Verständnis dieser Prinzipien wird die Entwicklung effizienter Algorithmen und Modelle zu einer Herausforderung. Die Wahrscheinlichkeit ist notwendig, um zu verstehen, wie Maschinen aus Daten lernen und Vorhersagen oder Entscheidungen treffen. Es ist wichtig, die Unsicherheitsaspekte des maschinellen rnens zu verstehen, da dies dazu beiträgt, robuste Modelle zu entwickeln, die sich an sich ändernde Datenverteilungen anpassen und Umschulungen vermeiden können. Durch das Studium der Wahrscheinlichkeit können e ein tieferes Verständnis der zugrunde liegenden Mechanismen des maschinellen rnens erlangen und genauere Vorhersagemodelle entwickeln.
W miarę jak świat zmienia się w coraz większym tempie, musimy prowadzić drogę i stale dostosowywać nasze technologie i narzędzia myślenia, aby nadążyć za tempem innowacji. Ta książka zabiera Cię w podróż odkrycia, aby pomóc Ci pozostać istotnym w nauce maszyn. Prawdopodobieństwo uczenia maszynowego jest czystość dla każdego, kto chce zbudować fundament w tym krytycznym obszarze. Prawdopodobieństwo uczenia maszynowego: Wykorzystywanie niepewności z Pythonem W dzisiejszym szybko rozwijającym się krajobrazie technologicznym uczenie maszynowe stało się integralną częścią różnych gałęzi przemysłu i zastosowań. Aby jednak naprawdę opanować tę dziedzinę, musisz zrozumieć podstawowe zasady prawdopodobieństwa. Prawdopodobieństwo uczenia maszynowego: Wykorzystywanie niepewności z Pythonem zapewnia kompleksowy przewodnik po nauce podstaw prawdopodobieństwa i stosowaniu go do uczenia maszynowego. Książka zawiera szczegółowe wyjaśnienie koncepcji probabilistycznych, dzięki czemu jest ona dostępna i łatwa do zrozumienia dla tych, którzy mogą nie posiadać silnej wiedzy z matematyki. Potrzeba prawdopodobieństwa w uczeniu maszynowym Uczenie maszynowe opiera się na zasadach prawdopodobieństwa i bez zdecydowanego zrozumienia tych zasad rozwój skutecznych algorytmów i modeli staje się trudnym zadaniem. Prawdopodobieństwo jest niezbędne dla zrozumienia, w jaki sposób maszyny uczą się od danych i podejmują przewidywania lub decyzje. Kluczowe znaczenie ma zrozumienie niepewnych aspektów uczenia maszynowego, ponieważ pomaga to w opracowaniu solidnych modeli, które mogą dostosować się do zmieniających się dystrybucji danych i unikać przekwalifikowania. Badając prawdopodobieństwo, można uzyskać głębsze zrozumienie podstawowych mechanizmów uczenia maszynowego i opracować dokładniejsze modele predykcyjne.
ככל שהעולם משתנה בקצב הולך וגובר, עלינו להוביל את הדרך ולהתאים כל הזמן את טכנולוגיות החשיבה והכלים שלנו הספר הזה לוקח אתכם למסע של תגליות שיעזרו לכם להישאר רלוונטיים בלימוד מכונה. ההסתברות ללמידת מכונה היא טוהר לכל מי שרוצה לבנות בסיס באזור קריטי זה. הסתברות ללימוד מכונה: ניצול אי ודאות עם פייתון בנוף הטכנולוגי המתפתח במהירות כיום, למידת מכונה הפכה לחלק בלתי נפרד מתעשיות ויישומים שונים. עם זאת, כדי באמת לשלוט בתחום זה, אתה צריך להבין את העקרונות הבסיסיים של הסתברות. הסתברות ללמידת מכונה: רתימת אי ודאות עם פייתון מספקת מדריך מקיף ללימוד יסודות ההסתברות ויישומה על למידת מכונה. הספר מציע הסבר מפורט של מושגים הסתברותיים, מה שהופך אותו נגיש וקל להבנה עבור אלה שאולי אין להם ידע רב במתמטיקה. הצורך בהסתברות ללמידת מכונה בנוי על עקרונות ההסתברות, וללא הבנה מוצקה של עקרונות אלה, פיתוח אלגוריתמים ומודלים יעילים הופך למשימה קשה. ההסתברות חיונית להבנת האופן שבו מכונות לומדות מנתונים ומקבלות תחזיות או החלטות. חיוני להבין את היבטי אי הוודאות של למידת מכונה כאשר הדבר עוזר לפתח מודלים חזקים שיכולים להתאים את עצמם לשינוי תפוצת הנתונים ולהימנע מהתאמנות מחדש. על ידי לימוד ההסתברות, ניתן לרכוש הבנה עמוקה יותר של המנגנונים הבסיסיים של למידת מכונה ולפתח מודלים חיזויים מדויקים יותר.''
Dünya giderek artan bir hızla değişirken, inovasyonun hızına ayak uydurmak için yol göstermeli ve düşünce teknolojilerimizi ve araçlarımızı sürekli uyarlamalıyız. Bu kitap sizi makine öğrenimi ile alakalı kalmanıza yardımcı olacak bir keşif yolculuğuna çıkarıyor. Makine öğrenimi olasılığı, bu kritik alanda bir temel oluşturmak isteyen herkes için saflıktır. Makine Öğrenimi için Olasılık: Python ile Belirsizlikten Yararlanma Günümüzün hızla gelişen teknoloji ortamında, makine öğrenimi çeşitli endüstrilerin ve uygulamaların ayrılmaz bir parçası haline gelmiştir. Ancak, bu alanda gerçekten ustalaşmak için, olasılığın temel ilkelerini anlamanız gerekir. Makine Öğrenimi için Olasılık: Python ile Belirsizlikten Yararlanma, olasılığın temellerini öğrenmek ve makine öğrenimine uygulamak için kapsamlı bir rehber sunar. Kitap, olasılıksal kavramların ayrıntılı bir açıklamasını sunarak, güçlü bir matematik bilgisine sahip olmayanlar için erişilebilir ve anlaşılması kolay hale getirir. Makine öğreniminde olasılık ihtiyacı Makine öğrenimi olasılık ilkeleri üzerine kuruludur ve bu ilkelerin kesin bir şekilde anlaşılmadan, etkili algoritmaların ve modellerin geliştirilmesi zor bir görev haline gelir. Olasılık, makinelerin verilerden nasıl öğrendiğini ve tahminler veya kararlar verdiğini anlamak için gereklidir. Makine öğreniminin belirsizlik yönlerini anlamak çok önemlidir, çünkü bu, değişen veri dağılımlarına uyum sağlayabilecek ve yeniden eğitimden kaçınabilecek sağlam modeller geliştirmeye yardımcı olur. Olasılığı inceleyerek, makine öğreniminin altında yatan mekanizmaları daha iyi anlayabilir ve daha doğru tahmin modelleri geliştirebilirsiniz.
مع تغير العالم بمعدل متزايد باستمرار، نحتاج إلى قيادة الطريق وتكييف تقنياتنا وأدواتنا الفكرية باستمرار لمواكبة سرعة الابتكار. يأخذك هذا الكتاب في رحلة اكتشاف لمساعدتك على البقاء على صلة بالتعلم الآلي. احتمالية التعلم الآلي هي النقاء لأي شخص يريد بناء أساس في هذا المجال الحرج. احتمالية التعلم الآلي: استغلال عدم اليقين مع بايثون في المشهد التكنولوجي سريع التطور اليوم، أصبح التعلم الآلي جزءًا لا يتجزأ من الصناعات والتطبيقات المختلفة. ومع ذلك، لإتقان هذا المجال حقًا، عليك أن تفهم المبادئ الأساسية للاحتمال. توفر احتمالية التعلم الآلي: تسخير عدم اليقين مع بايثون دليلاً شاملاً لتعلم أساسيات الاحتمال وتطبيقه على التعلم الآلي. يقدم الكتاب شرحًا مفصلاً للمفاهيم الاحتمالية، مما يجعله متاحًا وسهل الفهم لأولئك الذين قد لا يكون لديهم معرفة قوية بالرياضيات. تستند الحاجة إلى احتمالية التعلم الآلي إلى مبادئ الاحتمال، وبدون فهم راسخ لهذه المبادئ، يصبح تطوير خوارزميات ونماذج فعالة مهمة صعبة. الاحتمال ضروري لفهم كيفية تعلم الآلات من البيانات واتخاذ التنبؤات أو القرارات. من الأهمية بمكان فهم جوانب عدم اليقين في التعلم الآلي لأن هذا يساعد على تطوير نماذج قوية يمكنها التكيف مع توزيعات البيانات المتغيرة وتجنب إعادة التدريب. من خلال دراسة الاحتمالات، يمكن للمرء اكتساب فهم أعمق للآليات الأساسية للتعلم الآلي وتطوير نماذج تنبؤية أكثر دقة.
세계가 계속 증가하는 속도로 변화함에 따라, 우리는 혁신 속도에 보조를 맞추기 위해 사고 기술과 도구를 이끌고 지속적으로 적응해야합니다. 이 책은 머신 러닝과 관련성을 유지하는 데 도움이되는 발견의 여정을 안내합니다. 머신 러닝의 가능성은이 중요한 영역에 기초를 구축하려는 사람에게는 순도입니다. 머신 러닝의 가능성: Python으로 불확실성을 악용 오늘날의 빠르게 진화하는 기술 환경에서 머신 러닝은 다양한 산업 및 응용 분야에서 없어서는 안될 부분이되었습니다. 그러나이 분야를 진정으로 습득하려면 확률의 기본 원칙을 이해해야합니다. 기계 학습 확률: Python으로 불확실성을 해결하면 기본 확률을 배우고 기계 학습에 적용하는 포괄적 인 가이드를 제공합니다. 이 책은 확률 론적 개념에 대한 자세한 설명을 제공하여 수학에 대한 강력한 지식이없는 사람들이 쉽게 이해할 수 있도록합니다. 머신 러닝 머신 러닝의 확률에 대한 필요성은 확률의 원리를 기반으로하며 이러한 원리를 확실하게 이해하지 않으면 효과적인 알고리즘과 모델의 개발이 어려운 작업이됩니다. 기계가 데이터를 배우고 예측이나 결정을 내리는 방법을 이해하려면 확률이 필수적입니다. 변화하는 데이터 분포에 적응하고 재 훈련을 피할 수있는 강력한 모델을 개발하는 데 도움이되므로 머신 러닝의 불확실성 측면을 이해하는 것이 중요합니다. 확률을 연구함으로써 머신 러닝의 기본 메커니즘에 대해 더 깊이 이해하고보다 정확한 예측 모델을 개발할 수 있습니다.
隨著世界以不斷增長的速度變化,我們需要向前邁進,不斷調整我們的思維技術和工具,以跟上創新的步伐。本書將引導您進入發現之旅,以幫助您在機器學習領域保持相關性。對於任何希望在這個關鍵領域建立基礎的人來說,機器學習的概率都是純凈的。機器學習的概率:使用Python利用不確定性在當今快速發展的技術環境中,機器學習已成為各種行業和應用不可或缺的一部分。但是,要真正掌握這一領域,您需要了解概率的基本原理。機器學習的可行性:與Python建立聯系提供了全面的指南,以學習概率基礎及其在機器學習中的應用。這本書提供了概率概念的詳細解釋,使那些可能缺乏數學知識的人易於理解。機器學習的概率需求機器學習建立在概率原理的基礎上,如果沒有對這些原理的牢固理解,開發有效的算法和模型就變得具有挑戰性。需要概率來了解機器如何從數據中學習並做出預測或決策。了解機器學習的不確定性至關重要,因為它有助於開發能夠適應不斷變化的數據分布並避免再學習的可靠模型。通過研究概率,可以更好地了解機器學習的基本機制,並開發出更準確的預測模型。
