
BOOKS - PROGRAMMING - Machine Learning for Email Spam Filtering and Priority Inbox

Machine Learning for Email Spam Filtering and Priority Inbox
Author: Drew Conway, John White
Year: 2011
Pages: 148
Format: PDF
File size: 125,7 MB
Language: ENG
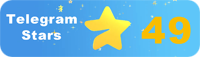
Year: 2011
Pages: 148
Format: PDF
File size: 125,7 MB
Language: ENG
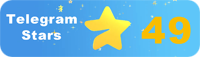
''
