
BOOKS - PROGRAMMING - Regularization in Deep Learning

Regularization in Deep Learning
Author: Peng Liu
Year: 2022 MEAP V3
Pages: 177
Format: PDF
File size: 11,1 MB
Language: ENG
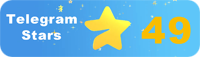
Year: 2022 MEAP V3
Pages: 177
Format: PDF
File size: 11,1 MB
Language: ENG
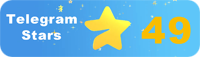
''
