
BOOKS - Shallow Learning vs. Deep Learning A Practical Guide for Machine Learning Sol...

Shallow Learning vs. Deep Learning A Practical Guide for Machine Learning Solutions
Author: Omer Faruk Ertugrul, Josep M Guerrero, Musa Yilmaz
Year: 2024
Pages: 283
Format: PDF | EPUB
File size: 45.5 MB
Language: ENG
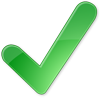
Year: 2024
Pages: 283
Format: PDF | EPUB
File size: 45.5 MB
Language: ENG
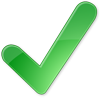
The book "Shallow Learning vs Deep Learning A Practical Guide for Machine Learning Solutions" explores the differences between shallow learning and deep learning, two fundamental approaches to machine learning that have revolutionized the field of artificial intelligence. The author, a renowned expert in the field, provides a comprehensive overview of these techniques, explaining their strengths and weaknesses, and offering practical guidance on how to choose the right approach for your specific needs. The book begins by defining the key concepts of shallow and deep learning, and discussing the historical context in which they emerged. It then delves into the technical details of each approach, highlighting their unique characteristics and applications. The author also provides examples of real-world scenarios where one or the other approach may be more appropriate, based on factors such as data availability, computational resources, and desired outcomes. As the book progresses, the author shifts focus to the challenges and limitations of both shallow and deep learning, and how they can be addressed through careful consideration of the underlying assumptions and constraints of each approach. He also offers insights into the future of machine learning, and how it is likely to evolve in response to advances in technology and societal needs. Throughout the book, the author emphasizes the importance of understanding the process of technological evolution, and how this can inform our choices about which approaches to use in different situations. He argues that by studying the history and development of machine learning, we can gain a deeper appreciation for the field's potential and limitations, and make more informed decisions about its application in various domains.
''
