
BOOKS - Machine Learning for Materials Discovery Numerical Recipes and Practical Appl...

Machine Learning for Materials Discovery Numerical Recipes and Practical Applications
Author: N. M. Anoop Krishnan, Hariprasad Kodamana, Ravinder Bhattoo
Year: 2024
Pages: 287
Format: PDF
File size: 11.5 MB
Language: ENG
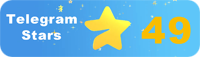
Year: 2024
Pages: 287
Format: PDF
File size: 11.5 MB
Language: ENG
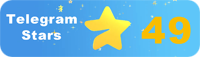
Book Machine Learning for Materials Discovery Numerical Recipes and Practical Applications Introduction: In today's fast-paced technological era, it is essential to understand the process of technology evolution and its impact on humanity. The development of modern knowledge is the key to survival, and the unification of people in a warring state is crucial. This book, "Machine Learning for Materials Discovery: Numerical Recipes and Practical Applications provides a comprehensive guide to machine learning techniques that can be applied to materials discovery and modeling. It covers broad areas of data-driven modeling, from simple regression to advanced machine learning and optimization methods, making it an indispensable resource for graduate students, researchers, and engineers working in materials science. Chapter 1: Fundamentals of Machine Learning The first chapter delves into the fundamentals of machine learning, providing a solid foundation for understanding the concepts presented throughout the book. It explains complex mathematical ideas in a clear and concise manner, ensuring that readers from diverse backgrounds can successfully use these techniques. The chapter explores the transformative impact of deep learning compared to classical approaches, which heavily rely on handcrafted features and hyperparameter tuning.
Book Machine arning for Materials Discovery Числовые рецепты и практические применения Введение: В современную быстро развивающуюся технологическую эру важно понимать процесс эволюции технологий и его влияние на человечество. Развитие современных знаний - залог выживания, а объединение людей в воюющем государстве имеет решающее значение. В этой книге «Машинное обучение для обнаружения материалов: числовые рецепты и практические приложения» представлено исчерпывающее руководство по методам машинного обучения, которые могут быть применены к обнаружению и моделированию материалов. Он охватывает широкие области моделирования на основе данных, от простой регрессии до передовых методов машинного обучения и оптимизации, что делает его незаменимым ресурсом для аспирантов, исследователей и инженеров, работающих в области материаловедения. Глава 1: Основы машинного обучения Первая глава углубляется в основы машинного обучения, обеспечивая прочную основу для понимания концепций, представленных на протяжении всей книги. Он объясняет сложные математические идеи в ясной и сжатой форме, гарантируя, что читатели из разных слоев общества могут успешно использовать эти методы. Глава исследует преобразующее влияние глубокого обучения по сравнению с классическими подходами, которые в значительной степени опираются на функции ручной работы и настройку гиперпараметров.
Book Machine arning for Materials Discovery Recettes numériques et applications pratiques Introduction : Dans une ère technologique moderne en évolution rapide, il est important de comprendre le processus d'évolution de la technologie et son impact sur l'humanité. développement des connaissances modernes est la clé de la survie, et l'unification des personnes dans un État en guerre est cruciale. Ce livre « L'apprentissage automatique pour la découverte de matériaux : recettes numériques et applications pratiques » présente un guide complet des techniques d'apprentissage automatique qui peuvent être appliquées à la détection et à la modélisation de matériaux. Il couvre de vastes domaines de la modélisation basée sur les données, de la simple régression aux techniques avancées d'apprentissage automatique et d'optimisation, ce qui en fait une ressource indispensable pour les étudiants diplômés, les chercheurs et les ingénieurs travaillant dans le domaine de la science des matériaux. Chapitre 1 : s bases de l'apprentissage automatique premier chapitre approfondit les bases de l'apprentissage automatique, fournissant une base solide pour comprendre les concepts présentés tout au long du livre. Il explique les idées mathématiques complexes sous une forme claire et concise, en veillant à ce que les lecteurs de différents milieux puissent utiliser ces méthodes avec succès. chapitre explore l'impact transformateur de l'apprentissage profond par rapport aux approches classiques, qui reposent en grande partie sur les fonctions manuelles et la personnalisation des hyperparamètres.
Book Machine Arning for Materials Discovery Recetas numéricas y aplicaciones prácticas Introducción: En la era tecnológica actual, en rápida evolución, es importante comprender el proceso de evolución de la tecnología y su impacto en la humanidad. desarrollo del conocimiento moderno es la clave de la supervivencia, y la unión de las personas en un Estado en guerra es crucial. Este libro, «Machine arning para la detección de materiales: recetas numéricas y aplicaciones prácticas», presenta una guía exhaustiva sobre técnicas de aprendizaje automático que se pueden aplicar a la detección y modelado de materiales. Abarca amplias áreas de modelado basado en datos, desde la regresión simple hasta técnicas avanzadas de aprendizaje automático y optimización, lo que lo convierte en un recurso indispensable para los estudiantes de posgrado, investigadores e ingenieros que trabajan en el campo de la ciencia de materiales. Capítulo 1: Fundamentos del aprendizaje automático primer capítulo profundiza en los fundamentos del aprendizaje automático, proporcionando una base sólida para comprender los conceptos presentados a lo largo del libro. Explica las complejas ideas matemáticas en una forma clara y concisa, asegurando que los lectores de diferentes sectores de la sociedad puedan utilizar con éxito estas técnicas. capítulo explora el impacto transformador del aprendizaje profundo en comparación con los enfoques clásicos, que se basan en gran medida en las funciones artesanales y la personalización de los hiperparámetros.
Buchmaschine rnen für Materialien Entdeckung Numerische Rezepte und praktische Anwendungen Einleitung: In der heutigen schnelllebigen technologischen Ära ist es wichtig, den Prozess der technologischen Evolution und seine Auswirkungen auf die Menschheit zu verstehen. Die Entwicklung des modernen Wissens ist der Schlüssel zum Überleben, und die Vereinigung der Menschen in einem kriegführenden Staat ist von entscheidender Bedeutung. Dieses Buch, Machine arning for Material Discovery: Numerische Rezepte und praktische Anwendungen, bietet einen umfassenden itfaden für Methoden des maschinellen rnens, die auf die Materialdetektion und -modellierung angewendet werden können. Es deckt breite Bereiche der datenbasierten Modellierung ab, von der einfachen Regression bis hin zu fortschrittlichen Methoden des maschinellen rnens und der Optimierung, was es zu einer unverzichtbaren Ressource für Doktoranden, Forscher und Ingenieure macht, die auf dem Gebiet der Materialwissenschaften arbeiten. Kapitel 1: Grundlagen des maschinellen rnens Das erste Kapitel befasst sich mit den Grundlagen des maschinellen rnens und bietet eine solide Grundlage für das Verständnis der Konzepte, die im gesamten Buch vorgestellt werden. Es erklärt komplexe mathematische Ideen in klarer und prägnanter Form und stellt sicher, dass ser mit unterschiedlichem Hintergrund diese Techniken erfolgreich anwenden können. Das Kapitel untersucht die transformative Wirkung von Deep arning im Vergleich zu klassischen Ansätzen, die stark auf handgefertigte Funktionen und die Einstellung von Hyperparametern setzen.
''
Malzeme Keşfi İçin Arning Kitap Makinesi Sayısal tarifler ve pratik uygulamalar Giriş: Günümüzün hızla gelişen teknolojik çağında, teknoloji evrimi sürecini ve insanlık üzerindeki etkisini anlamak önemlidir. Modern bilginin gelişimi hayatta kalmanın anahtarıdır ve insanların savaşan bir durumda birleşmesi çok önemlidir. Bu kitap, "Malzeme Keşfi için Makine Öğrenimi: Sayısal Tarifler ve Pratik Uygulamalar", malzeme keşfi ve modellemesine uygulanabilecek makine öğrenme teknikleri için kapsamlı bir rehber sunmaktadır. Basit regresyondan gelişmiş makine öğrenimi ve optimizasyon tekniklerine kadar geniş veri odaklı modelleme alanlarını kapsar, bu da onu malzeme bilimi alanında çalışan lisansüstü öğrenciler, araştırmacılar ve mühendisler için vazgeçilmez bir kaynak haline getirir. Bölüm 1: Makine Öğreniminin Temelleri İlk bölüm, makine öğreniminin temellerini inceler ve kitap boyunca sunulan kavramları anlamak için sağlam bir temel sağlar. Karmaşık matematiksel fikirleri açık ve özlü bir şekilde açıklayarak, farklı yaşam alanlarından okuyucuların bu teknikleri başarıyla kullanabilmelerini sağlar. Bu bölüm, derin öğrenmenin, el yapımı özelliklere ve hiper parametre ayarına dayanan klasik yaklaşımlara kıyasla dönüştürücü etkisini araştırıyor.
كتاب التعلم الآلي للمواد اكتشاف الوصفات الرقمية والتطبيقات العملية مقدمة: في العصر التكنولوجي سريع التطور اليوم، من المهم فهم تطور التكنولوجيا وتأثيرها على البشرية. تطوير المعرفة الحديثة هو مفتاح البقاء، وتوحيد الناس في دولة متحاربة أمر بالغ الأهمية. يقدم هذا الكتاب، «التعلم الآلي لاكتشاف المواد: الوصفات العددية والتطبيقات العملية»، دليلاً شاملاً لتقنيات التعلم الآلي التي يمكن تطبيقها على اكتشاف المواد والنمذجة. يغطي مجالات واسعة من النمذجة القائمة على البيانات، من الانحدار البسيط إلى تقنيات التعلم الآلي المتقدمة والتحسين، مما يجعله موردًا لا غنى عنه لطلاب الدراسات العليا والباحثين والمهندسين العاملين في علوم المواد. الفصل 1: أساسيات التعلم الآلي يتعمق الفصل الأول في أساسيات التعلم الآلي، مما يوفر أساسًا صلبًا لفهم المفاهيم المقدمة في جميع أنحاء الكتاب. يشرح الأفكار الرياضية المعقدة بطريقة واضحة وموجزة، مما يضمن أن القراء من مختلف مناحي الحياة يمكنهم استخدام هذه التقنيات بنجاح. يستكشف الفصل التأثير التحويلي للتعلم العميق مقارنة بالمناهج الكلاسيكية، التي تعتمد بشكل كبير على الميزات المصنوعة يدويًا وضبط هايبربارامتر.
