
BOOKS - Machine Learning for Materials Discovery Numerical Recipes and Practical Appl...

Machine Learning for Materials Discovery Numerical Recipes and Practical Applications
Author: N. M. Anoop Krishnan, Hariprasad Kodamana, Ravinder Bhattoo
Year: 2024
Pages: 287
Format: PDF
File size: 11.5 MB
Language: ENG
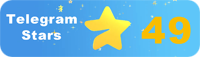
Year: 2024
Pages: 287
Format: PDF
File size: 11.5 MB
Language: ENG
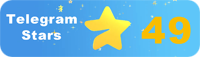
The book "Machine Learning for Materials Discovery: Numerical Recipes and Practical Applications" is a comprehensive guide to understanding the potential of machine learning in materials science and its practical applications. The author, Dr. N. M. Anoop Krishnan, Hariprasad Kodamana, Ravinder Bhattoo, is a renowned expert in the field of materials science and machine learning, and has written this book to provide readers with a thorough understanding of the current state of the art in machine learning techniques and their application to materials discovery. The book begins by introducing the concept of machine learning and its relevance to materials science, highlighting the need for a paradigm shift in how we approach the development of new materials. The author argues that traditional methods of materials discovery are no longer sufficient to meet the demands of modern technology, and that machine learning offers a powerful tool for accelerating the process of materials discovery and optimization. The book then delves into the details of various machine learning algorithms and their applications in materials science, including supervised and unsupervised learning, deep learning, and reinforcement learning. Each chapter provides a detailed explanation of the underlying principles of each algorithm, as well as practical examples of how they can be applied to materials discovery. The author also emphasizes the importance of data quality and preprocessing, highlighting the need for careful curation of datasets to ensure accurate predictions. One of the key themes of the book is the need for interdisciplinary collaboration between materials scientists, computer scientists, and engineers to develop effective machine learning models for materials discovery.
Книга «Machine arning for Materials Discovery: Numerical Recipes and Practical Applications» является всеобъемлющим руководством для понимания потенциала машинного обучения в материаловедении и его практических применений. Автор, доктор Н. М. Ануп Кришнан, Харипрасад Кодамана, Равиндер Бхатто, является известным экспертом в области материаловедения и машинного обучения, и написал эту книгу, чтобы дать читателям полное понимание современного состояния техники машинного обучения и их применение к обнаружению материалов. Книга начинается с введения понятия машинного обучения и его актуальности для материаловедения, подчеркивая необходимость смены парадигмы в том, как мы подходим к разработке новых материалов. Автор утверждает, что традиционных методов обнаружения материалов уже недостаточно для удовлетворения требований современных технологий, и что машинное обучение предлагает мощный инструмент для ускорения процесса обнаружения и оптимизации материалов. Затем книга углубляется в детали различных алгоритмов машинного обучения и их применения в материаловедении, включая контролируемое и неконтролируемое обучение, глубокое обучение и обучение с подкреплением. Каждая глава содержит подробное объяснение основных принципов каждого алгоритма, а также практические примеры того, как они могут быть применены к обнаружению материалов. Автор также подчеркивает важность качества данных и предварительной обработки, подчеркивая необходимость тщательной обработки наборов данных для обеспечения точных прогнозов. Одной из ключевых тем книги является необходимость междисциплинарного сотрудничества между материаловедами, компьютерщиками и инженерами для разработки эффективных моделей машинного обучения для обнаружения материалов.
''
