
BOOKS - PROGRAMMING - Knowledge-Guided Machine Learning Accelerating Discovery Using ...

Knowledge-Guided Machine Learning Accelerating Discovery Using Scientific Knowledge and Data
Author: Edited by Anuj Karpatne, Ramakrishnan Kannan, Vipin Kumar
Year: 2023
Pages: 442
Format: PDF
File size: 91.33 MB
Language: ENG
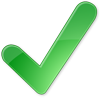
Year: 2023
Pages: 442
Format: PDF
File size: 91.33 MB
Language: ENG
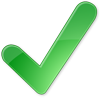
. The author proposes a new approach to ML that combines the strengths of both scientific knowledge and data-driven discovery to develop more accurate, interpretable and flexible models. The key idea is to leverage domain knowledge from experts in various fields to guide the development of ML models that can handle complex realworld problems with incomplete and uncertain information. This approach is based on the authors experience in developing ML models for challenging domains such as medical diagnosis and environmental monitoring. The proposed framework consists of three stages: 1) Knowledge Acquisition, 2) Model Development and 3) Evaluation and Validation. The author provides a detailed discussion of each stage and how it can be applied to different domains. The book concludes by highlighting the potential benefits of this approach for accelerating discovery and improving decision making in various fields. The book also includes examples from various domains to illustrate the power of the proposed approach and provide insights into the challenges and opportunities of integrating scientific knowledge and machine learning. The text includes practical tips and recommendations for implementing the approach in realworld settings. The target audience for this book is researchers, practitioners, and students who are interested in applying machine learning to solve complex problems in various domains. The need to study and understand the process of technology evolution has become increasingly important as we enter an era where technological advancements are rapidly transforming our society and shaping our lives.
. Автор предлагает новый подход к ML, который сочетает в себе сильные стороны как научных знаний, так и основанных на данных открытий для разработки более точных, интерпретируемых и гибких моделей. Ключевая идея состоит в том, чтобы использовать знания в области от экспертов в различных областях, чтобы направлять разработку ML-моделей, которые могут решать сложные проблемы реального мира с неполной и неопределенной информацией. Этот подход основан на опыте авторов в разработке моделей ML для сложных областей, таких как медицинская диагностика и мониторинг окружающей среды. Предлагаемая структура состоит из трех этапов: 1) получение знаний, 2) разработка модели и 3) оценка и валидация. Автор предоставляет подробное обсуждение каждого этапа и того, как его можно применить к различным областям. В заключение книга подчеркивает потенциальные преимущества такого подхода для ускорения открытия и улучшения принятия решений в различных областях. Книга также включает примеры из различных областей, чтобы проиллюстрировать силу предлагаемого подхода и дать представление о проблемах и возможностях интеграции научных знаний и машинного обучения. Текст включает практические советы и рекомендации по реализации подхода в реальных условиях. Целевая аудитория этой книги - исследователи, практики и студенты, заинтересованные в применении машинного обучения для решения сложных задач в различных областях. Необходимость изучения и понимания процесса эволюции технологий становится все более важной по мере того, как мы вступаем в эпоху, когда технологические достижения быстро преобразуют наше общество и формируют нашу жизнь.
. L'auteur propose une nouvelle approche de ML qui combine les forces des connaissances scientifiques et des découvertes basées sur des données pour développer des modèles plus précis, interprétables et flexibles. L'idée clé est d'utiliser les connaissances du domaine des experts dans différents domaines pour guider le développement de modèles ML qui peuvent résoudre les problèmes complexes du monde réel avec des informations incomplètes et incertaines. Cette approche est basée sur l'expérience des auteurs dans le développement de modèles ML dans des domaines complexes tels que le diagnostic médical et la surveillance de l'environnement. cadre proposé comprend trois étapes : 1) acquisition de connaissances, 2) élaboration de modèles et 3) évaluation et validation. L'auteur donne une discussion détaillée de chaque étape et de la façon dont elle peut être appliquée à différents domaines. En conclusion, le livre souligne les avantages potentiels d'une telle approche pour accélérer la découverte et améliorer la prise de décisions dans différents domaines. livre contient également des exemples de différents domaines pour illustrer la force de l'approche proposée et donner un aperçu des défis et des possibilités d'intégration des connaissances scientifiques et de l'apprentissage automatique. texte contient des conseils pratiques et des recommandations sur la mise en œuvre de l'approche en conditions réelles. public cible de ce livre sont des chercheurs, des praticiens et des étudiants intéressés à appliquer l'apprentissage automatique pour relever des défis complexes dans différents domaines. La nécessité d'étudier et de comprendre le processus d'évolution de la technologie devient de plus en plus importante à mesure que nous entrons dans une ère où les progrès technologiques transforment rapidement notre société et façonnent nos vies.
. autor propone un nuevo enfoque del ML que combina las fortalezas tanto del conocimiento científico como de los descubrimientos basados en datos para desarrollar modelos más precisos, interpretables y flexibles. La idea clave es aprovechar el conocimiento en el campo de expertos en diferentes campos para orientar el desarrollo de modelos ML que puedan resolver los complejos problemas del mundo real con información incompleta e incierta. Este enfoque se basa en la experiencia de los autores en el desarrollo de modelos de LM para áreas complejas como el diagnóstico médico y el monitoreo ambiental. La estructura propuesta consta de tres fases: 1) adquisición de conocimientos, 2) elaboración del modelo y 3) evaluación y validación. autor proporciona una discusión detallada de cada etapa y cómo se puede aplicar a diferentes áreas. En conclusión, el libro destaca los posibles beneficios de este enfoque para acelerar la apertura y mejorar la toma de decisiones en diferentes áreas. libro también incluye ejemplos de diferentes áreas para ilustrar el poder del enfoque propuesto y dar una idea de los desafíos y oportunidades de integrar el conocimiento científico y el aprendizaje automático. texto incluye consejos prácticos y recomendaciones para implementar el enfoque en condiciones reales. público objetivo de este libro son investigadores, practicantes y estudiantes interesados en aplicar el aprendizaje automático para resolver problemas complejos en diferentes campos. La necesidad de estudiar y entender el proceso de evolución de la tecnología es cada vez más importante a medida que entramos en una era en la que los avances tecnológicos transforman rápidamente nuestra sociedad y moldean nuestras vidas.
. O autor propõe uma nova abordagem do ML, que combina os pontos fortes tanto do conhecimento científico quanto das descobertas baseadas em dados para desenvolver modelos mais precisos, interpretáveis e flexíveis. A ideia chave é usar o conhecimento da área de especialistas em várias áreas para orientar o desenvolvimento de modelos ML que podem resolver problemas complexos do mundo real com informações incompletas e incertas. Esta abordagem é baseada na experiência dos autores no desenvolvimento de modelos ML para áreas complexas, como diagnóstico médico e monitoramento ambiental. A estrutura proposta consiste em três etapas: 1) conhecimento, 2) desenvolvimento de modelo e 3) avaliação e validação. O autor fornece uma discussão detalhada sobre cada etapa e como pode ser aplicada a diferentes áreas. Para terminar, o livro ressalta os potenciais benefícios dessa abordagem para acelerar a descoberta e melhorar a tomada de decisões em várias áreas. O livro também inclui exemplos de várias áreas para ilustrar o poder da abordagem proposta e dar uma ideia dos desafios e possibilidades de integração entre o conhecimento científico e o aprendizado de máquinas. O texto inclui conselhos práticos e recomendações para implementar a abordagem em condições reais. O público-alvo deste livro são pesquisadores, práticas e estudantes interessados em usar o aprendizado de máquinas para resolver tarefas complexas em várias áreas. A necessidade de explorar e compreender a evolução da tecnologia é cada vez mais importante à medida que entramos numa era em que os avanços tecnológicos transformam rapidamente a nossa sociedade e formam nossas vidas.
. L'autore propone un nuovo approccio al ML, che combina i punti di forza sia delle conoscenze scientifiche che delle scoperte basate su dati per sviluppare modelli più precisi, interpretabili e flessibili. L'idea chiave è quella di utilizzare le conoscenze provenienti da esperti in diversi settori per guidare lo sviluppo di modelli ML in grado di affrontare i problemi complessi del mondo reale con informazioni incomplete e incerte. Questo approccio si basa sull'esperienza degli autori nello sviluppo di modelli ML per aree complesse come la diagnostica medica e il monitoraggio ambientale. La struttura proposta si compone di tre fasi: 1) acquisizione della conoscenza, 2) sviluppo del modello e 3) valutazione e validazione. L'autore fornisce una discussione dettagliata su ogni fase e su come applicarla a diverse aree. In conclusione, il libro sottolinea i potenziali vantaggi di questo approccio per accelerare la scoperta e migliorare la presa di decisioni in diversi ambiti. Il libro include anche esempi provenienti da diversi ambiti per illustrare la forza dell'approccio proposto e dare un'idea delle sfide e delle opportunità di integrazione tra conoscenza scientifica e apprendimento automatico. Il testo include suggerimenti pratici e suggerimenti per l'implementazione dell'approccio in condizioni reali. Il pubblico target di questo libro sono ricercatori, pratici e studenti interessati a utilizzare l'apprendimento automatico per affrontare le sfide in diversi ambiti. La necessità di studiare e comprendere l'evoluzione della tecnologia diventa sempre più importante quando entriamo in un'epoca in cui i progressi tecnologici stanno rapidamente trasformando la nostra società e formando le nostre vite.
. Der Autor schlägt einen neuen Ansatz für ML vor, der die Stärken sowohl wissenschaftlicher Erkenntnisse als auch datenbasierter Entdeckungen kombiniert, um präzisere, interpretierbare und flexiblere Modelle zu entwickeln. Die Schlüsselidee besteht darin, das Fachwissen von Experten in verschiedenen Bereichen zu nutzen, um die Entwicklung von ML-Modellen zu leiten, die komplexe Probleme der realen Welt mit unvollständigen und unsicheren Informationen lösen können. Dieser Ansatz basiert auf der Expertise der Autoren bei der Entwicklung von ML-Modellen für komplexe Bereiche wie medizinische Diagnostik und Umweltüberwachung. Der vorgeschlagene Rahmen besteht aus drei Phasen: 1) Wissenserwerb, 2) Modellentwicklung und 3) Bewertung und Validierung. Der Autor bietet eine detaillierte Diskussion über jede Phase und wie sie auf verschiedene Bereiche angewendet werden kann. Abschließend hebt das Buch die potenziellen Vorteile eines solchen Ansatzes hervor, um die Entdeckung zu beschleunigen und die Entscheidungsfindung in verschiedenen Bereichen zu verbessern. Das Buch enthält auch Beispiele aus verschiedenen Bereichen, um die Stärke des vorgeschlagenen Ansatzes zu veranschaulichen und Einblicke in die Herausforderungen und Möglichkeiten der Integration von wissenschaftlichem Wissen und maschinellem rnen zu geben. Der Text enthält praktische Tipps und Tricks zur Umsetzung des Ansatzes unter realen Bedingungen. Die Zielgruppe dieses Buches sind Forscher, Praktiker und Studenten, die daran interessiert sind, maschinelles rnen anzuwenden, um komplexe Probleme in verschiedenen Bereichen zu lösen. Die Notwendigkeit, den Prozess der Technologieentwicklung zu studieren und zu verstehen, wird immer wichtiger, da wir in ein Zeitalter eintreten, in dem technologische Fortschritte unsere Gesellschaft schnell verändern und unser ben prägen.
. Autor proponuje nowe podejście do ML, które łączy w sobie mocne strony zarówno wiedzy naukowej, jak i odkryć opartych na danych, aby opracować dokładniejsze, bardziej interpretowalne i elastyczne modele. Kluczowym pomysłem jest wykorzystanie wiedzy z dziedziny od ekspertów z różnych dziedzin do kierowania rozwojem modeli ML, które mogą rozwiązać złożone problemy świata rzeczywistego z niekompletnymi i niepewnymi informacjami. Podejście to opiera się na doświadczeniach autorów w opracowywaniu modeli ML dla złożonych dziedzin, takich jak diagnostyka medyczna i monitorowanie środowiska. Proponowane ramy składają się z trzech etapów: 1) nabywania wiedzy, 2) opracowywania modelu oraz 3) oceny i walidacji. Autor przedstawia szczegółową dyskusję na temat każdego kroku i sposobu jego zastosowania w różnych dziedzinach. Podsumowując, książka podkreśla potencjalne korzyści płynące z tego podejścia, aby przyspieszyć odkrycie i usprawnić proces decyzyjny w różnych dziedzinach. Książka zawiera również przykłady z różnych dziedzin, aby zilustrować siłę proponowanego podejścia i zapewnić wgląd w wyzwania i możliwości integracji wiedzy naukowej i uczenia maszynowego. Tekst zawiera praktyczne wskazówki i sztuczki do wdrożenia podejścia w świecie rzeczywistym. Docelowym odbiorcą tej książki są badacze, praktycy i studenci zainteresowani zastosowaniem uczenia maszynowego do rozwiązywania złożonych problemów w różnych dziedzinach. Potrzeba badania i zrozumienia ewolucji technologii staje się coraz ważniejsza wraz z wejściem w erę, w której postęp technologiczny szybko przekształca nasze społeczeństwo i kształtuje nasze życie.
. המחבר מציע גישה חדשנית ל-ML המשלבת את נקודות החוזק של הידע המדעי וגם גילוי מונע נתונים לפיתוח מודלים מדויקים יותר, מפרשים וגמישים יותר. הרעיון המרכזי הוא להשתמש בידע בתחום ממומחים בתחומים שונים כדי להנחות פיתוח מודלים של ML שיכולים לפתור בעיות מורכבות בעולם האמיתי באמצעות מידע לא שלם ולא בטוח. גישה זו בונה על ניסיון המחברים בפיתוח מודלים של ML לתחומים מורכבים כמו אבחון רפואי וניטור סביבתי. המסגרת המוצעת כוללת שלושה שלבים: 1) רכישת ידע, 2) פיתוח מודל ו-3) הערכה ואימות. המחבר מספק דיון מפורט על כל צעד וכיצד ניתן לייחס אותו לתחומים שונים. לסיכום, הספר מדגיש את היתרונות האפשריים של גישה זו כדי להאיץ את הגילוי ולשפר את קבלת ההחלטות בתחומים שונים. הספר כולל גם דוגמאות מתחומים שונים כדי להמחיש את כוחה של הגישה המוצעת ולספק תובנה על האתגרים וההזדמנויות של שילוב ידע מדעי ולימוד מכונה. הטקסט כולל טיפים וטריקים מעשיים ליישום הגישה בעולם האמיתי. קהל היעד של ספר זה הוא חוקרים, חוקרים וסטודנטים המעוניינים ליישם למידת מכונה לפתרון בעיות מורכבות בתחומים שונים. הצורך לחקור ולהבין את התפתחות הטכנולוגיה הופך להיות יותר ויותר חשוב כשאנו נכנסים לעידן שבו ההתקדמות הטכנולוגית משנה במהירות את החברה שלנו ומעצבת את חיינו.''
. Yazar, daha doğru, yorumlanabilir ve esnek modeller geliştirmek için hem bilimsel bilginin hem de veri odaklı keşfin güçlü yönlerini birleştiren ML'ye yeni bir yaklaşım önermektedir. Temel fikir, karmaşık gerçek dünya sorunlarını eksik ve belirsiz bilgilerle çözebilecek ML modellerinin geliştirilmesine rehberlik etmek için çeşitli alanlardaki uzmanlardan gelen bilgileri kullanmaktır. Bu yaklaşım, yazarların tıbbi teşhis ve çevresel izleme gibi karmaşık alanlar için ML modelleri geliştirme konusundaki deneyimlerine dayanmaktadır. Önerilen çerçeve üç aşamadan oluşmaktadır: 1) bilgi edinme, 2) model geliştirme ve 3) değerlendirme ve doğrulama. Yazar, her adımın ve farklı alanlara nasıl uygulanabileceğinin ayrıntılı bir tartışmasını sunar. Sonuç olarak, kitap keşfi hızlandırmak ve çeşitli alanlarda karar vermeyi geliştirmek için bu yaklaşımın potansiyel faydalarını vurgulamaktadır. Kitap ayrıca, önerilen yaklaşımın gücünü göstermek ve bilimsel bilgi ve makine öğrenimini entegre etmenin zorlukları ve fırsatları hakkında fikir vermek için çeşitli alanlardan örnekler içermektedir. Metin, yaklaşımı gerçek dünya ortamında uygulamak için pratik ipuçları ve püf noktaları içerir. Bu kitabın hedef kitlesi, çeşitli alanlardaki karmaşık problemleri çözmek için makine öğrenimini uygulamak isteyen araştırmacılar, uygulayıcılar ve öğrencilerdir. Teknolojinin evrimini inceleme ve anlama ihtiyacı, teknolojik gelişmelerin toplumumuzu hızla dönüştürdüğü ve yaşamlarımızı şekillendirdiği bir döneme girerken giderek daha önemli hale geliyor.
. يقترح المؤلف نهجًا جديدًا تجاه ML يجمع بين نقاط القوة في كل من المعرفة العلمية والاكتشاف القائم على البيانات لتطوير نماذج أكثر دقة وقابلة للتفسير ومرونة. الفكرة الرئيسية هي استخدام المعرفة في هذا المجال من الخبراء في مختلف المجالات لتوجيه تطوير نماذج ML التي يمكن أن تحل مشاكل العالم الحقيقي المعقدة بمعلومات غير كاملة وغير مؤكدة. يعتمد هذا النهج على تجربة المؤلفين في تطوير نماذج ML لمجالات معقدة مثل التشخيص الطبي والمراقبة البيئية. ويتألف الإطار المقترح من ثلاث مراحل: 1) اكتساب المعارف، 2) تطوير النماذج، 3) التقييم والتصديق. يقدم المؤلف مناقشة مفصلة لكل خطوة وكيف يمكن تطبيقها على مجالات مختلفة. في الختام، يسلط الكتاب الضوء على الفوائد المحتملة لهذا النهج لتسريع الاكتشاف وتحسين صنع القرار في مختلف المجالات. يتضمن الكتاب أيضًا أمثلة من مختلف المجالات لتوضيح قوة النهج المقترح وتقديم نظرة ثاقبة لتحديات وفرص دمج المعرفة العلمية والتعلم الآلي. يتضمن النص نصائح وحيل عملية لتنفيذ النهج في بيئة واقعية. الجمهور المستهدف لهذا الكتاب هو الباحثون والممارسون والطلاب المهتمون بتطبيق التعلم الآلي لحل المشكلات المعقدة في مختلف المجالات. تزداد أهمية الحاجة إلى دراسة وفهم تطور التكنولوجيا مع دخولنا عصرًا يؤدي فيه التقدم التكنولوجي إلى تغيير مجتمعنا بسرعة وتشكيل حياتنا.
. 저자는 과학 지식과 데이터 중심 발견의 강점을 결합하여보다 정확하고 해석 가능하며 유연한 모델을 개발하는 ML에 대한 새로운 접근 방식을 제안합니다. 핵심 아이디어는 다양한 분야의 전문가들로부터 해당 분야의 지식을 사용하여 불완전하고 불확실한 정보로 복잡한 실제 문제를 해결할 수있는 ML 모델의 개발을 안내하는 것입니다. 이 접근법은 의료 진단 및 환경 모니터링과 같은 복잡한 분야를위한 ML 모델 개발에 대한 저자의 경험을 바탕으로합니다. 제안 된 프레임 워크는 1) 지식 습득, 2) 모델 개발 및 3) 평가 및 검증의 세 단계로 구성됩니다. 저자는 각 단계에 대한 자세한 설명과 다른 영역에 적용할 수있는 방법을 제공합니다. 결론적으로이 책은 다양한 분야에서 발견을 가속화하고 의사 결정을 개선하기위한이 접근 방식의 잠재적 이점을 강조합니다. 이 책에는 또한 제안 된 접근 방식의 힘을 설명하고 과학 지식과 기계 학습을 통합하는 과제와 기회에 대한 통찰력을 제공하기위한 다양한 분야의 예가 포함되어 있습니다. 이 텍스트에는 실제 환경에서 접근 방식을 구현하기위한 실용적인 팁과 요령이 포함되어 있습니다 이 책의 대상 고객은 다양한 분야의 복잡한 문제를 해결하기 위해 기계 학습을 적용하는 데 관심이있는 연구원, 실무자 및 학생입니다 기술 발전이 사회를 빠르게 변화시키고 삶을 형성하는 시대에 접어 들면서 기술의 진화를 연구하고 이해해야 할 필요성이 점점 중요 해지고 있습니다.
.著者は、科学的知識とデータ主導の発見の両方の強みを組み合わせて、より正確で解釈可能で柔軟なモデルを開発するMLへの新しいアプローチを提案します。主要なアイデアは、不完全で不確実な情報を持つ複雑な現実の問題を解決できるMLモデルの開発を導くために、様々な分野の専門家からの知識を使用することです。このアプローチは、医療診断や環境モニタリングなどの複雑な分野におけるMLモデルの開発における著者の経験に基づいています。提案されたフレームワークは、1)知識獲得、2)モデル開発、3)評価と検証の3段階で構成されています。著者は、各ステップの詳細な議論を提供し、それが異なる分野に適用することができます。結論として、本書は、このアプローチが様々な分野での発見を加速し、意思決定を改善するための潜在的な利点を強調しています。この本には、提案されたアプローチの力を説明し、科学的知識と機械学習を統合する課題と機会についての洞察を提供するためのさまざまな分野の例も含まれています。このテキストには、現実世界の設定でアプローチを実装するための実用的なヒントとコツが含まれています。本書の対象となるのは、様々な分野の複雑な問題の解決に機械学習を応用することに興味を持つ研究者、実践者、学生です。技術の進歩が急速に社会を変革し、私たちの生活を形作る時代に入るにつれて、技術の進化を研究し理解する必要性はますます重要になっています。
.作者提出了一種針對ML的新方法,該方法結合了科學知識和基於數據的發現的優勢,以開發更準確,可解釋和靈活的模型。一個關鍵的想法是利用來自不同領域專家的領域知識,指導ML模型的發展,這些模型可以通過不完整和不確定的信息來解決現實世界的復雜問題。這種方法基於作者為醫學診斷和環境監測等復雜領域開發ML模型的經驗。擬議的結構分為三個階段:(1)知識獲得;(2)建立模型;(3)評估和驗證。作者詳細討論了每個階段以及如何將其應用於各個領域。最後,該書強調了這種方法在加快發現和改善不同領域的決策方面的潛在好處。該書還包括來自各個領域的示例,以說明擬議方法的力量,並深入了解整合科學知識和機器學習的挑戰和機會。該案文包括關於在實際環境中實施該辦法的實用建議和建議。本書的目標受眾是研究人員,從業人員和學生,他們對應用機器學習來解決各個領域的復雜問題感興趣。隨著我們進入一個技術進步正在迅速改變我們的社會和塑造我們生活的時代,探索和理解技術演變過程的必要性變得越來越重要。
