
BOOKS - SCIENCE AND STUDY - Fundamental Statistical Inference A Computational Approac...

Fundamental Statistical Inference A Computational Approach
Author: Marc S. Paolella
Year: 2018
Pages: 572
Format: PDF
File size: 11,89 MB
Language: ENG
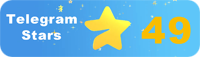
Year: 2018
Pages: 572
Format: PDF
File size: 11,89 MB
Language: ENG
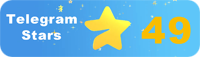
''
