
BOOKS - SCIENCE AND STUDY - Foundations of Statistical Algorithms With References to ...

Foundations of Statistical Algorithms With References to R Packages
Author: Claus Weihs, Olaf Mersmann, Uwe Ligges
Year: 2013
Pages: 500
Format: PDF
File size: 3 MB
Language: ENG
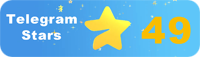
Year: 2013
Pages: 500
Format: PDF
File size: 3 MB
Language: ENG
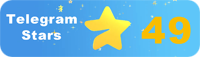
Book Description: The book "Foundations of Statistical Algorithms with References to R Packages" provides a comprehensive overview of the fundamental concepts and techniques of statistical algorithms, along with practical examples and references to relevant R packages. The book covers topics such as probability distributions, statistical inference, linear regression, time series analysis, and machine learning, among others. It is designed for students, researchers, and practitioners who want to gain a deeper understanding of statistical algorithms and their applications in R programming. - Long Description: In today's fast-paced world, technology is constantly evolving, and it is crucial to understand the process of technological advancements to stay ahead of the curve. The book "Foundations of Statistical Algorithms with References to R Packages" offers a unique perspective on the development of modern knowledge and its impact on humanity. By exploring the foundations of statistical algorithms and their practical applications, readers will gain a deeper understanding of how technology has shaped our society and how it can be used to unify people in a warring state. The book begins by discussing the importance of probability distributions, which form the basis of statistical analysis. It explains the different types of distributions, including the normal distribution, binomial distribution, and Poisson distribution, and their applications in real-world scenarios. The authors then delve into statistical inference, which involves making inferences about a population based on a sample of data. They cover various techniques such as hypothesis testing, confidence intervals, and regression analysis, providing readers with a solid foundation in statistical inference. Linear regression is another critical topic covered in the book, where the authors explain the principles of linear regression, including simple and multiple linear regression, and their applications in modeling real-world phenomena.
''
