
BOOKS - PROGRAMMING - Statistical Machine Learning A Unified Framework (Chapman & Hal...

Statistical Machine Learning A Unified Framework (Chapman & Hall/CRC Texts in Statistical Science)
Author: Richard Golden
Year: 2020
Pages: 525
Format: PDF
File size: 10.8 MB
Language: ENG
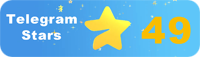
Year: 2020
Pages: 525
Format: PDF
File size: 10.8 MB
Language: ENG
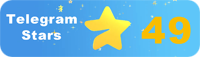
''
