
BOOKS - PROGRAMMING - Deep Learning for Engineers

Deep Learning for Engineers
Author: Tariq M. Arif, Md Adilur Rahim
Year: 2024
Pages: 170
Format: PDF
File size: 18.9 MB
Language: ENG
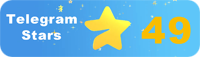
Year: 2024
Pages: 170
Format: PDF
File size: 18.9 MB
Language: ENG
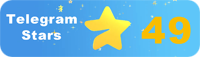
''
