
BOOKS - PROGRAMMING - Practical Machine Learning Innovations in Recommendation

Practical Machine Learning Innovations in Recommendation
Author: Ted Dunning, Ellen Friedman
Year: 2014
Pages: 56
Format: PDF
File size: 10,1 MB
Language: ENG
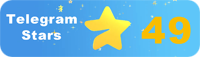
Year: 2014
Pages: 56
Format: PDF
File size: 10,1 MB
Language: ENG
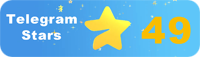
Book Description: Building a simple yet powerful recommendation system is much easier than you think! This report explains innovations that make machine learning practical for business production settings—and demonstrates how even a small-scale development team can design an effective large-scale recommendation system. The Plot: In a world where technology is rapidly evolving, it's crucial to understand the process of technological advancements and their impact on society. The book "Practical Machine Learning Innovations in Recommendation" highlights the need for a personal paradigm shift in perceiving the technological process of developing modern knowledge as the basis for humanity's survival and unity in a warring state. As the world becomes increasingly divided, this book offers a unique perspective on the role of machine learning in recommendation systems and its potential to unite people across cultures and borders. The story begins with the introduction of a small-scale development team tasked with building a recommendation system for their company. They soon realize that traditional methods are insufficient for their needs and turn to machine learning for solutions. Through a series of trials and errors, they discover the power of practical innovations in recommendation systems and how they can be applied to real-world scenarios. As the team delves deeper into the world of machine learning, they encounter challenges such as data bias, algorithmic fairness, and scalability issues. However, they also learn about the importance of collaboration and diversity in overcoming these obstacles.
Создание простой, но мощной системы рекомендаций намного проще, чем вы думаете! Этот отчет объясняет инновации, которые делают машинное обучение практичным для производственных параметров бизнеса, и демонстрирует, как даже небольшая команда разработчиков может разработать эффективную крупномасштабную систему рекомендаций. В мире, где технологии быстро развиваются, крайне важно понимать процесс технологических достижений и их влияние на общество. В книге «Practical Machine arning Innovations in Recommendation» подчеркивается необходимость персональной смены парадигмы в восприятии технологического процесса развития современных знаний как основы выживания и единства человечества в воюющем государстве. По мере того, как мир становится все более разделенным, эта книга предлагает уникальный взгляд на роль машинного обучения в рекомендательных системах и его потенциал для объединения людей между культурами и границами. История начинается с представления небольшой команды разработчиков, которой поручено построить систему рекомендаций для своей компании. Вскоре они понимают, что традиционные методы недостаточны для их нужд, и обращаются за решениями к машинному обучению. С помощью серии испытаний и ошибок они обнаруживают силу практических инноваций в рекомендательных системах и то, как их можно применить к реальным сценариям. По мере того как команда углубляется в мир машинного обучения, они сталкиваются с такими проблемами, как смещение данных, алгоритмическая справедливость и проблемы масштабируемости. Тем не менее, они также узнают о важности сотрудничества и разнообразия в преодолении этих препятствий.
Créer un système de recommandations simple mais puissant est beaucoup plus facile que vous ne le pensez ! Ce rapport explique les innovations qui rendent l'apprentissage automatique pratique pour les paramètres de production de l'entreprise et montre comment même une petite équipe de développement peut développer un système efficace de recommandations à grande échelle. Dans un monde où la technologie évolue rapidement, il est essentiel de comprendre le processus d'évolution technologique et son impact sur la société. livre « Practical Machine Arning Innovations in Recommandation » souligne la nécessité d'un changement de paradigme personnel dans la perception du processus technologique du développement des connaissances modernes comme base de la survie et de l'unité de l'humanité dans un État en guerre. Au fur et à mesure que le monde devient de plus en plus divisé, ce livre offre une vision unique du rôle de l'apprentissage automatique dans les systèmes de recommandation et de son potentiel pour rassembler les gens entre les cultures et les frontières. L'histoire commence par une petite équipe de développement chargée de construire un système de recommandations pour son entreprise. Ils se rendent bientôt compte que les méthodes traditionnelles ne sont pas suffisantes pour répondre à leurs besoins et cherchent des solutions à l'apprentissage automatique. Avec une série de tests et d'erreurs, ils découvrent la force de l'innovation pratique dans les systèmes de recommandation et comment ils peuvent être appliqués à des scénarios réels. Alors que l'équipe s'enfonce dans le monde de l'apprentissage automatique, elle est confrontée à des défis tels que le déplacement des données, l'équité algorithmique et les problèmes d'évolutivité. Cependant, ils apprennent aussi l'importance de la coopération et de la diversité pour surmonter ces obstacles.
¡Crear un sistema de recomendaciones simple pero potente es mucho más fácil de lo que crees! Este informe explica las innovaciones que hacen que el aprendizaje automático sea práctico para los parámetros de producción de un negocio, y demuestra cómo incluso un pequeño equipo de desarrollo puede desarrollar un sistema eficaz de recomendaciones a gran escala. En un mundo en el que la tecnología evoluciona rápidamente, es fundamental comprender el proceso de los avances tecnológicos y su impacto en la sociedad. libro Practical Machine Arning Innovations in Recommendation subraya la necesidad de un cambio de paradigma personal en la percepción del proceso tecnológico del desarrollo del conocimiento moderno como base de la supervivencia y unidad de la humanidad en un Estado en guerra. A medida que el mundo se divide cada vez más, este libro ofrece una visión única del papel del aprendizaje automático en los sistemas de recomendación y su potencial para unir a las personas entre culturas y fronteras. La historia comienza con la presentación de un pequeño equipo de desarrollo encargado de construir un sistema de recomendaciones para su empresa. Pronto se dan cuenta de que los métodos tradicionales no son suficientes para sus necesidades y recurren al aprendizaje automático para tomar decisiones. A través de una serie de pruebas y errores, descubren el poder de la innovación práctica en los sistemas de recomendación y cómo se pueden aplicar a escenarios reales. A medida que el equipo se adentra en el mundo del aprendizaje automático, se enfrentan a problemas como el desplazamiento de datos, la equidad algorítmica y los problemas de escalabilidad. n embargo, también aprenden la importancia de la cooperación y la diversidad para superar estos obstáculos.
Criar um sistema simples, mas poderoso de recomendações é muito mais fácil do que você pensa! Este relatório explica a inovação que torna o aprendizado de máquinas prático para os parâmetros de produção do negócio, e demonstra como até uma pequena equipe de desenvolvedores pode desenvolver um sistema de recomendação eficaz em larga escala. Num mundo em que a tecnologia evolui rapidamente, é fundamental compreender o processo de avanços tecnológicos e seus efeitos na sociedade. O livro «Pratical Machine Arning Inovations in Recordation» enfatiza a necessidade de uma mudança pessoal de paradigma na percepção do processo tecnológico de desenvolvimento do conhecimento moderno como base para a sobrevivência e unidade da humanidade num estado em guerra. À medida que o mundo se torna cada vez mais dividido, este livro oferece uma visão única do papel do aprendizado de máquinas nos sistemas de recomendação e do seu potencial para unir as pessoas entre culturas e fronteiras. A história começa com a apresentação de uma pequena equipe de desenvolvimento encarregada de construir um sistema de recomendação para a sua empresa. Logo percebem que os métodos tradicionais são insuficientes para as suas necessidades e procuram soluções para o aprendizado de máquinas. Através de uma série de testes e erros, eles revelam o poder da inovação prática em sistemas de recomendação e como eles podem ser aplicados a cenários reais. À medida que a equipe se aprofunda para o mundo do aprendizado de máquinas, eles enfrentam problemas como deslocamento de dados, justiça algoritmica e problemas de escalabilidade. No entanto, eles também aprendem sobre a importância da cooperação e da diversidade para superar esses obstáculos.
Das Erstellen eines einfachen, aber leistungsstarken Empfehlungssystems ist viel einfacher als e denken! Dieser Bericht erläutert die Innovationen, die maschinelles rnen für die Produktionsparameter eines Unternehmens praktisch machen, und zeigt, wie selbst ein kleines Entwicklungsteam ein effektives, groß angelegtes Empfehlungssystem entwickeln kann. In einer Welt, in der sich die Technologie schnell weiterentwickelt, ist es entscheidend, den Prozess des technologischen Fortschritts und seine Auswirkungen auf die Gesellschaft zu verstehen. Das Buch „Practical Machine arning Innovations in Recommendation“ betont die Notwendigkeit eines persönlichen Paradigmenwechsels in der Wahrnehmung des technologischen Prozesses der Entwicklung des modernen Wissens als Grundlage für das Überleben und die Einheit der Menschheit in einem kriegführenden Staat. Da die Welt zunehmend gespalten wird, bietet dieses Buch einen einzigartigen Einblick in die Rolle des maschinellen rnens in Empfehlungssystemen und sein Potenzial, Menschen über Kulturen und Grenzen hinweg zusammenzubringen. Die Geschichte beginnt mit der Vorstellung eines kleinen Entwicklungsteams, das mit dem Aufbau eines Empfehlungssystems für sein Unternehmen beauftragt ist. e erkennen bald, dass traditionelle Methoden für ihre Bedürfnisse nicht ausreichen, und suchen nach Lösungen für maschinelles rnen. Mit einer Reihe von Tests und Fehlern entdecken sie die Kraft praktischer Innovationen in Empfehlungssystemen und wie sie auf reale Szenarien angewendet werden können. Während das Team tiefer in die Welt des maschinellen rnens eintaucht, stehen sie vor Herausforderungen wie Datenverschiebungen, algorithmischer Fairness und Skalierbarkeitsproblemen. e lernen jedoch auch die Bedeutung von Zusammenarbeit und Vielfalt bei der Überwindung dieser Hindernisse kennen.
''
Basit ama güçlü bir öneri sistemi oluşturmak düşündüğünüzden çok daha kolay! Bu rapor, makine öğrenimini bir işletmenin üretim parametreleri için pratik kılan yenilikleri açıklamakta ve küçük bir geliştirme ekibinin bile etkili, büyük ölçekli bir öneri sistemi geliştirebileceğini göstermektedir. Teknolojinin hızla ilerlediği bir dünyada, teknolojik ilerlemelerin sürecini ve toplum üzerindeki etkilerini anlamak çok önemlidir. "Tavsiye Edilen Pratik Makine Öğrenimi Yenilikleri" kitabı, modern bilginin gelişiminin teknolojik sürecinin, savaşan bir durumda insanlığın hayatta kalması ve birliği için temel olarak algılanmasında kişisel bir paradigma değişimine duyulan ihtiyacı vurgulamaktadır. Dünya giderek daha fazla bölündükçe, bu kitap tavsiye sistemlerinde makine öğreniminin rolü ve insanları kültürler ve sınırlar arasında bağlama potansiyeli hakkında benzersiz bir bakış açısı sunuyor. Hikaye, şirketleri için bir öneri sistemi oluşturmakla görevli küçük bir geliştirme ekibinin tanıtımıyla başlar. Yakında geleneksel yöntemlerin ihtiyaçları için yetersiz olduğunu fark ederler ve çözümler için makine öğrenimine yönelirler. Bir dizi test ve hata sayesinde, öneri sistemlerinde pratik inovasyonun gücünü ve gerçek dünya senaryolarına nasıl uygulanabileceklerini keşfederler. Ekip makine öğrenimi dünyasına derinlemesine baktıkça, veri yanlılığı, algoritmik adalet ve ölçeklenebilirlik sorunları gibi zorluklarla karşı karşıya kalıyorlar. Bununla birlikte, bu engelleri aşmada işbirliğinin ve çeşitliliğin önemini de öğreniyorlar.
إنشاء نظام توصية بسيط وقوي أسهل بكثير مما تعتقد! يشرح هذا التقرير الابتكارات التي تجعل التعلم الآلي عمليًا لمعايير الإنتاج للشركة، ويوضح كيف يمكن لفريق التطوير الصغير تطوير نظام توصيات فعال واسع النطاق. في عالم تتقدم فيه التكنولوجيا بسرعة، من الأهمية بمكان فهم عملية التقدم التكنولوجي وتأثيرها على المجتمع. يؤكد كتاب «ابتكارات التعلم الآلي العملية في التوصية» على الحاجة إلى نقلة نوعية شخصية في تصور العملية التكنولوجية لتطور المعرفة الحديثة كأساس لبقاء البشرية ووحدتها في حالة حرب. مع تزايد انقسام العالم، يقدم هذا الكتاب منظورًا فريدًا لدور التعلم الآلي في أنظمة التوصيات وإمكاناته لربط الناس عبر الثقافات والحدود. تبدأ القصة بإدخال فريق تطوير صغير مكلف ببناء نظام توصية لشركتهم. سرعان ما يدركون أن الأساليب التقليدية غير كافية لاحتياجاتهم ويتجهون إلى التعلم الآلي للحصول على حلول. من خلال سلسلة من الاختبارات والأخطاء، يكتشفون قوة الابتكار العملي في أنظمة التوصيات وكيف يمكن تطبيقها على سيناريوهات العالم الحقيقي. بينما يتعمق الفريق في عالم التعلم الآلي، يواجهون تحديات مثل تحيز البيانات والعدالة الخوارزمية وقضايا قابلية التوسع. ومع ذلك، فهم يتعلمون أيضًا أهمية التعاون والتنوع في التغلب على هذه الحواجز.
