
BOOKS - Supervised and Unsupervised Learning for Data Science (Unsupervised and Semi-...

Supervised and Unsupervised Learning for Data Science (Unsupervised and Semi-Supervised Learning)
Author: Michael W. Berry
Year: September 4, 2019
Format: PDF
File size: PDF 16 MB
Language: English
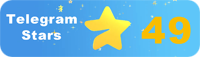
Year: September 4, 2019
Format: PDF
File size: PDF 16 MB
Language: English
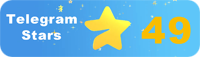
Supervised and Unsupervised Learning for Data Science: A Comprehensive Guide to Modern Knowledge Development In today's rapidly evolving technological landscape, it is crucial for individuals and societies to understand the process of technology development and its impact on modern knowledge. As we move forward in this digital age, it becomes increasingly important to develop a personal paradigm for perceiving the technological process of developing modern knowledge. This paradigm serves as the basis for the survival of humanity and the unification of people in a warring state. Supervised and Unsupervised Learning for Data Science: A Comprehensive Guide to Modern Knowledge Development provides a comprehensive overview of the state-of-the-art learning algorithms, including semi-supervised methods, to provide a broad scope of clustering and classification solutions for big data applications. The book is organized into eight chapters that cover discretization feature extraction and selection, classification, clustering, topic modeling, graph analysis, and applications. Each chapter offers a detailed description of the theoretical models of learning, along with practical case studies and best practices. The volume serves as an essential reference for practitioners and graduate students who seek to explore current and future research in unsupervised and semi-supervised learning.
Контролируемое и неконтролируемое обучение для науки о данных: всеобъемлющее руководство по развитию современных знаний В современном быстро развивающемся технологическом ландшафте для отдельных лиц и обществ крайне важно понимать процесс развития технологий и его влияние на современные знания. По мере продвижения вперед в эту цифровую эпоху становится все более важной разработка личностной парадигмы восприятия технологического процесса развития современных знаний. Эта парадигма служит основой для выживания человечества и объединения людей в воюющем государстве. «Контролируемое и неконтролируемое обучение для науки о данных: всеобъемлющее руководство по современному развитию знаний» содержит всесторонний обзор современных алгоритмов обучения, включая полууправляемые методы, для предоставления широкого спектра решений по кластеризации и классификации для приложений больших данных. Книга состоит из восьми глав, которые охватывают извлечение и выбор элементов дискретизации, классификацию, кластеризацию, тематическое моделирование, анализ графов и приложения. Каждая глава предлагает подробное описание теоретических моделей обучения, а также практические примеры и лучшие практики. Том служит важным справочным материалом для практиков и аспирантов, которые стремятся исследовать текущие и будущие исследования в области неконтролируемого и полу-контролируемого обучения.
Apprentissage contrôlé et non contrôlé pour la science des données : un guide complet pour le développement des connaissances modernes Dans le paysage technologique moderne en évolution rapide pour les individus et les sociétés, il est essentiel de comprendre le processus de développement technologique et son impact sur les connaissances modernes. À mesure que nous progressons dans cette ère numérique, il devient de plus en plus important de développer un paradigme personnel pour percevoir le processus technologique du développement des connaissances modernes. Ce paradigme sert de base à la survie de l'humanité et à l'unification des hommes dans un État en guerre. « L'apprentissage supervisé et non supervisé pour la science des données : un guide complet sur le développement des connaissances modernes » fournit un aperçu complet des algorithmes d'apprentissage modernes, y compris des méthodes semi-contrôlées, pour fournir un large éventail de solutions de regroupement et de classification pour les applications Big Data. livre se compose de huit chapitres qui traitent de l'extraction et du choix des éléments d'échantillonnage, de la classification, du regroupement, de la modélisation thématique, de l'analyse des graphiques et des applications. Chaque chapitre propose une description détaillée des modèles théoriques d'apprentissage, ainsi que des exemples pratiques et des meilleures pratiques. Tom est une référence importante pour les praticiens et les étudiants des cycles supérieures qui cherchent à explorer la recherche actuelle et future dans le domaine de l'apprentissage non contrôlé et semi-contrôlé.
Aprendizaje controlado e incontrolado para la ciencia de datos: una guía integral para el desarrollo del conocimiento moderno En el panorama tecnológico en rápida evolución actual, es fundamental que las personas y las sociedades comprendan el proceso de desarrollo de la tecnología y su impacto en el conocimiento moderno. A medida que avanzamos en esta era digital, es cada vez más importante desarrollar el paradigma personal de la percepción del proceso tecnológico del desarrollo del conocimiento moderno. Este paradigma sirve de base para la supervivencia de la humanidad y la unificación de los seres humanos en un Estado en guerra. «Aprendizaje controlado e incontrolado para la ciencia de datos: una guía integral para el desarrollo moderno del conocimiento» ofrece una visión general completa de los algoritmos de aprendizaje actuales, incluidos los métodos semi-administrados, para proporcionar una amplia gama de soluciones de clustering y clasificación para aplicaciones de big data. libro consta de ocho capítulos que abarcan la extracción y selección de elementos de muestreo, clasificación, clusterización, modelado temático, análisis de grafos y aplicaciones. Cada capítulo ofrece una descripción detallada de los modelos teóricos de aprendizaje, así como ejemplos prácticos y mejores prácticas. volumen sirve como un importante material de referencia para los practicantes y estudiantes de posgrado que buscan investigar la investigación actual y futura en el campo del aprendizaje descontrolado y semi-controlado.
Formação controlada e descontrolada para a ciência dos dados: guia abrangente para o desenvolvimento do conhecimento moderno No panorama tecnológico em desenvolvimento moderno, para indivíduos e sociedades, é fundamental compreender o processo de desenvolvimento da tecnologia e seus efeitos no conhecimento moderno. À medida que avançamos nesta era digital, é cada vez mais importante desenvolver um paradigma pessoal de percepção do processo tecnológico para o desenvolvimento do conhecimento moderno. Este paradigma serve de base para a sobrevivência da humanidade e para a união das pessoas num estado em guerra. «Treinamento supervisionado e descontrolado para a ciência de dados: guia abrangente para o desenvolvimento moderno do conhecimento» oferece uma revisão completa dos algoritmos modernos de aprendizagem, incluindo técnicas semigerenciadas, para fornecer uma ampla gama de soluções de clusterização e classificação para aplicações de big data. O livro é composto por oito capítulos que abrangem a extração e seleção de elementos de amostragem, classificação, clusterização, simulação temática, análise de gráficos e aplicativos. Cada capítulo oferece uma descrição detalhada dos modelos teóricos de aprendizagem, bem como exemplos práticos e melhores práticas. O volume é um importante material de referência para praticantes e estudantes de pós-graduação que procuram explorar estudos atuais e futuros sobre o ensino descontrolado e semi-controlado.
Formazione controllata e incontrollata per la scienza dei dati: una guida completa allo sviluppo delle conoscenze moderne In un panorama tecnologico in continua evoluzione per individui e società, è fondamentale comprendere il processo di sviluppo della tecnologia e il suo impatto sulle conoscenze moderne. Mentre progredisce in questa era digitale, diventa sempre più importante sviluppare un paradigma di percezione personale del processo tecnologico per lo sviluppo delle conoscenze moderne. Questo paradigma è la base per la sopravvivenza dell'umanità e per l'unione delle persone in uno stato in guerra. «Formazione controllata e non controllata per la scienza dei dati: una guida completa per lo sviluppo moderno delle conoscenze» fornisce una panoramica completa degli algoritmi di apprendimento avanzati, inclusi i metodi semigestiti, per fornire una vasta gamma di soluzioni di clustering e classificazione per le applicazioni di grandi dimensioni. Il libro è composto da otto capitoli che comprendono l'estrazione e la selezione di elementi di discrezionalizzazione, la classificazione, il clustering, la modellazione tematica, l'analisi di grafici e applicazioni. Ogni capitolo offre una descrizione dettagliata dei modelli teorici di apprendimento, nonché esempi pratici e migliori pratiche. Il volume è un importante materiale di riferimento per gli studiosi e i laureati che cercano di esplorare la ricerca attuale e futura nel campo dell'apprendimento incontrollato e semi-controllato.
Kontrolliertes und unkontrolliertes rnen für die Datenwissenschaft: Ein umfassender itfaden für die Entwicklung von aktuellem Wissen In der heutigen schnelllebigen Technologielandschaft ist es für Einzelpersonen und Gesellschaften von entscheidender Bedeutung, den technologischen Entwicklungsprozess und seine Auswirkungen auf das aktuelle Wissen zu verstehen. Mit dem Fortschritt in diesem digitalen Zeitalter wird es immer wichtiger, ein persönliches Paradigma für die Wahrnehmung des technologischen Prozesses der Entwicklung des modernen Wissens zu entwickeln. Dieses Paradigma dient als Grundlage für das Überleben der Menschheit und die Vereinigung der Menschen in einem kriegführenden Staat. „Controlled and Uncontrolled arning for Data Science: A Comprehensive Guide to Contemporary Knowledge Development“ bietet einen umfassenden Überblick über moderne rnalgorithmen einschließlich semi-kontrollierter Methoden, um ein breites Spektrum an Clustering- und Klassifizierungslösungen für Big-Data-Anwendungen bereitzustellen. Das Buch besteht aus acht Kapiteln, die die Extraktion und Auswahl von Diskretisierungselementen, Klassifikation, Clustering, thematische Modellierung, Graphenanalyse und Anwendungen abdecken. Jedes Kapitel bietet eine detaillierte Beschreibung der theoretischen rnmodelle sowie Praxisbeispiele und Best Practices. Der Band dient als wichtiges Referenzmaterial für Praktiker und Doktoranden, die aktuelle und zukünftige Forschung im Bereich des unkontrollierten und semi-kontrollierten rnens erforschen möchten.
Kontrolowane i niekontrolowane uczenie się na rzecz nauki o danych: Kompleksowy przewodnik po rozwoju nowoczesnej wiedzy W dzisiejszym szybko rozwijającym się krajobrazie technologicznym kluczowe znaczenie dla osób i społeczeństw ma zrozumienie procesu rozwoju technologii i jej wpływu na nowoczesną wiedzę. W erze cyfrowej coraz ważniejsze staje się rozwijanie osobistego paradygmatu postrzegania technologicznego procesu rozwoju nowoczesnej wiedzy. Paradygmat ten służy jako podstawa do przetrwania ludzkości i zjednoczenia ludzi w stanie wojennym. „Controlled and Unservised arning for Data Science: A Comprehensive Guide to Modern Knowledge Development” zapewnia kompleksowy przegląd nowoczesnych algorytmów uczenia się, w tym metod półprzewodnikowych, aby zapewnić szeroki zakres rozwiązań klastrujących i klasyfikacyjnych dla zastosowań dużych danych. Książka składa się z ośmiu rozdziałów, które obejmują pobieranie próbek i selekcję, klasyfikację, klastrowanie, modelowanie tematyczne, analizę wykresów i aplikacje. Każdy rozdział zawiera szczegółowy opis modeli uczenia się teoretycznego, a także praktyczne przykłady i najlepsze praktyki. Tom służy jako ważny punkt odniesienia dla praktyków i absolwentów, którzy starają się badać bieżące i przyszłe badania w niekontrolowanym i częściowo nadzorowanym kształceniu.
למידה מבוקרת ובלתי מבוקרת למדעי המידע: מדריך מקיף לקידום הידע המודרני בנוף הטכנולוגי המתפתח במהירות, הוא קריטי עבור יחידים וחברות להבין את תהליך התקדמות הטכנולוגיה ואת השפעתה על הידע המודרני. ככל שאנו מתקדמים בעידן הדיגיטלי הזה, יותר ויותר חשוב לפתח פרדיגמה אישית לתפיסה של התהליך הטכנולוגי של התפתחות הידע המודרני. פרדיגמה זו משמשת בסיס להישרדות האנושות ולאיחוד של אנשים במדינה לוחמת. מדריך מקיף לפיתוח ידע מודרני (Control and Unsupervised arning for Data Science: A Complicative Guide to Modern Knowledge Development) מספק סקירה מקיפה של אלגוריתמי למידה מודרניים, כולל שיטות מונחות למחצה, על מנת לספק מגוון רחב של פתרונות סיקור. הספר מורכב משמונה פרקים המכסים דגימות מיצוי ובחירה, סיווג, קיבוצים, מודלים ענייניים, ניתוח גרפים ויישומים. כל פרק מציג תיאור מפורט של מודלים ללמידה תיאורטית, כמו גם דוגמאות מעשיות ומנהגים טובים יותר. תום משמש כהתייחסות חשובה לעוסקים ולסטודנטים לתואר שני המבקשים לחקור מחקר עכשווי ועתידי בלמידה בלתי מבוקרת ומפוקחת למחצה.''
Veri Bilimi için Kontrollü ve Kontrolsüz Öğrenme: Modern Bilgiyi Geliştirmek İçin Kapsamlı Bir Rehber Günümüzün hızla gelişen teknolojik ortamında, bireylerin ve toplumların ilerleyen teknoloji sürecini ve modern bilgi üzerindeki etkisini anlamaları kritik öneme sahiptir. Bu dijital çağda ilerledikçe, modern bilginin gelişiminin teknolojik sürecinin algılanması için kişisel bir paradigma geliştirmek giderek daha önemli hale geliyor. Bu paradigma, insanlığın hayatta kalması ve insanların savaşan bir durumda birleşmesi için temel teşkil eder. "Veri Bilimi için Kontrollü ve Denetimsiz Öğrenme: Modern Bilgi Geliştirme için Kapsamlı Bir Kılavuz", büyük veri uygulamaları için çok çeşitli kümeleme ve sınıflandırma çözümleri sağlamak için yarı güdümlü yöntemler de dahil olmak üzere modern öğrenme algoritmalarına kapsamlı bir genel bakış sunar. Kitap örnekleme çıkarma ve seçimi, sınıflandırma, kümeleme, tematik modelleme, grafik analizi ve uygulamaları kapsayan sekiz bölümden oluşmaktadır. Her bölüm, teorik öğrenme modellerinin yanı sıra pratik örnekler ve en iyi uygulamaların ayrıntılı bir açıklamasını sunar. Tom, kontrolsüz ve yarı denetimli öğrenmede mevcut ve gelecekteki araştırmaları araştırmak isteyen uygulayıcılar ve lisansüstü öğrenciler için önemli bir referans görevi görür.
التعلم الخاضع للرقابة وغير المنضبط لعلوم البيانات: دليل شامل للنهوض بالمعرفة الحديثة في المشهد التكنولوجي سريع التطور اليوم، من المهم للأفراد والمجتمعات فهم عملية تطوير التكنولوجيا وتأثيرها على المعرفة الحديثة. بينما نمضي قدمًا في هذا العصر الرقمي، يصبح من المهم أكثر فأكثر تطوير نموذج شخصي لتصور العملية التكنولوجية لتطوير المعرفة الحديثة. هذا النموذج بمثابة أساس لبقاء البشرية وتوحيد الناس في دولة متحاربة. يوفر «التعلم الخاضع للرقابة وغير الخاضع للإشراف لعلوم البيانات: دليل شامل لتطوير المعرفة الحديثة» نظرة عامة شاملة على خوارزميات التعلم الحديثة، بما في ذلك الأساليب شبه الموجهة، لتوفير مجموعة واسعة من حلول التجميع والتصنيف لتطبيقات البيانات الضخمة. يتكون الكتاب من ثمانية فصول تغطي أخذ العينات والاختيار والتصنيف والتجميع والنمذجة المواضيعية وتحليل الرسم البياني والتطبيقات. ويقدم كل فصل وصفا مفصلا لنماذج التعلم النظري، فضلا عن أمثلة عملية وأفضل الممارسات. يعمل توم كمرجع مهم للممارسين وطلاب الدراسات العليا الذين يسعون إلى البحث في الأبحاث الحالية والمستقبلية في التعلم غير المنضبط وشبه الخاضع للإشراف.
데이터 과학에 대한 통제 및 통제되지 않은 학습: 현대 지식 발전을위한 포괄적 인 가이드 오늘날의 빠르게 진화하는 기술 환경에서 개인과 사회가 기술 발전 과정과 현대 지식에 미치는 영향을 이해하는 것이 중요합니다. 이 디지털 시대로 나아가면서 현대 지식 개발의 기술 프로세스에 대한 인식을위한 개인 패러다임을 개발하는 것이 점점 더 중요 해지고 있습니다. 이 패러다임은 인류의 생존과 전쟁 상태에있는 사람들의 통일의 기초가됩니다. "데이터 과학에 대한 통제 및 감독되지 않은 학습: 현대 지식 개발에 대한 포괄적 인 안내서" 는 빅 데이터 응용 프로그램을위한 광범위한 클러스터링 및 분류 솔루션을 제공하기 위해 세미 가이드 방법을 포함한 최신 학습 알고리즘에 대한 포괄적 인 개요소식을 제공급합니다. 이 책은 샘플링 추출 및 선택, 분류, 클러스터링, 주제 모델링, 그래프 분석 및 응용 프로그램을 다루는 8 개의 챕터로 구성됩니다. 각 장은 이론적 학습 모델에 대한 자세한 설명과 실용적인 예 및 모범 사례를 제공합니다. Tom은 통제되지 않은 반 감독 학습에서 현재와 미래의 연구를 연구하고자하는 실무자 및 대학원생에게 중요한 참고 자료입니다.
制御され、制御されていないデータサイエンスのための学習:現代の知識を進歩させるための包括的なガイド今日の急速に進化している技術風景では、個人や社会が技術を進歩させるプロセスと現代の知識への影響を理解することが重要です。このデジタル時代に進むにつれて、現代の知識の発展の技術プロセスの認識のための個人的なパラダイムを開発することがますます重要になります。このパラダイムは、人類の存続と戦争状態における人々の統一の基礎となる。「データサイエンスの制御と管理されていない学習:現代の知識開発の包括的ガイド」は、半導体法を含む現代の学習アルゴリズムの包括的な概要を提供し、ビッグデータアプリケーションに幅広いクラスタリングおよび分類ソリューションを提供します。本は、サンプリング抽出と選択、分類、クラスタリング、テーマ別モデリング、グラフ分析、およびアプリケーションをカバーする8つの章で構成されています。各章では、理論的学習モデルの詳細な説明と、実践的な例とベストプラクティスを提供しています。トムは、制御されていない半指導学習で現在および将来の研究を研究しようとする実務家や大学院生のための重要な参照として機能します。
數據科學的受控和無監督的學習:現代知識發展的綜合指南在當今快速發展的技術格局中,個人和社會必須了解技術的發展過程及其對現代知識的影響。隨著這個數字時代的發展,發展現代知識發展的技術過程的人格範式變得越來越重要。這種範式是人類生存和人類在交戰國團結的基礎。「數據科學的受控和非受控學習:現代知識發展的綜合指南」全面概述了現代學習算法,包括半受控技術,為大數據應用程序提供廣泛的聚類和分類解決方案。該書分為八章,涵蓋采樣元素的提取和選擇,分類,聚類,主題建模,圖形分析和應用。每章提供理論學習模式的詳細描述以及實例和最佳實踐。該卷為尋求探索非監督和半監督學習領域當前和未來的研究的從業人員和研究生提供了重要的參考資料。
