
BOOKS - Deep Learning for Video Understanding (Wireless Networks)

Deep Learning for Video Understanding (Wireless Networks)
Author: Zuxuan Wu
Year: August 2, 2024
Format: PDF
File size: PDF 33 MB
Language: English
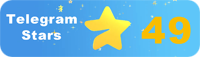
Year: August 2, 2024
Format: PDF
File size: PDF 33 MB
Language: English
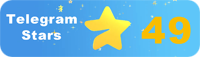
Book Deep Learning for Video Understanding Wireless Networks Book Overview: In this groundbreaking book, we delve into the realm of deep learning techniques for video understanding, exploring the latest advancements in machine learning pipelines and their applications in wireless networks. We begin by covering the fundamentals of deep learning notations and 2D and 3D Convolutional Neural Networks (CNNs) for spatial and temporal feature extraction. As we dive deeper into the world of video understanding, we introduce classical frameworks for image classification and elaborate on both image-based and clip-based 2D and 3D CNN networks for action recognition. Action Detection and Recognition: We explore various approaches to action detection and recognition, including sliding window proposals, single-stage, and two-stage methods, as well as spatial and temporal action localization. We also examine the importance of unsupervised feature learning and design better surrogate training tasks to learn video representations. This section is crucial in developing a personal paradigm for perceiving the technological process of developing modern knowledge, which is essential for the survival of humanity and the unity of people in a warring state. Video Captioning: Moving on to video captioning, we present language-based models and demonstrate how to perform sequence-to-sequence learning for video captioning.
Book Deep arning for Video Understanding Wireless Networks Обзор книги: В этой новаторской книге мы углубляемся в область методов глубокого обучения для понимания видео, исследуя последние достижения в области конвейеров машинного обучения и их приложений в беспроводных сетях. Мы начнем с рассмотрения основ нотации глубокого обучения и двумерных и трехмерных сверточных нейронных сетей (CNN) для пространственного и временного извлечения признаков. По мере того, как мы углубляемся в мир понимания видео, мы вводим классические основы для классификации изображений и разрабатываем как основанные на изображениях, так и основанные на клипах 2D и 3D сети CNN для распознавания действий. Обнаружение и распознавание действий: мы исследуем различные подходы к обнаружению и распознаванию действий, включая предложения скользящего окна, одноступенчатые и двухступенчатые методы, а также пространственную и временную локализацию действий. Мы также изучаем важность самостоятельного обучения признакам и разрабатываем более качественные суррогатные учебные задачи для изучения видеоизображений. Этот раздел имеет решающее значение в выработке личностной парадигмы восприятия технологического процесса развития современного знания, необходимого для выживания человечества и единства людей в воюющем государстве. Субтитры: Переходя к субтитрам, мы представляем языковые модели и демонстрируем, как выполнять обучение от последовательности к последовательности для субтитров.
Book Deep arning for Video Understanding Wireless Networks Aperçu du livre : Dans ce livre pionnier, nous approfondions le domaine des techniques d'apprentissage profond pour comprendre la vidéo en explorant les dernières avancées dans le domaine des pipelines d'apprentissage automatique et de leurs applications sur les réseaux sans fil. Nous commencerons par examiner les bases de la notation de l'apprentissage profond et des réseaux neuronaux convolutifs bidimensionnels et tridimensionnels (CNN) pour l'extraction spatiale et temporelle des traits. Au fur et à mesure que nous approfondissons le monde de la compréhension vidéo, nous introduisons des bases classiques pour la classification des images et développons à la fois des images et des clips 2D et 3D du réseau CNN pour reconnaître les actions. Détection et reconnaissance des actions : nous explorons différentes approches de détection et de reconnaissance des actions, y compris des propositions de fenêtre glissante, des méthodes en une étape et en deux étapes, ainsi que la localisation spatiale et temporelle des actions. Nous étudions également l'importance de l'auto-apprentissage des signes et développons de meilleures tâches d'apprentissage de substitution pour étudier les images vidéo. Cette section est cruciale dans l'élaboration du paradigme personnel de la perception du processus technologique du développement des connaissances modernes nécessaires à la survie de l'humanité et à l'unité des hommes dans un État en guerre. Sous-titres : En passant aux sous-titres, nous présentons des modèles linguistiques et montrons comment faire de l'apprentissage de séquence en séquence pour les sous-titres.
Book Deep arning for Video Understanding Wireless Networks Revisión del libro: En este libro pionero, profundizamos en el campo de las técnicas de aprendizaje profundo para la comprensión de videos, explorando los últimos avances en los transportadores de aprendizaje automático y sus aplicaciones en redes inalámbricas. Comenzaremos considerando los fundamentos de la notación de aprendizaje profundo y las redes neuronales convolucionales bidimensionales y tridimensionales (CNN) para la extracción espacial y temporal de rasgos. A medida que profundizamos en el mundo de la comprensión del vídeo, introducimos las bases clásicas para la clasificación de imágenes y desarrollamos tanto las basadas en imágenes como las basadas en clips de la red 2D y 3D de CNN para reconocer acciones. Detección y reconocimiento de acciones: investigamos diferentes enfoques para detectar y reconocer acciones, incluyendo sugerencias de ventana deslizante, métodos de una y dos etapas, y localización espacial y temporal de acciones. También estudiamos la importancia de la autoeducación de rasgos y desarrollamos tareas de formación sustitutas de mejor calidad para el estudio de imágenes de vídeo. Esta sección es crucial para generar el paradigma personal de percibir el proceso tecnológico de desarrollo del conocimiento moderno necesario para la supervivencia de la humanidad y la unidad de las personas en un Estado en guerra. Subtítulos: Al pasar a subtítulos, presentamos modelos de lenguaje y demostramos cómo realizar el aprendizaje de secuencia en secuencia para subtítulos.
Book Deep arning for Video Understanding Wireless Networks Review o Livro: Neste livro inovador, aprofundamo-nos na área de técnicas de aprendizagem profunda para compreender vídeos, explorando os avanços recentes nas linhas de montagem de aprendizagem de máquinas e suas aplicações nas redes sem fio. Vamos começar por considerar os fundamentos da notação de aprendizagem profunda e as redes neurais 3D e 3D (CNN) para extração espacial e temporária de sinais. À medida que nos aprofundamos no mundo da compreensão do vídeo, introduzimos as bases clássicas para classificar as imagens e desenvolvemos tanto as imagens baseadas nos clipes 2D e 3D da CNN para reconhecer as ações. Detecção e reconhecimento de ações: Investigamos várias abordagens para detecção e reconhecimento de ações, incluindo sugestões de janela deslizante, técnicas de um passo e dois passos, e localização espacial e temporária das ações. Também estudamos a importância da aprendizagem independente de sinais e desenvolvemos tarefas educacionais de aluguel de melhor qualidade para o estudo de imagens de vídeo. Esta seção é fundamental para a criação de um paradigma pessoal de percepção do processo tecnológico de desenvolvimento do conhecimento moderno, essencial para a sobrevivência da humanidade e a unidade das pessoas num Estado em guerra. gendas: Passando para legendas, apresentamos modelos linguísticos e mostramos como aprender de seqüência em sequência para legendas.
Book Deep arning for Video Understanding Wireless Networks Recensione del libro: In questo libro innovativo stiamo approfondendo le tecniche di apprendimento approfondito per comprendere i video, esplorando gli ultimi sviluppi nelle reti di montaggio e nelle loro applicazioni wireless. Inizieremo valutando le basi della notazione di apprendimento profondo e delle reti neurali compresse 3D (CNN) per l'estrazione spaziale e temporanea dei segni. Mentre stiamo approfondendo il mondo della comprensione dei video, stiamo introducendo le basi classiche per la classificazione delle immagini e sviluppando sia basate su immagini che basate sui video della CNN 2D e 3D per riconoscere le attività. Individuazione e riconoscimento delle attività: esploriamo diversi approcci per la rilevazione e il riconoscimento delle attività, inclusi i suggerimenti delle finestre scorrevole, i metodi a una e due fasi e la localizzazione temporale e spaziale delle attività. Studiamo anche l'importanza dell'apprendimento autonomo dei segni e sviluppiamo obiettivi di formazione surrogati di migliore qualità per lo studio delle immagini video. Questa sezione è fondamentale per sviluppare il paradigma personale della percezione del processo tecnologico di sviluppo della conoscenza moderna necessaria per la sopravvivenza dell'umanità e dell'unità delle persone in uno stato in guerra. Sottotitoli: Passando ai sottotitoli, presentiamo i modelli di lingua e mostriamo come imparare dalla sequenza alla sequenza per i sottotitoli.
Book Deep arning for Video Understanding Wireless Networks Buchbesprechung: In diesem bahnbrechenden Buch gehen wir tiefer in das Gebiet der Deep-arning-Techniken für das Verständnis von Videos ein und untersuchen die neuesten Fortschritte bei Machine-arning-Pipelines und deren Anwendungen in drahtlosen Netzwerken. Wir werden zunächst die Grundlagen der Deep-arning-Notation und der zwei- und dreidimensionalen Convolutional Neural Networks (CNNs) für die räumliche und zeitliche Extraktion von Merkmalen untersuchen. Während wir tiefer in die Welt des Videoverständnisses eintauchen, stellen wir klassische Grundlagen für die Klassifizierung von Bildern vor und entwickeln sowohl bildbasierte als auch clipbasierte 2D- und 3D-Netzwerke von CNN, um Aktionen zu erkennen. Aktionserkennung und -erkennung: Wir untersuchen verschiedene Ansätze zur Aktionserkennung und -erkennung, einschließlich gleitender Fenstervorschläge, einstufiger und zweistufiger Methoden sowie räumlicher und zeitlicher Lokalisierung von Aktionen. Wir untersuchen auch die Bedeutung des Selbsttrainings von Merkmalen und entwickeln bessere Surrogattrainingsaufgaben für das rnen von Videobildern. Dieser Abschnitt ist von entscheidender Bedeutung für die Entwicklung eines persönlichen Paradigmas der Wahrnehmung des technologischen Prozesses der Entwicklung des modernen Wissens, das für das Überleben der Menschheit und die Einheit der Menschen in einem kriegführenden Staat notwendig ist. Untertitel: Indem wir zu Untertiteln übergehen, stellen wir Sprachmodelle vor und zeigen, wie man das rnen von Sequenz zu Sequenz für Untertitel durchführt.
Book Deep arning for Video Understanding Wireless Networks Przegląd książki: W tej przełomowej książce zagłębiamy się w dziedzinie technik głębokiego uczenia się w celu zrozumienia wideo, odkrywając najnowsze osiągnięcia w zakresie rurociągów maszynowych i ich zastosowań w sieciach bezprzewodowych. Zaczynamy od rozważenia podstaw notacji głębokiego uczenia się oraz konwolucyjnych sieci neuronowych 2D i 3D (CNN) do ekstrakcji funkcji przestrzennych i czasowych. Ponieważ zagłębiamy się w świat zrozumienia wideo, wprowadzamy klasyczne ramy klasyfikacji obrazu i rozwijamy zarówno oparte na obrazie, jak i klipach sieci 2D i 3D CNN do rozpoznawania akcji. Wykrywanie i rozpoznawanie aktywności: Badamy różne podejścia do wykrywania i rozpoznawania aktywności, w tym sugestie okien przesuwnych, metody jednoetapowe i dwustopniowe oraz przestrzenne i czasowe lokalizowanie działań. Badamy również znaczenie samodzielnego uczenia się funkcji i rozwijania lepszych zadań w zakresie nauki zastępczej dla uczenia się obrazów wideo. Sekcja ta ma kluczowe znaczenie dla rozwoju osobistego paradygmatu postrzegania technologicznego procesu rozwoju nowoczesnej wiedzy, niezbędnego do przetrwania ludzkości i jedności ludzi w stanie wojującym. Napisy: Przechodząc do napisów, prezentujemy modele językowe i pokazujemy, jak wykonywać trening sekwencji do sekwencji napisów.
Book Deep arning for Video Understanding Wireless Networks Overview: בספר פורץ דרך זה אנו מתעמקים בתחום של שיטות למידה עמוקה להבנת וידאו על ידי חקר ההתקדמות האחרונה בצינורות למידת מכונה ויישומיהם ברשתות אלחוטיות. אנו מתחילים על ידי התחשבות ביסודות של סימון למידה עמוקה ורשתות עצביות קונבולוציוניות דו מימדיות ותלת מימדיות כשאנו מתעמקים יותר בעולם הבנת הווידאו, אנו מציגים מסגרות קלאסיות של סיווג תמונות ומפתחים גם רשתות 2D מבוססות תמונה וגם רשתות תלת ממד CNN לזיהוי פעולה. זיהוי פעילות והכרה: אנו חוקרים גישות שונות לזיהוי פעילות והכרה, כולל הזזת הצעות חלון, שיטות חד-שלביות ודו-שלבי, ומיקום מרחבי וזמני של פעילויות. אנחנו גם חוקרים את החשיבות של למידת תכונה בבימוי עצמי ומפתחים משימות למידה פונדקאיות טובות יותר ללמידת תמונות וידאו. סעיף זה חיוני בפיתוח פרדיגמה אישית לתפישת התהליך הטכנולוגי של התפתחות הידע המודרני, ההכרחי להישרדות האנושות ולאחדות בני האדם במדינה לוחמת. כתוביות: עוברים לכתוביות, אנו מציגים מודלים של שפה ומדגימים כיצד לבצע אימוני רצף לרצף לכתוביות.''
Kablosuz Ağları Anlamak İçin Derin Öğrenme Kitabı Kitaba Genel Bakış: Bu çığır açan kitapta, makine öğrenimi boru hatlarındaki en son gelişmeleri ve kablosuz ağlardaki uygulamalarını keşfederek videoyu anlamak için derin öğrenme teknikleri alanına giriyoruz. Mekansal ve zamansal özellik çıkarma için derin öğrenme gösteriminin ve 2D ve 3D evrişimli sinir ağlarının (CNN'ler) temellerini göz önünde bulundurarak başlıyoruz. Video anlama dünyasına daha derinlemesine baktığımızda, klasik resim sınıflandırma çerçevelerini tanıtıyoruz ve eylem tanıma için hem görüntü tabanlı hem de klip tabanlı 2D ve 3D CNN ağları geliştiriyoruz. Etkinlik algılama ve tanıma: Kayan pencere önerileri, tek aşamalı ve iki aşamalı yöntemler ve faaliyetlerin mekansal ve zamansal lokalizasyonu dahil olmak üzere etkinlik algılama ve tanıma için çeşitli yaklaşımları araştırıyoruz. Ayrıca, kendi kendini yöneten özellik öğreniminin önemini araştırıyor ve video görüntülerini öğrenmek için daha iyi vekil öğrenme görevleri geliştiriyoruz. Bu bölüm, modern bilginin teknolojik gelişim sürecinin algılanması için, insanlığın hayatta kalması ve savaşan bir devlette insanların birliği için gerekli olan kişisel bir paradigma geliştirmede çok önemlidir. Altyazılar: Altyazılara geçerek, dil modelleri sunuyoruz ve altyazılar için sıralı eğitimin nasıl yapılacağını gösteriyoruz.
كتاب التعلم العميق لفهم الفيديو نظرة عامة على كتاب الشبكات اللاسلكية: في هذا الكتاب الرائد، نتعمق في مجال تقنيات التعلم العميق لفهم الفيديو من خلال استكشاف أحدث التطورات في خطوط أنابيب التعلم الآلي وتطبيقاتها في الشبكات اللاسلكية. نبدأ بالنظر في أساسيات تدوين التعلم العميق والشبكات العصبية التلافيفية ثنائية الأبعاد وثلاثية الأبعاد (CNNs) لاستخراج الميزات المكانية والزمنية. بينما نتعمق أكثر في عالم فهم الفيديو، نقدم أطر تصنيف الصور الكلاسيكية ونطور شبكات CNN ثنائية وثلاثية الأبعاد القائمة على الصور والمقاطع للتعرف على الحركة. اكتشاف الأنشطة والتعرف عليها: نستكشف طرقا مختلفة لكشف الأنشطة والتعرف عليها، بما في ذلك اقتراحات النوافذ المنزلقة، وطرق الخطوة الواحدة والخطوتين، وتوطين الأنشطة مكانيا وزمانيا. نحن نستكشف أيضًا أهمية تعلم الميزات الموجهة ذاتيًا وتطوير مهام تعليمية بديلة أفضل لتعلم صور الفيديو. هذا القسم حاسم في تطوير نموذج شخصي لتصور العملية التكنولوجية لتطوير المعرفة الحديثة، الضرورية لبقاء البشرية ووحدة الناس في دولة متحاربة. الترجمة: بالانتقال إلى الترجمة، نقدم نماذج لغوية ونوضح كيفية أداء التدريب من تسلسل إلى تسلسل للترجمة.
무선 네트워크 도서 개요를 이해하기위한 비디오 딥 러닝을위한 도서 딥 러닝: 이 획기적인 책에서 우리는 머신 러닝 파이프 라인의 최신 발전과 무선 네트워크의 응용 프로그램을 탐색하여 비디오를 이해하기위한 딥 러닝 기술 분야를 탐구합니다. 우리는 공간 및 시간 특징 추출을위한 딥 러닝 표기법과 2D 및 3D 컨볼 루션 신경망 (CNN) 의 기본을 고려하여 시작합니다. 비디오 이해의 세계를 자세히 살펴보면서 고전적인 그림 분류 프레임 워크를 도입하고 행동 인식을 위해 이미지 기반 및 클립 기반 2D 및 3D CNN 네트워크를 개발하고 있습니다. 활동 감지 및 인식: 슬라이딩 창 제안, 단일 단계 및 2 단계 방법, 활동의 공간 및 시간적 위치화 등 활동 감지 및 인식에 대한 다양한 접근 방식을 탐색합니다. 또한 자체 감독 기능 학습의 중요성을 탐구하고 비디오 이미지 학습을위한 더 나은 대리 학습 작업을 개발하고 있습니다. 이 섹션은 인류의 생존과 전쟁 상태에있는 사람들의 통일성에 필요한 현대 지식의 기술 개발 과정에 대한 인식을위한 개인적인 패러다임을 개발하는 데 중요합니다. 자막: 자막으로 넘어 가면 언어 모델을 제시하고 자막에 대한 시퀀스 간 교육을 수행하는 방법을 보여줍니다.
Book Deep arning for Video Understanding Wireless Networks Book概要:この画期的な本では、機械学習パイプラインの最新の進歩とワイヤレスネットワークのアプリケーションを探索し、ビデオを理解するためのディープラーニング技術の分野を掘り下げます。まず、ディープラーニング表記の基礎と、空間的および時間的特徴抽出のための2Dおよび3D畳み込みニューラルネットワーク(CNN)を検討します。映像理解の世界を深く掘り下げながら、古典的な画像分類フレームワークを導入し、アクション認識のための画像ベースとクリップベースの2Dおよび3D CNNネットワークの両方を開発しています。アクティビティ検出と認識:スライディングウィンドウの提案、シングルステップと2ステップの方法、アクティビティの空間的および時間的局在化など、アクティビティの検出と認識に関するさまざまなアプローチを検討します。また、自主的な機能学習の重要性を探り、ビデオ画像を学習するためのより良いサロゲート学習タスクを開発しています。このセクションは、人類の生存と戦争状態における人々の団結のために必要な、現代の知識の発展の技術的プロセスの認識のための個人的なパラダイムを開発する上で重要です。字幕:字幕に移動して、言語モデルを提示し、字幕のシーケンストレーニングを実行する方法を示します。
Book Deep arning for Video Understanding Wireless Networks書評:在這本開創性的書中,我們深入研究深度學習技術領域以了解視頻,探索機器學習管道及其在無線網絡中的應用的最新進展。我們將首先考慮深度學習符號的基礎以及用於空間和時間特征提取的二維和三維卷積神經網絡(CNN)。當我們深入視頻理解世界時,我們介紹了圖像分類的經典基礎,並開發了基於圖像的CNN 2D和3D網絡剪輯來識別動作。活動檢測和識別:我們探索不同的活動檢測和識別方法,包括滑動窗口建議、單級和兩級方法以及活動的空間和時間定位。我們還研究了自我指導的特征學習的重要性,並開發了更高質量的替代教學任務來研究視頻圖像。本節對於發展人類生存和交戰國人民團結所需的現代知識的技術過程的個人範式至關重要。字幕:通過進入字幕,我們介紹了語言模型,並演示了如何對字幕進行序列到序列的學習。
