
BOOKS - Adversarial Machine Learning: Attack Surfaces, Defence Mechanisms, Learning T...

Adversarial Machine Learning: Attack Surfaces, Defence Mechanisms, Learning Theories in Artificial Intelligence
Author: Aneesh Sreevallabh Chivukula
Year: March 6, 2023
Format: PDF
File size: PDF 5.5 MB
Language: English
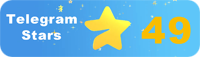
Year: March 6, 2023
Format: PDF
File size: PDF 5.5 MB
Language: English
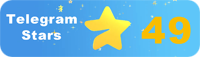
Book Adversarial Machine Learning Attack Surfaces Defence Mechanisms Learning Theories in Artificial Intelligence Introduction: Artificial intelligence (AI) has revolutionized numerous aspects of modern life, from healthcare to finance, transportation to education, and entertainment to communication. However, with great power comes great responsibility, and AI is no exception. As AI technology advances, it becomes increasingly vulnerable to security attacks from intelligent cyber adversaries. One critical challenge in deep learning is the susceptibility of deep learning networks to security threats, even from innocuous perturbations to the training data that can manipulate the behavior of deep networks in unintended ways. This book reviews the latest developments in adversarial attack technologies in computer vision, natural language processing, and cybersecurity, particularly regarding multidimensional textual and image data sequence data and temporal data. Adversarial Attacks in Deep Learning: Adversarial attacks refer to malicious manipulations of the input data designed to cause a model to misclassify or make incorrect predictions. These attacks exploit the brittleness of deep learning models, which are sensitive to small changes in the input data. Even slight perturbations can be used to deceive the model's predictions, leading to potential security risks. Adversarial attacks have become a significant concern for deep learning practitioners and researchers, as they can compromise the integrity of AI systems and undermine their reliability.
Название книги: Состязательное машинное обучение атакует поверхности Защитные механизмы Теории обучения в искусственном интеллекте Введение: Искусственный интеллект (ИИ) произвел революцию во многих аспектах современной жизни, от здравоохранения до финансов, транспорта до образования и развлечений до общения. Однако с большой силой приходит большая ответственность, и ИИ не исключение. По мере развития технологий искусственного интеллекта он становится все более уязвимым для атак безопасности со стороны интеллектуальных кибер-противников. Одной из важнейших проблем глубокого обучения является восприимчивость сетей глубокого обучения к угрозам безопасности, даже от безобидных возмущений до обучающих данных, которые могут непреднамеренно манипулировать поведением глубоких сетей. В этой книге рассматриваются последние разработки в технологиях состязательных атак в области компьютерного зрения, обработки естественного языка и кибербезопасности, особенно в отношении многомерных текстовых данных и данных последовательности данных изображения и временных данных. Adversarial Attacks in Deep arning: Под Adversarial attacks понимаются злонамеренные манипуляции с входными данными, призванные заставить модель неправильно классифицировать или делать неверные прогнозы. Эти атаки используют хрупкость моделей глубокого обучения, которые чувствительны к небольшим изменениям входных данных. Даже небольшие возмущения могут быть использованы для обмана прогнозов модели, что приведет к потенциальным рискам для безопасности. Состязательные атаки стали серьезной проблемой для практиков глубокого обучения и исследователей, поскольку они могут поставить под угрозу целостность систем ИИ и подорвать их надежность.
Titre du livre : L'apprentissage machine compétitif attaque les surfaces Mécanismes de défense Théorie de l'apprentissage en intelligence artificielle Introduction : L'intelligence artificielle (IA) a révolutionné de nombreux aspects de la vie moderne, de la santé à la finance, des transports à l'éducation et au divertissement à la communication. Cependant, une grande responsabilité vient avec une grande force, et l'IA n'est pas une exception. À mesure que les technologies de l'intelligence artificielle évoluent, elles deviennent de plus en plus vulnérables aux attaques de sécurité des cyber-adversaires intelligents. L'un des problèmes les plus importants du deep learning est la sensibilité des réseaux deep learning aux menaces à la sécurité, allant des perturbations inoffensives aux données d'apprentissage qui peuvent manipuler involontairement le comportement des réseaux deep. Ce livre examine les développements récents dans les technologies d'attaques concurrentielles dans les domaines de la vision par ordinateur, du traitement du langage naturel et de la cybersécurité, en particulier en ce qui concerne les données textuelles multidimensionnelles et les données de séquence de données d'image et de données temporelles. Attaques adverses dans Deep arning : les attaques adverses comprennent la manipulation malveillante des données d'entrée, conçue pour forcer le modèle à mal classer ou à faire des prévisions erronées. Ces attaques exploitent la fragilité des modèles d'apprentissage profond qui sont sensibles aux petites variations des données d'entrée. Même de petites perturbations peuvent être utilisées pour tromper les prévisions du modèle, ce qui entraînerait des risques potentiels pour la sécurité. s attaques concurrentielles sont devenues un défi majeur pour les praticiens et les chercheurs de l'apprentissage profond, car elles peuvent compromettre l'intégrité des systèmes d'IA et nuire à leur fiabilité.
Título del libro: aprendizaje automático contradictorio ataca las superficies Mecanismos protectores Teorías del aprendizaje en inteligencia artificial Introducción: La inteligencia artificial (IA) ha revolucionado muchos aspectos de la vida moderna, desde la salud hasta las finanzas, el transporte, la educación y el entretenimiento hasta la comunicación. n embargo, con mucha fuerza viene una gran responsabilidad, y la IA no es la excepción. A medida que las tecnologías de inteligencia artificial avanzan, se vuelve cada vez más vulnerable a los ataques de seguridad por parte de los cibernéticos inteligentes. Uno de los problemas más importantes del aprendizaje profundo es la susceptibilidad de las redes de aprendizaje profundo a amenazas de seguridad, incluso desde perturbaciones inofensivas hasta datos de aprendizaje que pueden manipular involuntariamente comportamientos de redes profundas. En este libro se examinan los últimos avances en tecnologías de ataque contradictorio en el campo de la visión informática, el procesamiento del lenguaje natural y la ciberseguridad, especialmente en relación con datos de texto multidimensionales y datos de secuencia de datos de imagen y datos temporales. Ataques adversarios en línea profunda: ataques adversarios se entienden como manipulaciones malintencionadas de datos de entrada diseñadas para hacer que el modelo clasifique incorrectamente o haga predicciones incorrectas. Estos ataques aprovechan la fragilidad de los modelos de aprendizaje profundo, que son sensibles a pequeños cambios en la entrada. Incluso pequeñas perturbaciones pueden ser usadas para engañar las predicciones del modelo, dando lugar a potenciales riesgos de seguridad. ataques adversarios se han convertido en un gran desafío para los profesionales del aprendizaje profundo y los investigadores, ya que pueden poner en peligro la integridad de los sistemas de IA y socavar su fiabilidad.
Título do livro: Aprendizagem de Máquinas Concorrentes ataca superfícies Mecanismos de Defesa da Teoria da Inteligência Artificial Introdução: Inteligência Artificial (IA) revolucionou muitos aspectos da vida moderna, desde saúde, finanças, transporte, educação e entretenimento até comunicação. No entanto, uma grande responsabilidade vem com grande força, e a IA não é exceção. À medida que as tecnologias de inteligência artificial avançam, torna-se cada vez mais vulnerável a ataques de segurança por parte de ciber-opositores inteligentes. Um dos maiores desafios do aprendizado profundo é a receptividade das redes de treinamento profundo às ameaças de segurança, mesmo desde perturbações inofensivas até dados de aprendizagem que podem, inadvertidamente, manipular o comportamento das redes profundas. Este livro aborda os últimos desenvolvimentos em tecnologias de competição de ataques de visão de computador, processamento de linguagem natural e segurança cibernética, especialmente em relação a dados de texto multidimensionais e seqüência de dados de imagem e dados temporários. Adversarial Attacks in Deep arning: Por Adversarial attacks, é compreendida a manipulação maliciosa de dados de entrada para forçar o modelo a classificar ou fazer previsões erradas. Estes ataques usam a fragilidade de modelos de aprendizado profundo que são sensíveis a pequenas alterações de dados de entrada. Mesmo pequenas perturbações podem ser usadas para enganar as previsões do modelo, levando a riscos potenciais para a segurança. Os ataques em competição tornaram-se um grande problema para os praticantes de treinamento profundo e pesquisadores, porque eles podem comprometer a integridade dos sistemas de IA e minar sua confiabilidade.
L'intelligenza artificiale (intelligenza artificiale) ha rivoluzionato molti aspetti della vita moderna, dalla salute alla finanza, ai trasporti all'educazione e all'intrattenimento. Ma con grande forza arriva una grande responsabilità, e l'IA non fa eccezione. Con l'evoluzione delle tecnologie di intelligenza artificiale, diventa sempre più vulnerabile agli attacchi di sicurezza da parte dei cyber-oppositori intelligenti. Uno dei problemi più importanti dell'apprendimento profondo è la sensibilità delle reti di apprendimento alle minacce per la sicurezza, anche dalle perturbazioni innocue ai dati di apprendimento, che possono manipolare involontariamente il comportamento delle reti profonde. In questo libro vengono illustrati gli ultimi sviluppi delle tecnologie per gli attacchi competitivi in termini di visione informatica, gestione del linguaggio naturale e cybersecurity, in particolare per quanto riguarda i dati multi-dimensioni e la sequenza dei dati immagine e temporanei. Adversarial Attacks in Deep arning: Adversarial attacks è una manipolazione malevola dei dati di input destinata a costringere il modello a classificare o a fare previsioni errate. Questi attacchi sfruttano la fragilità dei modelli di apprendimento approfondito che sono sensibili a piccoli cambiamenti nei dati di input. Anche piccole perturbazioni possono essere usate per ingannare le previsioni del modello, causando potenziali rischi per la sicurezza. Gli attacchi competitivi sono diventati un problema serio per gli studiosi e i ricercatori, perché possono compromettere l'integrità dei sistemi di IA e compromettere la loro affidabilità.
Buchtitel: Kontradiktorisches maschinelles rnen greift Oberflächen an Abwehrmechanismen rntheorien in der Künstlichen Intelligenz Einleitung: Künstliche Intelligenz (KI) hat viele Aspekte des modernen bens revolutioniert, vom Gesundheitswesen über Finanzen, Transport über Bildung und Unterhaltung bis hin zur Kommunikation. Mit großer Kraft kommt jedoch eine große Verantwortung, und KI ist keine Ausnahme. Mit der Weiterentwicklung der KI-Technologie wird es zunehmend anfällig für cherheitsangriffe von intelligenten Cybergegnern. Eines der wichtigsten Probleme bei Deep arning ist die Anfälligkeit von Deep-arning-Netzwerken für cherheitsbedrohungen, auch von harmlosen Störungen bis hin zu Trainingsdaten, die das Verhalten von Deep Networks unbeabsichtigt manipulieren können. Dieses Buch untersucht die neuesten Entwicklungen in den Technologien der kontradiktorischen Angriffe in den Bereichen Computer Vision, natürliche Sprachverarbeitung und Cybersicherheit, insbesondere in Bezug auf mehrdimensionale Textdaten und Bilddaten und Zeitdatensequenzdaten. Adversarial Attacks in Deep arning: Unter Adversarial Attacks versteht man die böswillige Manipulation von Inputs, die ein Modell dazu bringen sollen, falsch zu klassifizieren oder falsche Vorhersagen zu treffen. Diese Angriffe nutzen die Fragilität von Deep-arning-Modellen aus, die empfindlich auf kleine Änderungen der Eingabedaten reagieren. Selbst kleine Störungen können verwendet werden, um Modellprognosen zu täuschen, was zu potenziellen cherheitsrisiken führt. Kontradiktorische Angriffe sind zu einem großen Problem für Deep-arning-Praktiker und Forscher geworden, da sie die Integrität von KI-Systemen gefährden und ihre Zuverlässigkeit untergraben können.
Tytuł książki: Adversarial machine learning attacks surfaces Mechanizmy obronne Teorie nauki w sztucznej inteligencji Wprowadzenie: Sztuczna inteligencja (AI) zrewolucjonizowała wiele aspektów współczesnego życia, od opieki zdrowotnej do finansowania, transportu do edukacji i rozrywki do komunikacji. Jednak z wielką mocą przychodzi wielka odpowiedzialność, a AI nie jest wyjątkiem. Wraz z rozwojem technologii sztucznej inteligencji staje się ona coraz bardziej narażona na ataki cybernetyczne ze strony inteligentnych przeciwników. Jednym z najważniejszych problemów głębokiego uczenia się jest podatność sieci głębokiego uczenia się na zagrożenia bezpieczeństwa, nawet od nieszkodliwych zakłóceń po dane szkoleniowe, które mogą nieumyślnie manipulować zachowaniem głębokich sieci. Książka ta analizuje najnowsze osiągnięcia w zakresie technologii ataków przeciwnych w wizji komputerowej, przetwarzaniu języka naturalnego i cyberbezpieczeństwie, w szczególności w odniesieniu do wielowymiarowych danych tekstowych oraz danych dotyczących sekwencji obrazu i danych czasowych. Ataki przeciwne w głębokim uczeniu się: Ataki przeciwne odnoszą się do złośliwej manipulacji danymi wejściowymi, które mają spowodować błędną klasyfikację modelu lub błędne przewidywania. Ataki te wykorzystują kruchość modeli głębokiego uczenia się, które są wrażliwe na małe zmiany danych wejściowych. Nawet małe perturbacje mogą być wykorzystywane do oszukiwania prognoz modelowych, co prowadzi do potencjalnych zagrożeń dla bezpieczeństwa. Ataki przeciwstawne stały się główną troską dla praktyków i badaczy głębokiego uczenia się, ponieważ mogą zagrozić integralności systemów grypy ptaków i podważyć ich niezawodność.
שם הספר: Adversarial machine learning attacks faces supports Theories of arning in Artifical Intelligence Intelligence: Artificial Intelligence (בינה מלאכותית) חוללה מהפכה בהיבטים רבים של החיים המודרניים. עם זאת, עם כוח רב מגיעה אחריות גדולה, ובינה מלאכותית אינה יוצאת מן הכלל. ככל שטכנולוגיות בינה מלאכותית מתפתחות, הן הופכות לפגיעות יותר ויותר להתקפות אבטחה מצד יריבי סייבר אינטליגנטיים. אחת הבעיות החשובות ביותר של למידה מעמיקה היא הרגישות של רשתות למידה מעמיקה לאיומים ביטחוניים, אפילו מהפרעות לא מזיקות ספר זה בוחן את ההתפתחויות האחרונות בטכנולוגיות מתקפות נגד בתחום הראייה הממוחשבת, עיבוד שפה טבעית ואבטחת סייבר, במיוחד ביחס לנתוני טקסט רב-ממדיים ותמונה ונתוני רצף נתונים זמניים. התקפות נגד בלמידה עמוקה (באנגלית: Adversarial Attacks in Deep arning: Adversarial Attacks) הן התקפות המתייחסות למניפולציה זדונית של נתוני קלט שנועדו לגרום למודל לסווג באופן שגוי או לבצע תחזיות שגויות. התקפות אלו מנצלות את השבריריות של מודלים ללמידה עמוקה אשר רגישים לשינויים קטנים בנתוני הקלט. אפילו הפרעות קטנות יכולות לשמש להטעיית תחזיות מודל, מה שמוביל לסיכונים בטיחותיים פוטנציאליים. התקפות נגד הפכו לדאגה גדולה לעוסקים בחקר הלמידה העמוקה, משום שהן עלולות לפגוע בשלמות מערכות הבינה המלאכותית ולערער את מהימנותן.''
Kitap başlığı: Düşmanca makine öğrenme saldırıları yüzeyler Savunma mekanizmaları Yapay zekada öğrenme teorileri Giriş: Yapay zeka (AI), sağlıktan finansa, ulaşımdan eğitime ve eğlenceden iletişime kadar modern yaşamın birçok alanında devrim yarattı. Bununla birlikte, büyük güçle büyük sorumluluk gelir ve AI istisna değildir. Yapay zeka teknolojileri geliştikçe, akıllı siber rakiplerin güvenlik saldırılarına karşı giderek daha savunmasız hale geliyor. Derin öğrenmenin en önemli sorunlarından biri, derin öğrenme ağlarının güvenlik tehditlerine karşı duyarlılığıdır, hatta zararsız bozukluklardan, derin ağların davranışını yanlışlıkla manipüle edebilecek eğitim verilerine kadar. Bu kitap, bilgisayar görüşü, doğal dil işleme ve siber güvenlik alanlarındaki düşmanca saldırı teknolojilerindeki son gelişmeleri, özellikle çok boyutlu metin verileri ve görüntü ve zamansal veri dizisi verileri açısından incelemektedir. Derin Öğrenmede Düşmanca Saldırılar: Düşmanca saldırılar, bir modelin yanlış sınıflandırılmasına veya yanlış tahminlerde bulunmasına neden olmak için tasarlanmış girdi verilerinin kötü amaçlı manipülasyonunu ifade eder. Bu saldırılar, giriş verilerindeki küçük değişikliklere duyarlı derin öğrenme modellerinin kırılganlığından yararlanır. Model tahminlerini aldatmak için küçük tedirginlikler bile kullanılabilir ve bu da potansiyel güvenlik risklerine yol açabilir. Düşmanca saldırılar, derin öğrenme uygulayıcıları ve araştırmacıları için büyük bir endişe haline gelmiştir, çünkü AI sistemlerinin bütünlüğünü tehlikeye atabilir ve güvenilirliklerini zayıflatabilir.
عنوان الكتاب: هجمات التعلم الآلي العدائية على الأسطح آليات الدفاع نظريات التعلم في الذكاء الاصطناعي المقدمة: أحدث الذكاء الاصطناعي (AI) ثورة في العديد من جوانب الحياة الحديثة، من الرعاية الصحية إلى التمويل والنقل إلى التعليم والترفيه إلى التواصل. ومع ذلك، مع القوة العظمى تأتي مسؤولية كبيرة، والذكاء الاصطناعي ليس استثناءً. مع تطور تقنيات الذكاء الاصطناعي، تصبح عرضة بشكل متزايد للهجمات الأمنية من خصوم الإنترنت الأذكياء. واحدة من أهم مشاكل التعلم العميق هي قابلية شبكات التعلم العميق للتهديدات الأمنية، حتى من الاضطرابات غير الضارة إلى بيانات التدريب التي يمكن أن تتلاعب عن غير قصد بسلوك الشبكات العميقة. يدرس هذا الكتاب التطورات الأخيرة في تقنيات الهجوم العدائي في الرؤية الحاسوبية ومعالجة اللغة الطبيعية والأمن السيبراني، لا سيما فيما يتعلق ببيانات النصوص متعددة الأبعاد وبيانات الصورة وتسلسل البيانات الزمنية. الهجمات العدائية في التعلم العميق: تشير الهجمات العدائية إلى التلاعب الخبيث ببيانات الإدخال المصممة للتسبب في سوء تصنيف النموذج أو إجراء تنبؤات غير صحيحة. تستغل هذه الهجمات هشاشة نماذج التعلم العميق الحساسة للتغيرات الصغيرة في بيانات المدخلات. حتى الاضطرابات الصغيرة يمكن استخدامها لخداع تنبؤات النماذج، مما يؤدي إلى مخاطر محتملة على السلامة. أصبحت الهجمات العدائية مصدر قلق كبير لممارسي التعلم العميق والباحثين لأنها يمكن أن تعرض سلامة أنظمة الذكاء الاصطناعي للخطر وتقوض موثوقيتها.
책 제목: 적대적 기계 학습 공격은 인공 지능 소개에서 학습의 방어 메커니즘 이론을 표면화합니다. 인공 지능 (AI) 은 의료에서 금융, 교통 및 엔터테인먼트, 의사 소통에 이르기까지 현대 생활의 여러 측면에 혁명을 일으켰습니다. 그러나 큰 힘으로 큰 책임이 따르며 AI도 예외는 아닙니다. 인공 지능 기술이 발전함에 따라 지능형 사이버 적의 보안 공격에 점점 더 취약 해집니다. 딥 러닝의 가장 중요한 문제 중 하나는 딥 러닝 네트워크가 무해한 장애에서부터 딥 네트워크의 동작을 실수로 조작 할 수있는 교육 데이터에 이르기까지 보안 위협에 대한 감수성입니다. 이 책은 특히 다차원 텍스트 데이터 및 이미지 및 시간 데이터 시퀀스 데이터와 관련하여 컴퓨터 비전, 자연어 처리 및 사이버 보안의 적대적 공격 기술의 최근 개발을 검토합니다. 딥 러닝의 적대적 공격: 적대적 공격은 모델이 잘못 분류하거나 잘못 예측하도록 설계된 입력 데이터의 악의적 인 조작을 말합니다. 이러한 공격은 입력 데이터의 작은 변화에 민감한 딥 러닝 모델의 취약성을 활용합니다. 작은 섭동조차도 모델 예측을 속이는 데 사용되어 잠재적 인 안전 위험을 초래할 수 있습니다. 적대적 공격은 AI 시스템의 무결성을 손상시키고 신뢰성을 손상시킬 수 있기 때문에 딥 러닝 실무자 및 연구원에게 주요 관심사가되었습니다.
Book title: Adversarial machine learning attack surfaces防衛メカニズム人工知能における学習の理論はじめに:人工知能(AI)は、医療から金融、交通、教育、娯楽、コミュニケーションまで、現代生活の多くの側面に革命をもたらしました。しかし、大きな力を持つことは大きな責任であり、AIも例外ではありません。人工知能技術が進化するにつれて、インテリジェントなサイバー攻撃からのセキュリティ攻撃に対してますます脆弱になります。ディープラーニングの最も重要な問題の1つは、ディープラーニングネットワークがセキュリティの脅威に敏感になることです。本書では、コンピュータビジョン、自然言語処理、サイバーセキュリティにおける敵対的攻撃技術の最近の進展、特に多次元テキストデータおよび画像および時間的データ配列データに関して考察する。ディープラーニングにおける敵対的攻撃:敵対的攻撃とは、モデルが誤って分類したり、誤った予測をしたりするように設計された入力データの悪意のある操作を指します。これらの攻撃は、入力データの小さな変化に敏感なディープラーニングモデルの脆弱性を利用します。小さな摂動であっても、モデルの予測を欺くために使用することができ、潜在的な安全上のリスクにつながります。敵対的攻撃は、AIシステムの完全性を損ない、信頼性を損なう可能性があるため、ディープラーニング実践者や研究者にとって大きな関心事となっています。
書名:對抗性機器學習攻擊表面防禦機制人工智能學習理論:人工智能(AI)徹底改變了現代生活的許多方面,從醫療保健到金融,運輸到教育和娛樂再到交流。但是,大量責任隨之而來,AI也不例外。隨著人工智能技術的發展,它越來越容易受到智能網絡對手的安全攻擊。深度學習最重要的問題之一是深度學習網絡容易受到安全威脅的影響,甚至從無害的幹擾到可能無意中操縱深度網絡行為的學習數據。本書探討了計算機視覺,自然語言處理和網絡安全領域的對抗性攻擊技術的最新發展,特別是在多維文本數據以及圖像和時間數據序列數據方面。深度學習中的Adversarial Attacks:Adversarial Attacks是指惡意輸入操作,旨在迫使模型錯誤分類或做出錯誤的預測。這些攻擊利用了對輸入小的變化敏感的深度學習模型的脆弱性。即使是小擾動也可以用來欺騙模型的預測,從而導致潛在的安全風險。對抗性攻擊已成為深度學習從業者和研究人員的主要問題,因為它們可能會損害AI系統的完整性並損害其可靠性。
