
BOOKS - Feature Engineering for Modern Machine Learning with Scikit-Learn Advanced Da...

Feature Engineering for Modern Machine Learning with Scikit-Learn Advanced Data Science and Practical Applications
Author: Cuantum Technologies
Year: November 6, 2024
Pages: 506
Format: PDF | EPUB
File size: 13.2 MB
Language: ENG
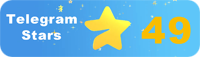
Year: November 6, 2024
Pages: 506
Format: PDF | EPUB
File size: 13.2 MB
Language: ENG
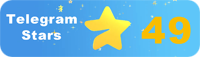
Book Description: Feature engineering is a critical component of machine learning that involves selecting and transforming variables from raw data into features that are suitable for modeling. This book provides a comprehensive guide to feature engineering for modern machine learning with scikit-learn, covering advanced data science and practical applications. The book begins by introducing the concept of feature engineering and its importance in machine learning, followed by an overview of the scikit-learn library and its capabilities. The next section covers the essential techniques for feature engineering, including data cleaning, normalization, feature scaling, and dimensionality reduction. The book then delves into more advanced topics such as feature selection, feature extraction, and domain adaptation. Finally, it discusses practical applications of feature engineering in various industries, including healthcare, finance, and marketing. The book's primary focus is on the practical aspects of feature engineering, providing readers with hands-on experience through exercises and case studies. It also includes interviews with industry experts to provide real-world insights and examples of feature engineering in action. By the end of this book, readers will have gained a deep understanding of feature engineering and be able to apply their knowledge to real-world problems. Book Outline: I. Introduction to Feature Engineering * Definition and Importance of Feature Engineering * Overview of Scikit-Learn Library II.
Feature engineering - критически важный компонент машинного обучения, который включает в себя выбор и преобразование переменных из необработанных данных в функции, подходящие для моделирования. Эта книга содержит исчерпывающее руководство по разработке функций для современного машинного обучения с scikit-learn, охватывающее передовые науки о данных и практические приложения. Книга начинается с введения концепции feature engineering и её важности в машинном обучении, после чего следует обзор библиотеки scikit-learn и её возможностей. В следующем разделе рассматриваются основные методы проектирования элементов, включая очистку данных, нормализацию, масштабирование элементов и уменьшение размерности. Затем книга углубляется в более продвинутые темы, такие как выбор признаков, извлечение признаков и адаптация доменов. Наконец, в нем обсуждаются практические применения feature engineering в различных отраслях, включая здравоохранение, финансы и маркетинг. Основное внимание в книге уделяется практическим аспектам feature engineering, предоставляя читателям практический опыт посредством упражнений и тематических исследований. Он также включает интервью с отраслевыми экспертами, чтобы предоставить реальную информацию и примеры функционального проектирования в действии. К концу этой книги читатели получат глубокое понимание инженерии функций и смогут применить свои знания к реальным проблемам. Структура книги: I. Введение в разработку функций * Определение и важность разработки функций * Обзор библиотеки Scikit-arn Library II.
''
