
BOOKS - PROGRAMMING - A Brief Introduction to Machine Learning for Engineers (Foundat...

A Brief Introduction to Machine Learning for Engineers (Foundations and Trends(r) in Signal Processing)
Author: Osvaldo Simeone
Year: 2019
Pages: 250
Format: PDF
File size: 10 MB
Language: ENG
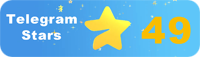
Year: 2019
Pages: 250
Format: PDF
File size: 10 MB
Language: ENG
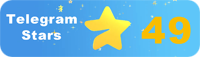
A Brief Introduction to Machine Learning for Engineers Foundations and Trends in Signal Processing Introduction: In today's fast-paced technological era, it is imperative for engineers to have a comprehensive understanding of machine learning, a field that has revolutionized the way we approach problems in various industries. With the ever-evolving nature of technology, it becomes increasingly crucial to stay updated on the latest advancements in the field of machine learning. As an engineer, it can be overwhelming to navigate through the vast amount of literature available on the subject. Therefore, A Brief Introduction to Machine Learning for Engineers is an indispensable resource for those seeking a foundational understanding of machine learning. This book serves as a starting point for engineers who are new to the field, providing a unified treatment of key concepts and principles, while also offering pointers to the literature for further study. Chapter 1: Probabilistic Models The first chapter delves into probabilistic models, which form the foundation of machine learning. It covers the basics of probability theory and introduces the reader to the concept of Bayes' theorem. The authors explain how these fundamental principles are applied in machine learning algorithms, enabling readers to understand the underlying logic of these techniques. The chapter concludes with a discussion on the importance of probability distributions in machine learning and their relevance to real-world applications.
Краткое введение в машинное обучение для инженеров Основы и тенденции в обработке сигналов Введение: В современную быстро развивающуюся технологическую эру инженерам необходимо иметь всестороннее понимание машинного обучения, области, которая произвела революцию в подходе к проблемам в различных отраслях. С постоянно развивающейся природой технологий становится все более важным постоянно быть в курсе последних достижений в области машинного обучения. Как инженеру может быть непосильно ориентироваться в огромном количестве литературы, доступной по этому вопросу. Поэтому «Краткое введение в машинное обучение для инженеров» является незаменимым ресурсом для тех, кто ищет фундаментальное понимание машинного обучения. Эта книга служит отправной точкой для инженеров, которые являются новичками в этой области, предоставляя единый подход к ключевым концепциям и принципам, а также предлагая ссылки на литературу для дальнейшего изучения. Глава 1: Вероятностные модели Первая глава углубляется в вероятностные модели, которые формируют основу машинного обучения. Она охватывает основы теории вероятностей и знакомит читателя с понятием теоремы Байеса. Авторы объясняют, как эти фундаментальные принципы применяются в алгоритмах машинного обучения, позволяя читателям понять основную логику этих методов. Глава завершается обсуждением важности вероятностных распределений в машинном обучении и их актуальности для реальных приложений.
Brève introduction au Machine arning pour ingénieurs s bases et les tendances du traitement du signal Introduction : Dans l'ère technologique moderne en évolution rapide, les ingénieurs doivent avoir une compréhension complète du Machine arning, un domaine qui a révolutionné l'approche des problèmes dans différentes industries. Avec l'évolution constante de la technologie, il devient de plus en plus important de se tenir au courant des dernières avancées dans le domaine de l'apprentissage automatique. En tant qu'ingénieur, il peut être insupportable de naviguer dans la grande quantité de littérature disponible sur cette question. Par conséquent, une « brève introduction à l'apprentissage automatique pour les ingénieurs » est une ressource indispensable pour ceux qui recherchent une compréhension fondamentale de l'apprentissage automatique. Ce livre sert de point de départ pour les ingénieurs qui sont nouveaux dans ce domaine, offrant une approche unifiée des concepts et des principes clés, ainsi que des liens vers la littérature pour une étude plus approfondie. Chapitre 1 : Modèles probabilistes premier chapitre explore les modèles probabilistes qui forment la base de l'apprentissage automatique. Il couvre les fondements de la théorie des probabilités et présente au lecteur la notion du théorème de Bayes. s auteurs expliquent comment ces principes fondamentaux sont appliqués dans les algorithmes d'apprentissage automatique, permettant aux lecteurs de comprendre la logique sous-jacente de ces méthodes. chapitre se termine par une discussion sur l'importance des distributions probabilistes dans l'apprentissage automatique et leur pertinence pour les applications réelles.
Breve introducción al aprendizaje automático para ingenieros Conceptos básicos y tendencias en el procesamiento de señales Introducción: En una era tecnológica moderna y en rápida evolución, los ingenieros necesitan tener una comprensión integral del aprendizaje automático, un campo que ha revolucionado el enfoque de los problemas en diferentes industrias. Con la naturaleza en constante evolución de la tecnología, es cada vez más importante estar constantemente al tanto de los últimos avances en el aprendizaje automático. Como ingeniero puede ser imposible navegar por la enorme cantidad de literatura disponible sobre el tema. Por lo tanto, «Breve introducción al aprendizaje automático para ingenieros» es un recurso indispensable para aquellos que buscan una comprensión fundamental del aprendizaje automático. Este libro sirve como punto de partida para los ingenieros que son nuevos en este campo, proporcionando un enfoque unificado a los conceptos y principios clave, y ofreciendo referencias a la literatura para un mayor estudio. Capítulo 1: Modelos probabilísticos primer capítulo profundiza en los modelos probabilísticos que forman la base del aprendizaje automático. Abarca los fundamentos de la teoría de la probabilidad e introduce al lector en el concepto del teorema de Bayes. autores explican cómo estos principios fundamentales se aplican en los algoritmos de aprendizaje automático, permitiendo a los lectores comprender la lógica básica de estos métodos. capítulo concluye con una discusión sobre la importancia de las distribuciones probabilísticas en el aprendizaje automático y su relevancia para aplicaciones reales.
Breve introdução ao aprendizado de máquinas para engenheiros Básico e tendências no processamento de sinais Introdução: Na era tecnológica moderna em rápida evolução, os engenheiros precisam ter uma compreensão completa do aprendizado de máquinas, área que revolucionou a abordagem de problemas em vários setores. Com a natureza da tecnologia em constante evolução, é cada vez mais importante estar sempre a par dos avanços recentes no aprendizado de máquinas. Como engenheiro pode ser indefectível em uma grande quantidade de literatura disponível sobre este assunto. Por isso, a «Introdução Breve ao Aprendizado de Máquinas para Engenheiros» é um recurso indispensável para aqueles que buscam uma compreensão básica do aprendizado de máquinas. Este livro serve de ponto de partida para engenheiros que são novos na área, fornecendo uma abordagem unificada de conceitos e princípios essenciais e oferecendo referências à literatura para um estudo mais aprofundado. Capítulo 1: Os modelos prováveis Primeiro Capítulo aprofundam-se nos modelos prováveis que formam a base do aprendizado de máquina. Abrange os fundamentos da teoria da probabilidade e apresenta ao leitor o conceito de teorema de Bayes. Os autores explicam como esses princípios fundamentais são aplicados nos algoritmos de aprendizagem automática, permitindo aos leitores compreender a lógica básica dessas técnicas. O capítulo termina discutindo a importância da distribuição de probabilidades no aprendizado de máquinas e sua relevância para aplicações reais.
Breve introduzione all'apprendimento automatico per ingegneri Basi e tendenze nel trattamento dei segnali Introduzione: In un'era tecnologica moderna in rapida evoluzione, gli ingegneri devono avere una piena comprensione dell'apprendimento automatico, un campo che ha rivoluzionato l'approccio alle sfide in diversi settori. Con la natura della tecnologia in continua evoluzione, diventa sempre più importante essere sempre al corrente degli ultimi progressi nell'apprendimento automatico. Come ingegnere può essere impreparato in una grande quantità di letteratura disponibile su questo argomento. La «breve introduzione all'apprendimento automatico per gli ingegneri» è quindi una risorsa indispensabile per coloro che cercano una comprensione fondamentale dell'apprendimento automatico. Questo libro è il punto di partenza per gli ingegneri che sono nuovi in questo campo, fornendo un approccio unico ai concetti e ai principi chiave, e offrendo riferimenti alla letteratura per ulteriori ricerche. Capitolo 1: I modelli probabilistici Il primo capitolo viene approfondito nei modelli probabilistici che formano la base dell'apprendimento automatico. Copre le basi della teoria delle probabilità e fa conoscere al lettore il concetto di teorema di Bayes. Gli autori spiegano come questi principi fondamentali si applicano negli algoritmi di apprendimento automatico, permettendo ai lettori di comprendere la logica di base di questi metodi. Il capitolo si conclude con un dibattito sull'importanza della distribuzione delle probabilità nell'apprendimento automatico e sulla loro rilevanza per le applicazioni reali.
Kurze Einführung in maschinelles rnen für Ingenieure Grundlagen und Trends in der gnalverarbeitung Einleitung: In der heutigen schnelllebigen technologischen Ära benötigen Ingenieure ein umfassendes Verständnis des maschinellen rnens, ein Bereich, der die Herangehensweise an Probleme in verschiedenen Branchen revolutioniert hat. Mit der sich ständig weiterentwickelnden Natur der Technologie wird es immer wichtiger, sich ständig über die neuesten Fortschritte im Bereich des maschinellen rnens auf dem Laufenden zu halten. Als Ingenieur kann es überwältigend sein, durch die riesige Menge an Literatur zu navigieren, die zu diesem Thema verfügbar ist. Die „Kurze Einführung in maschinelles rnen für Ingenieure“ ist daher eine unverzichtbare Ressource für diejenigen, die ein grundlegendes Verständnis von maschinellem rnen suchen. Dieses Buch dient als Ausgangspunkt für Ingenieure, die neu in diesem Bereich sind, und bietet einen einheitlichen Ansatz für Schlüsselkonzepte und -prinzipien sowie Literaturverweise zur weiteren Untersuchung. Kapitel 1: Probabilistische Modelle Das erste Kapitel befasst sich mit probabilistischen Modellen, die die Grundlage für maschinelles rnen bilden. Es behandelt die Grundlagen der Wahrscheinlichkeitstheorie und führt den ser in das Konzept des Bayes-Theorems ein. Die Autoren erklären, wie diese grundlegenden Prinzipien in Algorithmen für maschinelles rnen angewendet werden, so dass die ser die zugrunde liegende Logik dieser Methoden verstehen können. Das Kapitel schließt mit einer Diskussion über die Bedeutung von Wahrscheinlichkeitsverteilungen im maschinellen rnen und ihre Relevanz für reale Anwendungen.
Krótkie wprowadzenie do uczenia maszynowego dla inżynierów Fundamenty i trendy w przetwarzaniu sygnałów Wprowadzenie: W dzisiejszej szybko rozwijającej się erze technologicznej inżynierowie muszą mieć kompleksowe zrozumienie uczenia maszynowego, dziedziny, która zrewolucjonizowała sposób, w jaki podchodzimy do problemów w różnych branżach. Wraz z stale rozwijającym się charakterem technologii, coraz ważniejsze staje się, aby śledzić najnowsze postępy w nauce maszyn. Jako inżynier, to może być przytłaczające, aby poruszać się ogromną ilość literatury dostępnej w tej kwestii. Dlatego „Krótkie wprowadzenie do uczenia maszynowego dla inżynierów” jest niezbędnym zasobem dla tych, którzy szukają podstawowego zrozumienia uczenia maszynowego. Książka ta stanowi punkt wyjścia dla inżynierów, którzy są nowi w tej dziedzinie, zapewniając jednolite podejście do kluczowych pojęć i zasad, a także oferując odniesienia do literatury do dalszych badań. Rozdział 1: Modele probabilistyczne Pierwszy rozdział przechodzi w modele probabilistyczne, które stanowią podstawę uczenia maszynowego. Obejmuje podstawy teorii prawdopodobieństwa i wprowadza czytelnika do koncepcji teorii Bayesa. Autorzy wyjaśniają jak te podstawowe zasady są stosowane w algorytmach uczenia maszynowego, pozwalając czytelnikom zrozumieć podstawową logikę tych metod. Rozdział kończy się dyskusją na temat znaczenia dystrybucji prawdopodobieństwa w nauce maszynowej i ich znaczenia dla zastosowań w świecie rzeczywistym.
מבוא קצר ללמידת מכונה עבור מהנדסים יסודיים ומגמות במבוא לעיבוד אותות: בעידן הטכנולוגי המתפתח במהירות, מהנדסים צריכים הבנה מקיפה של למידת מכונה, עם הטבע המתפתח של טכנולוגיה, זה הופך להיות יותר ויותר חשוב לשמור על מעודכן של ההתקדמות האחרונה בלמידת מכונה. כמהנדס, זה יכול להיות מכריע לנווט הכמות העצומה של ספרות זמינה בנושא זה. לכן, ”מבוא קצר ללמידת מכונה עבור מהנדסים” הוא משאב הכרחי עבור אלה המחפשים הבנה בסיסית של למידת מכונה. ספר זה משמש נקודת התחלה למהנדסים חדשים בתחום, המספקים גישה מאוחדת למושגי מפתח ועקרונות, וכן מציעים התייחסויות לספרות למחקר נוסף. פרק 1: מודלים הסתברותיים הפרק הראשון מתעמק במודלים הסתברותיים הוא מכסה את היסודות של תורת ההסתברות ומציג את הקורא למושג משפט בייס. המחברים מסבירים כיצד עקרונות יסוד אלה מיושמים באלגוריתמי למידת מכונה, ומאפשרים לקוראים להבין את הלוגיקה הבסיסית של שיטות אלה. הפרק מסתיים בדיון על חשיבותן של התפלגויות הסתברותיות בלמידת מכונה ועל הרלוונטיות שלהן ליישומים בעולם האמיתי.''
Mühendisler için Makine Öğrenimine Kısa Bir Giriş nyal İşlemede Temel Bilgiler ve Eğilimler Giriş: Günümüzün hızla gelişen teknolojik çağında, mühendislerin endüstrilerdeki sorunlara nasıl yaklaştığımız konusunda devrim yaratan bir alan olan makine öğrenimi konusunda kapsamlı bir anlayışa sahip olmaları gerekir. Teknolojinin sürekli gelişen doğası ile, makine öğrenimindeki en son gelişmelerden haberdar olmak giderek daha önemli hale geliyor. Bir mühendis olarak, bu konuda mevcut olan çok miktarda literatürde gezinmek çok zor olabilir. Bu nedenle, "Mühendisler için Makine Öğrenimine Kısa Bir Giriş", makine öğreniminin temel bir anlayışını arayanlar için vazgeçilmez bir kaynaktır. Bu kitap, alanda yeni olan mühendisler için bir başlangıç noktası olarak hizmet etmekte, temel kavram ve ilkelere birleşik bir yaklaşım sağlamakta ve daha fazla çalışma için literatüre referanslar sunmaktadır. Bölüm 1: Olasılıksal Modeller İlk bölüm makine öğreniminin temelini oluşturan olasılıksal modelleri inceler. Olasılık teorisinin temellerini kapsar ve okuyucuya Bayes teoremi kavramını tanıtır. Yazarlar, bu temel ilkelerin makine öğrenme algoritmalarında nasıl uygulandığını açıklayarak, okuyucuların bu yöntemlerin altında yatan mantığı anlamalarını sağlar. Bu bölüm, makine öğreniminde olasılık dağılımlarının önemi ve bunların gerçek dünya uygulamaları ile ilgisi üzerine bir tartışma ile sona ermektedir.
مقدمة موجزة للتعلم الآلي للمهندسين الأساسيات والاتجاهات في معالجة الإشارات مقدمة: في العصر التكنولوجي سريع التطور اليوم، يحتاج المهندسون إلى فهم شامل للتعلم الآلي، وهو مجال أحدث ثورة في كيفية تعاملنا مع المشكلات عبر الصناعات. مع الطبيعة المتطورة باستمرار للتكنولوجيا، أصبح من المهم بشكل متزايد مواكبة أحدث التطورات في التعلم الآلي. كمهندس، قد يكون من الساحق التنقل في الكم الهائل من الأدبيات المتاحة حول هذه القضية. لذلك، فإن «مقدمة موجزة للتعلم الآلي للمهندسين» هي مورد لا غنى عنه لأولئك الذين يبحثون عن فهم أساسي للتعلم الآلي. يعمل هذا الكتاب كنقطة انطلاق للمهندسين الجدد في هذا المجال، حيث يوفر نهجًا موحدًا للمفاهيم والمبادئ الرئيسية، بالإضافة إلى تقديم إشارات إلى الأدبيات لمزيد من الدراسة. الفصل 1: النماذج الاحتمالية يتعمق الفصل الأول في النماذج الاحتمالية التي تشكل أساس التعلم الآلي. يغطي أساسيات نظرية الاحتمالات ويقدم القارئ إلى مفهوم مبرهنة بايز. يشرح المؤلفون كيفية تطبيق هذه المبادئ الأساسية في خوارزميات التعلم الآلي، مما يسمح للقراء بفهم المنطق الأساسي لهذه الأساليب. يختتم الفصل بمناقشة أهمية التوزيعات المحتملة في التعلم الآلي وصلتها بتطبيقات العالم الحقيقي.
신호 처리 소개의 엔지니어 기초 및 트렌드를위한 기계 학습에 대한 간략한 소개: 오늘날의 빠르게 진화하는 기술 시대에 엔지니어는 기계 학습에 대한 포괄적 인 이해가 필요합니다. 끊임없이 진화하는 기술의 특성으로 인해 머신 러닝의 최신 발전을 파악하는 것이 점점 중요 해지고 있습니다. 엔지니어로서이 문제에 대해 이용할 수있는 방대한 양의 문헌을 탐색하는 것은 압도적 일 수 있습니다. 따라서 "엔지니어를위한 기계 학습에 대한 간략한 소개" 는 기계 학습에 대한 근본적인 이해를 원하는 사람들에게 없어서는 안될 리소스입니다. 이 책은 현장에 새로운 엔지니어를위한 출발점 역할을하며 주요 개념과 원칙에 대한 통일 된 접근 방식을 제공하고 추가 연구를위한 문헌에 대한 참조를 제공합니다. 1 장: 확률 모델 첫 번째 장은 기계 학습의 기초를 형성하는 확률 적 모델을 탐구합니다. 그것은 확률 이론의 기본을 다루고 독자에게 Bayes 정리의 개념을 소개합니다. 저자는 이러한 기본 원리가 머신 러닝 알고리즘에 어떻게 적용되는지 설명하여 독자가 이러한 방법의 기본 논리를 이해할 수 있도록합니다. 이 장은 머신 러닝에서 확률 분포의 중요성과 실제 응용 프로그램과의 관련성에 대한 토론으로 마무리됩니다.
エンジニアのための機械学習の基礎と信号処理の傾向の概要:今日急速に進化している技術時代において、エンジニアは機械学習を包括的に理解する必要があります。進化し続ける技術の本質により、機械学習の最新の進歩を常に把握することがますます重要になっています。エンジニアとして、この問題で利用可能な膨大な文献をナビゲートすることは圧倒的です。したがって「、エンジニアのための機械学習の簡単な紹介」は、機械学習の基本的な理解を求める人々にとって不可欠なリソースです。この本は、この分野を初めて経験したエンジニアの出発点となり、主要な概念と原則への統一されたアプローチを提供し、さらに研究のための文献への参照を提供します。第1章:確率モデル第1章では、機械学習の基礎となる確率モデルを掘り下げます。確率理論の基礎をカバーし、読者にベイズの定理の概念を紹介する。著者たちは、これらの基本原理が機械学習アルゴリズムにどのように適用されるかを説明し、読者はこれらの方法の根本的な論理を理解することができる。この章では、機械学習における確率分布の重要性と実際のアプリケーションとの関連性について議論します。
工程師對機器學習的簡要介紹信號處理的基礎和趨勢介紹:在當今快速發展的技術時代,工程師需要對機器學習有全面的了解,該領域徹底改變了解決各個行業問題的方法。隨著技術的不斷發展,不斷了解機器學習的最新進展變得越來越重要。作為一名工程師,瀏覽有關該問題的大量文獻可能是不可能的。因此,「工程師的機器學習簡介」對於那些尋求對機器學習的基本理解的人來說是不可或缺的資源。本書為在該領域嶄露頭角的工程師提供了起點,為關鍵概念和原則提供了統一的方法,並提供了文獻參考以供進一步研究。第一章概率模型深入研究構成機器學習基礎的概率模型。它涵蓋了概率論的基礎,並向讀者介紹了貝葉斯定理的概念。作者解釋了這些基本原理如何在機器學習算法中應用,從而使讀者能夠理解這些方法的基本邏輯。本章最後討論了概率分布在機器學習中的重要性及其與實際應用的相關性。
