
BOOKS - PROGRAMMING - Machine Learning with Neural Networks An Introduction for Scien...

Machine Learning with Neural Networks An Introduction for Scientists and Engineers
Author: B. Mehlig
Year: 2022
Pages: 261
Format: PDF
File size: 10 MB
Language: ENG
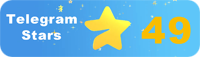
Year: 2022
Pages: 261
Format: PDF
File size: 10 MB
Language: ENG
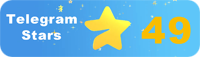
The content is organized into four main parts: Part I presents the basics of probability theory statistics and linear algebra needed for an understanding of the field Part II introduces the core ideas of machine learning and describes the most popular algorithms used in practice Part III delves into the heart of the subject presenting advanced topics such as backpropagation and the neural network paradigm Part IV discusses the most recent advances in the field including deep learning and neural networks for big data and large scale learning The book "Machine Learning with Neural Networks: An Introduction for Scientists and Engineers" is a comprehensive guide to understanding the principles and practices of machine learning with neural networks, written in a clear and accessible style. The book covers the mathematical foundations of the topic, its historical evolution, and its connections to underlying methods from statistical physics. It is designed as a self-contained text that can be used by undergraduate students in physics, mathematics, and engineering, providing a solid introduction to the key aspects of modern machine learning with artificial neural networks. Part I of the book focuses on the basics of probability theory, statistics, and linear algebra, laying the foundation for understanding the field of machine learning. This part provides the necessary background information to appreciate the concepts and techniques discussed later in the book.
Содержание состоит из четырех основных частей: В части I представлены основы статистики теории вероятностей и линейной алгебры, необходимые для понимания области. В части II представлены основные идеи машинного обучения и описаны наиболее популярные алгоритмы, используемые на практике. Часть III углубляется в суть предмета, представляя продвинутые темы, такие как обратное распространение и парадигма нейронной сети. Часть IV обсуждает самые последние достижения в этой области, включая глубокое обучение и нейронные сети для больших данных и крупномасштабного обучения книга "Машинное обучение с нейронными сетями: Введение для ученых и инженеров" - комплексное руководство по пониманию принципов и практик машинного обучения с помощью нейронных сетей, написанное в понятном и доступном стиле. Книга охватывает математические основы темы, её историческую эволюцию и её связи с лежащими в основе методами из статистической физики. Он разработан как самодостаточный текст, который может использоваться студентами бакалавриата по физике, математике и инженерии, обеспечивая основательное введение в ключевые аспекты современного машинного обучения с искусственными нейронными сетями. Часть I книги посвящена основам теории вероятностей, статистики и линейной алгебры, закладывая основу для понимания области машинного обучения. Эта часть предоставляет необходимую справочную информацию, чтобы оценить концепции и методы, обсуждаемые позже в книге.
contenu se compose de quatre parties principales : La partie I présente les bases des statistiques de la théorie des probabilités et de l'algèbre linéaire nécessaires pour comprendre le domaine. La partie II présente les idées de base de l'apprentissage automatique et décrit les algorithmes les plus populaires utilisés dans la pratique. La partie III explore l'essence du sujet en présentant des sujets avancés tels que la propagation inverse et le paradigme du réseau neuronal. La partie IV traite des progrès les plus récents dans ce domaine, y compris l'apprentissage profond et les réseaux neuronaux pour les grandes données et l'apprentissage à grande échelle, un livre intitulé « L'apprentissage automatique avec les réseaux neuronaux : une introduction pour les scientifiques et les ingénieurs » - un guide complet pour comprendre les principes et les pratiques de l'apprentissage automatique à l'aide des réseaux neuronaux, écrit dans un style compréhensible et accessible. livre couvre les fondements mathématiques du sujet, son évolution historique et ses liens avec les méthodes sous-jacentes de la physique statistique. Il est conçu comme un texte autosuffisant qui peut être utilisé par les étudiants de premier cycle en physique, mathématiques et ingénierie, offrant une introduction approfondie aux aspects clés de l'apprentissage automatique moderne avec des réseaux neuronaux artificiels. La première partie du livre traite des fondements de la théorie des probabilités, des statistiques et de l'algèbre linéaire, jetant les bases de la compréhension du domaine de l'apprentissage automatique. Cette partie fournit les informations de base nécessaires pour évaluer les concepts et les méthodes discutés plus loin dans le livre.
contenido consta de cuatro partes principales: La parte I presenta los fundamentos de la estadística de la teoría de la probabilidad y el álgebra lineal necesarios para entender el dominio. La Parte II presenta las ideas básicas del aprendizaje automático y describe los algoritmos más populares utilizados en la práctica. La parte III profundiza en la esencia del tema, presentando temas avanzados como la propagación inversa y el paradigma de la red neuronal. La Parte IV analiza los avances más recientes en este campo, incluyendo el aprendizaje profundo y las redes neuronales para el big data y el aprendizaje a gran escala, el libro «Machine arning con redes neuronales: Una introducción para científicos e ingenieros», una guía integral para entender los principios y prácticas del machine learning con redes neuronales, escrita en un estilo comprensible y accesible. libro abarca los fundamentos matemáticos del tema, su evolución histórica y sus conexiones con los métodos subyacentes a partir de la física estadística. Está diseñado como un texto autosuficiente que puede ser utilizado por estudiantes de pregrado en Física, Matemáticas e Ingeniería, proporcionando una introducción minuciosa a los aspectos clave del aprendizaje automático moderno con redes neuronales artificiales. La Parte I del libro trata de los fundamentos de la teoría de la probabilidad, la estadística y el álgebra lineal, sentando las bases para la comprensión del campo del aprendizaje automático. Esta parte proporciona la información de referencia necesaria para evaluar los conceptos y métodos discutidos más adelante en el libro.
O conteúdo é composto por quatro partes principais: a parte I apresenta as estatísticas de teoria de probabilidade e álgebra linear necessárias para compreender a área. A parte II apresenta as principais ideias de aprendizado de máquina e descreve os algoritmos mais populares usados na prática. A Parte III aprofunda-se na essência da matéria, apresentando temas avançados, como a disseminação inversa e o paradigma da rede neural. A parte IV discute os avanços mais recentes neste campo, incluindo o aprendizado profundo e as redes neurais de big data e aprendizagem em larga escala, o livro «Aprendizagem de máquinas com redes neurais: Introdução para cientistas e engenheiros», um guia completo para compreender os princípios e práticas de aprendizagem de máquinas através de redes neurais, escrito em estilo compreensível e acessível. O livro abrange os fundamentos matemáticos do tema, sua evolução histórica e suas conexões com os métodos subjacentes da física estatística. Ele foi concebido como um texto autossuficiente que pode ser usado por estudantes de graduação em Física, Matemática e Engenharia, fornecendo uma introdução fundamental a aspectos essenciais do aprendizado moderno de máquinas com redes neurais artificiais. A parte I do livro trata dos fundamentos da teoria da probabilidade, estatística e álgebra linear, criando as bases para a compreensão do campo da aprendizagem de máquinas. Esta parte fornece as informações de referência necessárias para avaliar os conceitos e métodos mais tarde discutidos no livro.
Il contenuto è costituito da quattro parti principali: La parte I presenta le basi delle statistiche sulla teoria delle probabilità e l'algebra lineare necessarie per comprendere il campo. La parte II presenta le idee di base per l'apprendimento automatico e descrive gli algoritmi più popolari utilizzati in pratica. La parte III approfondisce il tema presentando argomenti avanzati, come la diffusione inversa e il paradigma della rete neurale. La parte IV discute dei progressi più recenti in questo campo, tra cui l'apprendimento approfondito e le reti neurali per i big data e l'apprendimento su larga scala, il libro «Apprendimento automatico con le reti neurali: introduzione per scienziati e ingegneri», una guida completa alla comprensione dei principi e delle pratiche di apprendimento automatico tramite le reti neurali, scritta in modo comprensibile e accessibile. Il libro tratta le basi matematiche del tema, la sua evoluzione storica e i suoi legami con le tecniche basate sulla fisica statistica. È stato sviluppato come testo autosufficiente che può essere utilizzato dagli studenti di laurea in fisica, matematica e ingegneria, fornendo un'introduzione fondata agli aspetti chiave dell'apprendimento automatico moderno con reti neurali artificiali. La parte I del libro è incentrata sulla teoria delle probabilità, statistiche e algebra lineare, ponendo le basi per comprendere il campo dell'apprendimento automatico. Questa parte fornisce le informazioni di riferimento necessarie per valutare i concetti e i metodi discussi successivamente nel libro.
Der Inhalt besteht aus vier Hauptteilen: Teil I stellt die Grundlagen der Statistik der Wahrscheinlichkeitstheorie und der linearen Algebra vor, die für das Verständnis des Gebiets notwendig sind. Teil II stellt die wichtigsten Ideen des maschinellen rnens vor und beschreibt die beliebtesten Algorithmen, die in der Praxis verwendet werden. Teil III geht auf das Wesentliche des Themas ein und stellt fortgeschrittene Themen wie die umgekehrte Ausbreitung und das Paradigma des neuronalen Netzwerks vor. Teil IV diskutiert die jüngsten Fortschritte in diesem Bereich, einschließlich Deep arning und neuronale Netze für Big Data und Large Scale arning Buch Machine arning mit neuronalen Netzen: Eine Einführung für Wissenschaftler und Ingenieure - ein umfassender itfaden zum Verständnis der Prinzipien und Praktiken des maschinellen rnens mit neuronalen Netzen, geschrieben in einem klaren und zugänglichen Stil. Das Buch behandelt die mathematischen Grundlagen des Themas, seine historische Entwicklung und seine Verbindungen zu den zugrunde liegenden Methoden der statistischen Physik. Es ist als autarker Text konzipiert, der von Bachelor-Studenten in Physik, Mathematik und Ingenieurwissenschaften verwendet werden kann und eine fundierte Einführung in die wichtigsten Aspekte des modernen maschinellen rnens mit künstlichen neuronalen Netzen bietet. Teil I des Buches widmet sich den Grundlagen der Wahrscheinlichkeitstheorie, der Statistik und der linearen Algebra und legt den Grundstein für das Verständnis des Bereichs des maschinellen rnens. Dieser Teil liefert die notwendigen Hintergrundinformationen, um die Konzepte und Methoden zu bewerten, die später im Buch diskutiert werden.
Treść składa się z czterech głównych części: Część I przedstawia podstawy statystyki teorii prawdopodobieństwa i algebry liniowej niezbędne do zrozumienia dziedziny. Część II przedstawia podstawowe idee uczenia maszynowego i opisuje najpopularniejsze algorytmy stosowane w praktyce. Część III zagłębia się w istotę przedmiotu, przedstawiając zaawansowane tematy, takie jak backdistribution i paradygmat sieci neuronowej. Część IV omawia najnowsze postępy w tej dziedzinie, w tym głębokie sieci uczenia się i neuronowe dla dużych danych i uczenia się na dużą skalę książki „Machine arning with Neural Networks: An Introduction for Scientists and Engineers” - kompleksowy przewodnik do zrozumienia zasad i praktyk uczenia maszynowego za pomocą sieci neuronowych, napisany w zrozumiałym i dostępnym stylu. Książka obejmuje matematyczne podstawy tematu, jego ewolucję historyczną oraz powiązania z podstawowymi metodami fizyki statystycznej. Jest on zaprojektowany jako samodzielny tekst, który może być używany przez studentów licencjackich w fizyce, matematyce i inżynierii, zapewniając dokładne wprowadzenie do kluczowych aspektów nowoczesnego uczenia maszynowego ze sztucznymi sieciami neuronowymi. Część I książki dotyczy podstaw teorii prawdopodobieństwa, statystyk i algebry liniowej, kładąc podwaliny pod zrozumienie dziedziny uczenia maszynowego. Ta część zawiera informacje tła niezbędne do oceny pojęć i metod omówionych później w książce.
התוכן מורכב מארבעה חלקים עיקריים: חלק I מציג את הבסיס של הסטטיסטיקה של תורת ההסתברות ואלגברה לינארית הנחוצה להבנת השדה. חלק II מציג את הרעיונות הבסיסיים של למידת מכונה ומתאר את האלגוריתמים הפופולריים ביותר בשימוש בפועל. חלק III מתעמק בחומר הנושא, ומציג נושאים מתקדמים כמו חלוקה לאחור ופרדיגמת הרשת העצבית. חלק IV דן בהתקדמות האחרונה בתחום, כולל למידה עמוקה ורשתות עצביות עבור נתונים גדולים ולמידה בקנה מידה גדול של הספר ”Machine arning with Neural Networks: An Introduction for Sciences and Engineers” - מדריך מקיף להבנת העקרונות והפרקטיקות של למידת מכונה באמצעות רשתות עצביות, שנכתב בסגנון מובן ונגיש. הספר עוסק ביסודות המתמטיים של הנושא, בהתפתחותו ההיסטורית ובקשריו עם השיטות הבסיסיות של הפיזיקה הסטטיסטית. הוא מעוצב כטקסט עצמאי שניתן להשתמש בו על ידי סטודנטים לתואר ראשון בפיזיקה, מתמטיקה והנדסה, המספקים היכרות יסודית להיבטים מרכזיים של למידת מכונה מודרנית עם רשתות עצביות מלאכותיות. חלק I בספר עוסק ביסודות תורת ההסתברות, הסטטיסטיקה והאלגברה הליניארית, ומניח את היסודות להבנת תחום למידת המכונה. חלק זה מספק את הרקע הדרוש כדי להעריך את המושגים והשיטות שנידונו מאוחר יותר בספר.''
İçerik dört ana bölümden oluşur: Bölüm I, alanı anlamak için gerekli olan olasılık teorisi ve doğrusal cebir istatistiklerinin temellerini sunar. Bölüm II, makine öğreniminin temel fikirlerini sunar ve pratikte kullanılan en popüler algoritmaları açıklar. Bölüm III, konunun özünü inceleyerek, geri dağılım ve sinir ağı paradigması gibi gelişmiş konuları sunar. Bölüm IV, büyük veri için derin öğrenme ve sinir ağları ve büyük ölçekli öğrenme kitabı "nir Ağları ile Makine Öğrenimi: Bilim Adamları ve Mühendisler için Bir Giriş" kitabı da dahil olmak üzere alandaki en son gelişmeleri tartışıyor - sinir ağlarını kullanarak makine öğreniminin ilkelerini ve uygulamalarını anlamak için kapsamlı bir rehber, anlaşılabilir ve erişilebilir bir tarzda yazılmıştır. Kitap, konunun matematiksel temellerini, tarihsel evrimini ve istatistiksel fiziğin altında yatan yöntemlerle olan bağlantılarını kapsar. Fizik, matematik ve mühendislik lisans öğrencileri tarafından kullanılabilecek bağımsız bir metin olarak tasarlanmıştır ve yapay sinir ağları ile modern makine öğreniminin temel yönlerine kapsamlı bir giriş sağlar. Kitabın I. Bölümü, olasılık teorisi, istatistik ve doğrusal cebirin temelleri ile ilgilenir ve makine öğrenimi alanını anlamak için temel oluşturur. Bu bölüm, daha sonra kitapta tartışılan kavram ve yöntemleri değerlendirmek için gerekli arka plan bilgilerini sağlar.
يتكون المحتوى من أربعة أجزاء رئيسية: يعرض الجزء الأول أساسيات إحصاءات نظرية الاحتمالات والجبر الخطي الضروري لفهم المجال. يقدم الجزء الثاني الأفكار الأساسية للتعلم الآلي ويصف الخوارزميات الأكثر شيوعًا المستخدمة في الممارسة العملية. يتعمق الجزء الثالث في جوهر الموضوع، ويعرض مواضيع متقدمة مثل التوزيع الخلفي ونموذج الشبكة العصبية. يناقش الجزء الرابع أحدث التطورات في هذا المجال، بما في ذلك التعلم العميق والشبكات العصبية للبيانات الضخمة والتعلم على نطاق واسع كتاب «التعلم الآلي بالشبكات العصبية: مقدمة للعلماء والمهندسين» - دليل شامل لفهم مبادئ وممارسات التعلم الآلي باستخدام الشبكات العصبية، مكتوبة بأسلوب مفهوم ويمكن الوصول إليه. يغطي الكتاب الأسس الرياضية للموضوع وتطوره التاريخي وصلاته بالطرق الأساسية للفيزياء الإحصائية. تم تصميمه كنص قائم بذاته يمكن استخدامه من قبل طلاب البكالوريوس في الفيزياء والرياضيات والهندسة، مما يوفر مقدمة شاملة للجوانب الرئيسية للتعلم الآلي الحديث مع الشبكات العصبية الاصطناعية. يتناول الجزء الأول من الكتاب أساسيات نظرية الاحتمالات والإحصاءات والجبر الخطي، مما يضع الأساس لفهم مجال التعلم الآلي. يقدم هذا الجزء المعلومات الأساسية اللازمة لتقييم المفاهيم والأساليب التي تمت مناقشتها لاحقًا في الكتاب.
내용은 네 가지 주요 부분으로 구성됩니다. 파트 I은 필드를 이해하는 데 필요한 확률 이론 및 선형 대수 통계의 기본 사항을 제시합니다. 파트 II는 머신 러닝의 기본 아이디어를 제시하고 실제로 사용되는 가장 인기있는 알고리즘을 설명합니다. 파트 III은 주제의 내용을 탐구하여 역 분포 및 신경망 패러다임과 같은 고급 주제를 제시합니다. 파트 IV는 빅 데이터를위한 딥 러닝 및 신경망과 대규모 학습 "신경망을 이용한 머신 러닝: 과학자 및 엔지니어를위한 소개" 책을 포함하여이 분야에서 가장 최근의 발전에 대해 논의합니다. 이해할 수 있고 접근 가능한 스타일로 작성된 기계 학습. 이 책은 주제의 수학적 기초, 역사적 진화 및 통계 물리학의 기본 방법과의 연관성을 다룹니다. 물리, 수학 및 공학 분야의 학부생들이 사용할 수있는 독립적 인 텍스트로 설계되어 인공 신경망을 통한 현대 기계 학습의 주요 측면에 대한 철저한 소개를 제공합니다. 이 책의 1 부는 확률 이론, 통계 및 선형 대수의 기본을 다루며 머신 러닝 분야를 이해하기위한 토대를 마련합니다. 이 부분은 책에서 나중에 논의 된 개념과 방법을 평가하는 데 필요한 배경 정보를 제공합니다.
内容は4つの主要な部分から成っています:Part Iは、フィールドを理解するために必要な確率理論と線形代数の統計の基礎を提示します。パートIIは機械学習の基本的なアイデアを提示し、実際に使用される最も一般的なアルゴリズムを説明します。Part IIIは、被験者の物質を掘り下げ、バックディストリビューションやニューラルネットワークのパラダイムなどの高度なトピックを提示します。パートIVでは、ビッグデータのためのディープラーニングとニューラルネットワーク、大規模な学習を含むこの分野の最新の進歩について説明しています。この本は、このトピックの数学的基礎、その歴史的進化、および統計物理学からの基礎となる方法との関連を網羅しています。物理、数学、工学の学部生が使用できる自己完結型テキストとして設計されており、人工ニューラルネットワークを備えた現代機械学習の重要な側面を徹底的に紹介しています。本書の第一部では、確率論、統計学、線形代数学の基礎を扱っており、機械学習の分野を理解する基礎を築いている。この部分は、本の後半で議論された概念や方法を評価するために必要な背景情報を提供します。
Nekromans Opus 1: The Dark Queen's Revenge在Necro的國家,黑暗女王統治著一個鐵拳,每個生活在她王國的人都害怕和尊重。她是一個怪物,是無法形容的力量和殘酷的生物,要求臣民絕對服從。她的統治以死亡和毀滅為標誌,沒有希望或幸福的余地。但是,在這個嚴峻的景觀中,英雄的崛起預示著將導致她的垮臺並恢復王國的和平。輸入伊娃,一個輕的大提琴手選擇成為這個英雄的命運。但在她實現自己的目的之前,她遇到了一個過早的結局,被女王憤怒的受害者摔倒了。然而,死亡並不標誌著她旅程的結束。相反,它使她走上了發現死靈法典秘密的道路,死靈法典是被禁止的藝術,是永生的關鍵。在神秘的死靈法師的幫助下,夏娃被允許進行危險的搜索,以挑戰女王的統治並為Necro國家帶來光明。故事始於夏娃(Eve)的去世,但很快將註意力轉移到死靈法師身上,死靈法師是一個強大的生物,他有計劃成為輕的大提琴手。他將她視為尋求權力和永生的潛在盟友,並打算操縱她並控制她,在生存遊戲中使用她作為典當。
