
BOOKS - PROGRAMMING - Source Separation and Machine Learning

Source Separation and Machine Learning
Author: Jen-Tzung Chien
Year: 2019
Pages: 384
Format: PDF
File size: 12 MB
Language: ENG
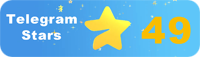
Year: 2019
Pages: 384
Format: PDF
File size: 12 MB
Language: ENG
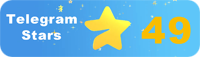
''
