
BOOKS - Green Machine Learning Protocols for Future Communication Networks

Green Machine Learning Protocols for Future Communication Networks
Author: Saim Ghafoor
Year: October 25, 2023
Format: PDF
File size: PDF 13 MB
Language: English
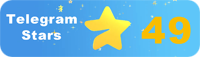
Year: October 25, 2023
Format: PDF
File size: PDF 13 MB
Language: English
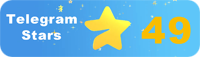
Book Green Machine Learning Protocols for Future Communication Networks Introduction: The rapid evolution of technology has led to significant advancements in various fields, including machine learning, which has revolutionized the way we approach complex problems in communication networks. However, the increasing computational requirements and energy consumption of these algorithms pose a threat to their sustainability and the survival of humanity. The need of the hour is to develop personal paradigms that can help us perceive the technological process of developing modern knowledge as the basis for the survival of humanity and the unification of people in a warring state. This book focuses on the development of green machine learning protocols that can process and analyze data efficiently while reducing energy consumption, thereby reducing the carbon footprint. Chapter 1: The Need for Green Machine Learning Protocols The chapter begins by discussing the significance of machine learning in solving complex network problems and providing situation and parameter prediction. It highlights the limitations of traditional machine learning algorithms, such as high resource consumption, and the need for lightweight protocols that can provide timely responses while minimizing energy consumption. The authors emphasize the importance of designing novel and lightweight green machine learning algorithms to reduce energy consumption and carbon footprint.
Book Green Machine arning Protocols for Future Communication Networks Введение: Быстрое развитие технологий привело к значительным достижениям в различных областях, включая машинное обучение, которое произвело революцию в подходе к сложным проблемам в сетях связи. Однако возрастающие вычислительные требования и энергопотребление этих алгоритмов представляют угрозу их устойчивости и выживанию человечества. Потребность часа - в выработке личностных парадигм, которые могут помочь нам воспринимать технологический процесс развития современного знания как основы выживания человечества и объединения людей в воюющем государстве. Эта книга посвящена разработке «зеленых» протоколов машинного обучения, которые могут эффективно обрабатывать и анализировать данные, одновременно снижая потребление энергии, тем самым уменьшая углеродный след. Глава 1: Потребность в «зеленых» протоколах машинного обучения Глава начинается с обсуждения значимости машинного обучения в решении сложных сетевых задач и обеспечения прогнозирования ситуации и параметров. В нем подчеркиваются ограничения традиционных алгоритмов машинного обучения, такие как высокое потребление ресурсов, и необходимость в легковесных протоколах, которые могут обеспечить своевременную реакцию при минимизации энергопотребления. Авторы подчеркивают важность разработки новых и легких «зеленых» алгоритмов машинного обучения для снижения энергопотребления и углеродного следа.
Book Green Machine Arning Protocols for Future Communication Networks Introduction : développement rapide de la technologie a conduit à des progrès importants dans divers domaines, y compris l'apprentissage automatique, qui a révolutionné l'approche des problèmes complexes dans les réseaux de communication. Cependant, les exigences informatiques et la consommation énergétique croissantes de ces algorithmes menacent leur résilience et la survie de l'humanité. besoin d'une heure est d'élaborer des paradigmes personnels qui peuvent nous aider à percevoir le processus technologique du développement des connaissances modernes comme les fondements de la survie de l'humanité et de l'unification des gens dans un État en guerre. Ce livre est consacré au développement de protocoles d'apprentissage automatique « verts » qui peuvent traiter et analyser efficacement les données tout en réduisant la consommation d'énergie, réduisant ainsi l'empreinte carbone Chapitre 1 : La nécessité de protocoles d'apprentissage automatique « verts » chapitre commence par discuter de l'importance de l'apprentissage automatique dans la résolution de problèmes complexes de réseau et la prévision de la situation et des paramètres. Il souligne les limites des algorithmes d'apprentissage automatique traditionnels, tels que la consommation élevée de ressources, et la nécessité de protocoles légers qui peuvent fournir une réponse rapide tout en minimisant la consommation d'énergie. s auteurs soulignent l'importance de développer de nouveaux algorithmes d'apprentissage automatique « verts » légers pour réduire la consommation d'énergie et l'empreinte carbone.
Book Green Machine Arning Protocols for Future Communication Networks Introducción: rápido desarrollo de la tecnología ha dado lugar a avances significativos en varios campos, incluido el aprendizaje automático, que ha revolucionado el enfoque de los problemas complejos en las redes de comunicación. n embargo, los crecientes requerimientos computacionales y el consumo de energía de estos algoritmos representan una amenaza para su sostenibilidad y la supervivencia de la humanidad. La necesidad de la hora es generar paradigmas personales que nos puedan ayudar a percibir el proceso tecnológico del desarrollo del conocimiento moderno como la base de la supervivencia de la humanidad y la unión de las personas en un Estado en guerra. Este libro se centra en el desarrollo de protocolos de aprendizaje automático «ecológicos» que pueden procesar y analizar los datos de manera eficiente, reduciendo al mismo tiempo el consumo de energía, reduciendo así la huella de carbón.Capítulo 1: Necesidad de protocolos de aprendizaje automático «ecológicos» capítulo comienza discutiendo la importancia del aprendizaje automático en la resolución de problemas de red complejos y asegurando la predicción de la situación y los parámetros. Destaca las limitaciones de los algoritmos tradicionales de aprendizaje automático, como el alto consumo de recursos, y la necesidad de protocolos ligeros que puedan proporcionar una respuesta oportuna mientras se minimiza el consumo de energía. autores subrayan la importancia de desarrollar algoritmos nuevos y ligeros de aprendizaje automático «verde» para reducir el consumo de energía y la huella de carbono.
Book Green Machine Arning Protocols for Future Comunicação Networks Introdução: O rápido desenvolvimento da tecnologia levou a avanços significativos em várias áreas, incluindo o aprendizado de máquinas, que revolucionou a abordagem de problemas complexos nas redes de comunicação. No entanto, as crescentes exigências computacionais e o consumo de energia desses algoritmos representam uma ameaça à sua estabilidade e sobrevivência humana. A necessidade da hora é desenvolver paradigmas de personalidade que possam nos ajudar a considerar o processo tecnológico de desenvolvimento do conhecimento moderno como a base da sobrevivência humana e da união das pessoas num Estado em guerra. Este livro trata do desenvolvimento de protocolos verdes de aprendizagem de máquinas que podem processar e analisar os dados de forma eficiente, ao mesmo tempo em que reduz o consumo de energia, reduzindo assim a pegada de carbono. Ele enfatiza as limitações dos algoritmos tradicionais de aprendizagem de máquinas, como o alto consumo de recursos, e a necessidade de protocolos leves que possam garantir uma resposta pontual ao minimizar o consumo de energia. Os autores destacam a importância de desenvolver novos e leves algoritmos de aprendizagem de máquinas para reduzir o consumo de energia e a pegada de carbono.
Book Green Machine arning Protocols for Future Communication Networks Introduzione: il rapido sviluppo della tecnologia ha portato a notevoli progressi in diversi settori, tra cui l'apprendimento automatico, che ha rivoluzionato l'approccio alle sfide complesse delle reti di comunicazione. Tuttavia, i crescenti requisiti di elaborazione e il consumo energetico di questi algoritmi minacciano la loro stabilità e la sopravvivenza dell'umanità. Il bisogno dell'ora è quello di sviluppare paradigmi personali che possano aiutarci a considerare il processo tecnologico di sviluppo della conoscenza moderna come la base della sopravvivenza dell'umanità e dell'unione delle persone in uno stato in guerra. Questo libro è dedicato allo sviluppo di protocolli di apprendimento automatico green in grado di elaborare e analizzare i dati in modo efficiente, riducendo al contempo il consumo di energia, riducendo così le tracce di carbonio. Sottolinea i limiti dei tradizionali algoritmi di apprendimento automatico, come ad esempio l'elevato consumo di risorse, e la necessità di protocolli leggeri in grado di garantire una risposta tempestiva al minimo consumo energetico. Gli autori sottolineano l'importanza di sviluppare algoritmi di apprendimento automatico «green» nuovi e leggeri per ridurre il consumo energetico e l'impronta di carbonio.
Buch Grüne maschinelle rnprotokolle für zukünftige Kommunikationsnetzwerke Einleitung: Die rasante Entwicklung der Technologie hat zu bedeutenden Fortschritten in verschiedenen Bereichen geführt, einschließlich des maschinellen rnens, das die Herangehensweise an komplexe Probleme in Kommunikationsnetzwerken revolutioniert hat. Die steigenden Rechenanforderungen und der Energieverbrauch dieser Algorithmen stellen jedoch eine Bedrohung für ihre Nachhaltigkeit und das Überleben der Menschheit dar. Die Notwendigkeit der Stunde besteht darin, persönliche Paradigmen zu entwickeln, die uns helfen können, den technologischen Prozess der Entwicklung des modernen Wissens als Grundlage für das Überleben der Menschheit und die Vereinigung der Menschen in einem kriegführenden Staat wahrzunehmen. Dieses Buch konzentriert sich auf die Entwicklung von „grünen“ Machine-arning-Protokollen, die Daten effizient verarbeiten und analysieren können, während sie den Energieverbrauch senken und damit den CO2-Fußabdruck reduzieren.Kapitel 1: Die Notwendigkeit von „grünen“ Machine-arning-ProtokollenDas Kapitel beginnt mit einer Diskussion über die Bedeutung des maschinellen rnens bei der Lösung komplexer Netzwerkprobleme und der Bereitstellung von tuations- und Parametervorhersagen. Es hebt die Grenzen herkömmlicher Algorithmen für maschinelles rnen hervor, wie den hohen Ressourcenverbrauch und die Notwendigkeit von leichtgewichtigen Protokollen, die eine zeitnahe Reaktion bei gleichzeitiger Minimierung des Energieverbrauchs gewährleisten können. Die Autoren betonen die Bedeutung der Entwicklung neuer und leichter „grüner“ Algorithmen für maschinelles rnen, um den Energieverbrauch und den CO2-Fußabdruck zu reduzieren.
Book Green Machine arning Protocols for Future Communication Networks Wprowadzenie: Szybki rozwój technologii doprowadził do znacznych postępów w różnych dziedzinach, w tym uczenia maszynowego, który zrewolucjonizował podejście do złożonych problemów w sieciach komunikacyjnych. Jednak rosnące wymagania obliczeniowe i zużycie energii przez te algorytmy stanowią zagrożenie dla ich stabilności i przetrwania ludzkości. Potrzeba godziny polega na opracowaniu osobistych paradygmatów, które pomogą nam postrzegać technologiczny proces rozwoju nowoczesnej wiedzy jako podstawę przetrwania ludzkości i zjednoczenia ludzi w stanie wojennym. Książka ta koncentruje się na opracowaniu „zielonych” protokołów uczenia maszynowego, które mogą skutecznie przetwarzać i analizować dane, zmniejszając jednocześnie zużycie energii, zmniejszając tym samym ślad węglowy. Rozdział 1: Potrzeba „zielonych” protokołów uczenia maszynowego Rozdział rozpoczyna się od omówienia znaczenia uczenia maszynowego w rozwiązywaniu złożonych problemów sieciowych i zapewnianiu prognozy sytuacji i parametrów. Podkreśla ograniczenia tradycyjnych algorytmów uczenia maszynowego, takich jak wysokie zużycie zasobów, oraz potrzebę lekkich protokołów, które mogą zapewnić szybką reakcję przy jednoczesnym minimalizacji zużycia energii. Autorzy podkreślają znaczenie opracowania nowych i lekkich „zielonych” algorytmów uczenia maszynowego w celu zmniejszenia zużycia energii i śladu węglowego.
Book Green Machine Arning Protocols for Future Communication Networks Introduction: הפיתוח המהיר של הטכנולוגיה הוביל להתקדמות משמעותית בתחומים שונים, כולל למידת מכונה, שחוללה מהפכה בגישה לבעיות מורכבות ברשתות תקשורת. עם זאת, דרישות החישוב הגוברות וצריכת החשמל של אלגוריתמים אלה מהווים איום ליציבותם ולהישרדות האנושות. הצורך של השעה הוא לפתח פרדיגמות אישיות שיכולות לעזור לנו לתפוס את התהליך הטכנולוגי של פיתוח הידע המודרני כבסיס להישרדות האנושות ולאיחוד של אנשים במדינה לוחמת. ספר זה מתמקד בפיתוח פרוטוקולי למידת מכונה ”ירוקה” המסוגלים לעבד ולנתח נתונים ביעילות תוך צמצום צריכת האנרגיה, ובכך לצמצם את טביעת הרגל הפחמנית. פרק 1: הצורך בפרוטוקולי למידת מכונה ”ירוקה” הפרק מתחיל בדיון על החשיבות של למידת מכונה בפתרון בעיות רשת מורכבות הוא מדגיש את מגבלותיהם של אלגוריתמי למידת מכונה מסורתיים, כגון צריכת משאבים גבוהה, ואת הצורך בפרוטוקולים קלים שיכולים לספק מענה בזמן תוך צמצום צריכת החשמל. המחברים מדגישים את החשיבות של פיתוח אלגוריתמי למידת מכונה ”ירוקה” חדשים וקלים משקל להפחתת צריכת אנרגיה וטביעת רגל פחמנית.''
Book Green Machine arning Protocols for Future Communication Networks Giriş: Teknolojinin hızlı gelişimi, iletişim ağlarındaki karmaşık sorunlara yaklaşımda devrim yaratan makine öğrenimi de dahil olmak üzere çeşitli alanlarda önemli gelişmelere yol açmıştır. Bununla birlikte, bu algoritmaların artan hesaplama gereksinimleri ve güç tüketimi, istikrarı ve insanlığın hayatta kalması için bir tehdit oluşturmaktadır. Saatin ihtiyacı, modern bilginin gelişiminin teknolojik sürecini insanlığın hayatta kalması ve insanların savaşan bir durumda birleşmesi için temel olarak algılamamıza yardımcı olabilecek kişisel paradigmalar geliştirmektir. Bu kitap, enerji tüketimini azaltırken verileri verimli bir şekilde işleyebilen ve analiz edebilen ve böylece karbon ayak izini azaltan'yeşil "makine öğrenimi protokollerinin geliştirilmesine odaklanmaktadır. Bölüm 1:'yeşil "makine öğrenimi protokollerine duyulan ihtiyaç Bölüm, karmaşık ağ problemlerini çözmede ve durum ve parametrelerin tahminini sağlamada makine öğreniminin öneminin tartışılmasıyla başlar. Yüksek kaynak tüketimi gibi geleneksel makine öğrenme algoritmalarının sınırlamalarını ve güç tüketimini en aza indirirken zamanında yanıt verebilecek hafif protokollere duyulan ihtiyacı vurgular. Yazarlar, enerji tüketimini ve karbon ayak izini azaltmak için yeni ve hafif'yeşil "makine öğrenme algoritmaları geliştirmenin önemini vurgulamaktadır.
كتاب بروتوكولات التعلم الآلي الأخضر لشبكات الاتصالات المستقبلية مقدمة: أدى التطور السريع للتكنولوجيا إلى تقدم كبير في مختلف المجالات، بما في ذلك التعلم الآلي، مما أحدث ثورة في نهج المشاكل المعقدة في شبكات الاتصالات. ومع ذلك، فإن المتطلبات الحسابية المتزايدة واستهلاك الطاقة لهذه الخوارزميات يشكل تهديدًا لاستقرارها وبقاء البشرية. إن حاجة الساعة هي تطوير نماذج شخصية يمكن أن تساعدنا على إدراك العملية التكنولوجية لتطوير المعرفة الحديثة كأساس لبقاء البشرية وتوحيد الناس في دولة متحاربة. يركز هذا الكتاب على تطوير بروتوكولات التعلم الآلي «الخضراء» التي يمكنها معالجة البيانات وتحليلها بكفاءة مع تقليل استهلاك الطاقة، وبالتالي تقليل البصمة الكربونية. الفصل 1: الحاجة إلى بروتوكولات التعلم الآلي «الخضراء» يبدأ الفصل بمناقشة أهمية التعلم الآلي في حل مشاكل الشبكة المعقدة وتوفير التنبؤ بالوضع والمعايير. يسلط الضوء على قيود خوارزميات التعلم الآلي التقليدية، مثل الاستهلاك العالي للموارد، والحاجة إلى بروتوكولات خفيفة الوزن يمكن أن توفر استجابة في الوقت المناسب مع تقليل استهلاك الطاقة. يؤكد المؤلفون على أهمية تطوير خوارزميات جديدة وخفيفة الوزن للتعلم الآلي «الأخضر» لتقليل استهلاك الطاقة والبصمة الكربونية.
Book Green Machine Arning Protocols for Future Communication Networks簡介:技術的快速發展在包括機器學習在內的各個領域取得了重大進展,徹底改變了解決通信網絡復雜問題的方法。但是,這些算法的計算需求和功耗的增加對它們的可持續性和人類的生存構成了威脅。一個小時的需要是建立個人範式,可以幫助我們將現代知識發展的技術過程視為人類生存和交戰國人民團結的基礎。本書致力於開發「綠色」機器學習協議,這些協議可以有效地處理和分析數據,同時降低能耗,從而減少碳足跡。第一章:需要「綠色」機器學習協議本章首先討論機器學習在解決復雜網絡問題方面的重要性,並提供情況和參數預測。它強調了傳統機器學習算法的局限性,例如高資源消耗,以及對輕量級協議的需求,這些協議可以在最大程度地減少功耗的同時提供及時響應。作者強調了開發新型和輕型「綠色」機器學習算法以減少能耗和碳足跡的重要性。
