
BOOKS - Stochastic Methods for Modeling and Predicting Complex Dynamical Systems: Unc...

Stochastic Methods for Modeling and Predicting Complex Dynamical Systems: Uncertainty Quantification, State Estimation, and Reduced-Order Models (Synthesis Lectures on Mathematics and Statistics)
Author: Nan Chen
Year: March 13, 2023
Format: PDF
File size: PDF 24 MB
Language: English
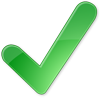
Year: March 13, 2023
Format: PDF
File size: PDF 24 MB
Language: English
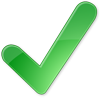
Book Stochastic Methods for Modeling and Predicting Complex Dynamical Systems: Uncertainty Quantification, State Estimation, and Reduced-Order Models Introduction: In today's rapidly evolving technological landscape, it is crucial to understand the process of technology development and its impact on humanity. The book "Stochastic Methods for Modeling and Predicting Complex Dynamical Systems" provides a comprehensive guide to modeling and predicting complex systems using stochastic tools, highlighting the importance of developing a personal paradigm for perceiving the technological process of developing modern knowledge. This book is an essential resource for researchers and practitioners seeking to understand and predict complex dynamical systems, particularly those with uncertainty and complexity. Chapter 1: Introduction to Stochastic Methods The first chapter introduces the fundamental concepts of stochastic methods for modeling and predicting complex dynamical systems. The author emphasizes the need to balance computational efficiency and modeling accuracy, providing readers with practical ideas to build useful models. The chapter covers the basics of stochastic processes, probability distributions, and statistical inference, laying the foundation for the subsequent chapters. Chapter 2: Qualitative and Quantitative Modeling Approaches This chapter explores the interplay between qualitative and quantitative modeling approaches, showcasing the importance of combining both to achieve accurate predictions. The author discusses the relevance of physical intuitions and thinking in understanding complex systems, highlighting the need for a comprehensive approach that integrates mathematical theories, computational science, and data science.
Book Stochastic Methods for Modeling and Predicting Complex Dynamic Systems: Uncertainty Quantification, State Estimation, and Reduced-Order Models Введение: В современном быстро развивающемся технологическом ландшафте крайне важно понимать процесс развития технологий и его влияние на человечество. В книге «Стохастические методы моделирования и прогнозирования сложных динамических систем» представлено комплексное руководство по моделированию и прогнозированию сложных систем с использованием стохастических инструментов, подчёркивающее важность выработки личностной парадигмы восприятия технологического процесса развития современных знаний. Эта книга является важным ресурсом для исследователей и практиков, стремящихся понять и предсказать сложные динамические системы, особенно те, которые имеют неопределенность и сложность. Глава 1: Введение в стохастические методы В первой главе представлены фундаментальные концепции стохастических методов моделирования и прогнозирования сложных динамических систем. Автор подчеркивает необходимость сбалансировать вычислительную эффективность и точность моделирования, предоставляя читателям практические идеи для построения полезных моделей. Глава охватывает основы стохастических процессов, распределения вероятностей и статистического вывода, закладывая основу для последующих глав. Глава 2: Подходы к качественному и количественному моделированию В этой главе рассматривается взаимодействие между подходами к качественному и количественному моделированию, демонстрируя важность сочетания обоих подходов для достижения точных прогнозов. Автор обсуждает актуальность физических интуиций и мышления в понимании сложных систем, подчеркивая необходимость комплексного подхода, объединяющего математические теории, вычислительную науку и науку о данных.
Book Stochastic Methods for Modeling and Predicting Complex Dynamic Systems : Uncertainty Quantification, State Estimation, and Reduced-Order Models Introduction : Dans le paysage technologique en évolution rapide d'aujourd'hui, il est essentiel de comprendre le processus de développement technologique et son impact l'humanité. livre « Méthodes stochastiques de modélisation et de prévision des systèmes dynamiques complexes » présente un guide complet sur la modélisation et la prévision des systèmes complexes à l'aide d'outils stochastiques, soulignant l'importance de développer un paradigme personnel de la perception du processus technologique du développement des connaissances modernes. Ce livre est une ressource importante pour les chercheurs et les praticiens qui cherchent à comprendre et à prédire les systèmes dynamiques complexes, en particulier ceux qui ont de l'incertitude et de la complexité. Chapitre 1 : Introduction aux méthodes stochastiques premier chapitre présente les concepts fondamentaux des méthodes stochastiques de modélisation et de prévision des systèmes dynamiques complexes. L'auteur souligne la nécessité d'équilibrer l'efficacité informatique et la précision de la modélisation en fournissant aux lecteurs des idées pratiques pour construire des modèles utiles. chapitre traite des bases des processus stochastiques, de la distribution des probabilités et de la conclusion statistique, jetant les bases des chapitres suivants. Chapitre 2 : Approches de la modélisation qualitative et quantitative Ce chapitre traite de l'interaction entre les approches de la modélisation qualitative et quantitative, démontrant l'importance de combiner les deux approches pour obtenir des prévisions précises. L'auteur discute de la pertinence des intuitions physiques et de la pensée dans la compréhension des systèmes complexes, soulignant la nécessité d'une approche intégrée combinant les théories mathématiques, la science computationnelle et la science des données.
Book Stochastic Methods for Modeling and Predicting Complex Dynamic Systems: Uncertainty Quantification, State Estimation, and Redued-Order Models Introducción: En la actualidad es fundamental que el panorama tecnológico en rápida evolución comprenda el proceso de desarrollo de la tecnología y su impacto en la humanidad. libro «Técnicas estocásticas para modelar y predecir sistemas dinámicos complejos» presenta una guía integral para modelar y predecir sistemas complejos utilizando herramientas estocásticas, destacando la importancia de generar un paradigma personal para percibir el proceso tecnológico del desarrollo del conocimiento moderno. Este libro es un recurso importante para investigadores y profesionales que buscan comprender y predecir sistemas dinámicos complejos, especialmente aquellos que tienen incertidumbre y complejidad. Capítulo 1: Introducción a los métodos estocásticos primer capítulo presenta los conceptos fundamentales de las técnicas estocásticas de modelado y predicción de sistemas dinámicos complejos. autor subraya la necesidad de equilibrar la eficiencia computacional y la precisión del modelado, proporcionando a los lectores ideas prácticas para construir modelos útiles. capítulo cubre los fundamentos de los procesos estocásticos, la distribución de probabilidad y la conclusión estadística, sentando las bases para los capítulos posteriores. Capítulo 2: Enfoques de la modelización cualitativa y cuantitativa Este capítulo examina la interacción entre los enfoques de la simulación cualitativa y cuantitativa, demostrando la importancia de combinar ambos enfoques para lograr predicciones precisas. autor discute la relevancia de las intuiciones físicas y el pensamiento en la comprensión de sistemas complejos, destacando la necesidad de un enfoque complejo que combine las teorías matemáticas, la ciencia computacional y la ciencia de datos.
Buch Stochastic Methods for Modeling and Predicting Complex Dynamic Systems: Uncertainty Quantification, State Estimation, and Reduced-Order Models Einführung: In der heutigen schnelllebigen Technologielandschaft ist es entscheidend, den Prozess der Technologieentwicklung und ihre Auswirkungen auf die Menschheit zu verstehen. Das Buch „Stochastische Methoden der Modellierung und Vorhersage komplexer dynamischer Systeme“ bietet eine umfassende Anleitung zur Modellierung und Vorhersage komplexer Systeme mit stochastischen Werkzeugen und betont die Bedeutung der Entwicklung eines persönlichen Paradigmas für die Wahrnehmung des technologischen Prozesses der Entwicklung des modernen Wissens. Dieses Buch ist eine wichtige Ressource für Forscher und Praktiker, die komplexe dynamische Systeme verstehen und vorhersagen möchten, insbesondere solche mit Unsicherheit und Komplexität. Kapitel 1: Einführung in stochastische Methoden Im ersten Kapitel werden grundlegende Konzepte stochastischer Methoden zur Modellierung und Vorhersage komplexer dynamischer Systeme vorgestellt. Der Autor betont die Notwendigkeit, Recheneffizienz und mulationsgenauigkeit in Einklang zu bringen, indem er den sern praktische Ideen für den Aufbau von Gebrauchsmustern liefert. Das Kapitel behandelt die Grundlagen stochastischer Prozesse, Wahrscheinlichkeitsverteilungen und statistischer Schlussfolgerungen und legt die Grundlage für die nachfolgenden Kapitel. Kapitel 2: Ansätze zur qualitativen und quantitativen Modellierung Dieses Kapitel untersucht das Zusammenspiel von Ansätzen zur qualitativen und quantitativen Modellierung und zeigt, wie wichtig es ist, beide Ansätze zu kombinieren, um genaue Vorhersagen zu treffen. Der Autor diskutiert die Relevanz physikalischer Intuitionen und des Denkens für das Verständnis komplexer Systeme und betont die Notwendigkeit eines integrierten Ansatzes, der mathematische Theorien, Computerwissenschaft und Datenwissenschaft kombiniert.
''
Karmaşık Dinamik stemlerin Modellenmesi ve Öngörülmesi için Stokastik Yöntemler: Belirsizlik Ölçümü, Durum Tahmini ve Azaltılmış Düzen Modelleri Giriş: Günümüzün hızla gelişen teknolojik ortamında, teknoloji geliştirme sürecini ve insanlık üzerindeki etkisini anlamak çok önemlidir. "Stochastic Methods for Modeling and Forecasting Complex Dynamic Systems" (Karmaşık Dinamik stemleri Modellemek ve Tahmin Etmek için Stokastik Yöntemler) adlı kitap, modern bilgiyi geliştirmenin teknolojik sürecinin algılanması için kişisel bir paradigma geliştirmenin önemini vurgulayarak, stokastik araçları kullanarak karmaşık sistemleri modellemek ve tahmin etmek için kapsamlı bir rehber sunmaktadır. Bu kitap, karmaşık dinamik sistemleri, özellikle de belirsizlik ve karmaşıklığa sahip olanları anlamak ve tahmin etmek isteyen araştırmacılar ve uygulayıcılar için önemli bir kaynaktır. Bölüm 1: Stokastik Yöntemlere Giriş İlk bölüm, karmaşık dinamik sistemleri modellemek ve tahmin etmek için stokastik yöntemlerin temel kavramlarını sunar. Yazar, hesaplama verimliliğini ve modelleme doğruluğunu dengeleme ihtiyacını vurgulayarak, okuyuculara yararlı modeller oluşturmak için pratik fikirler sunar. Bölüm, stokastik süreçlerin temellerini, olasılık dağılımını ve istatistiksel çıkarımı kapsar ve sonraki bölümlerin temelini oluşturur. Bölüm 2: Nitel ve Nicel Modelleme Yaklaşımları Bu bölüm, nitel ve nicel modelleme yaklaşımları arasındaki etkileşimi inceleyerek, doğru tahminler elde etmek için her iki yaklaşımı birleştirmenin önemini göstermektedir. Yazar, fiziksel sezgilerin ve düşüncenin karmaşık sistemleri anlamadaki önemini tartışarak, matematiksel teorileri, hesaplamalı bilimi ve veri bilimini birleştiren entegre bir yaklaşıma duyulan ihtiyacı vurgulamaktadır.
كتاب الأساليب العشوائية للنمذجة والتنبؤ بالأنظمة الديناميكية المعقدة: عدم اليقين الكمي، وتقدير الدولة، ونماذج الترتيب المخفض مقدمة: في المشهد التكنولوجي سريع التطور اليوم، من الأهمية بمكان فهم عملية تطوير التكنولوجيا وتأثيرها على البشرية. يقدم كتاب «الأساليب العشوائية للنمذجة والتنبؤ بالأنظمة الديناميكية المعقدة» دليلاً شاملاً لنمذجة الأنظمة المعقدة والتنبؤ بها باستخدام الأدوات العشوائية، مع التأكيد على أهمية تطوير نموذج شخصي لتصور العملية التكنولوجية لتطوير المعرفة الحديثة. يعد هذا الكتاب مصدرًا مهمًا للباحثين والممارسين الذين يسعون إلى فهم الأنظمة الديناميكية المعقدة والتنبؤ بها، خاصة تلك التي تعاني من عدم اليقين والتعقيد. الفصل 1: مقدمة للطرق العشوائية يقدم الفصل الأول مفاهيم أساسية للطرق العشوائية لنمذجة النظم الديناميكية المعقدة والتنبؤ بها. يؤكد المؤلف على الحاجة إلى الموازنة بين الكفاءة الحسابية ودقة النمذجة، وتزويد القراء بأفكار عملية لبناء نماذج مفيدة. يغطي الفصل أساسيات العمليات العشوائية وتوزيع الاحتمالات والاستدلال الإحصائي، مما يضع الأساس للفصول اللاحقة. يبحث هذا الفصل التفاعل بين نهجي النمذجة النوعية والكمية، ويبين أهمية الجمع بين النهجين لتحقيق تنبؤات دقيقة. يناقش المؤلف أهمية الحدس المادي والتفكير في فهم الأنظمة المعقدة، مع التأكيد على الحاجة إلى نهج متكامل يدمج النظريات الرياضية والعلوم الحسابية وعلوم البيانات.
