
BOOKS - Machine Learning Support for Fault Diagnosis of System-on-Chip

Machine Learning Support for Fault Diagnosis of System-on-Chip
Author: Patrick Girard
Year: March 14, 2023
Format: PDF
File size: PDF 43 MB
Language: English
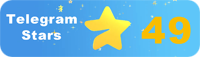
Year: March 14, 2023
Format: PDF
File size: PDF 43 MB
Language: English
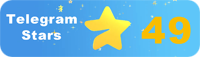
Machine Learning Support for Fault Diagnosis of System-on-Chip Introduction: In today's fast-paced technological world, it is essential to stay up-to-date with the latest advancements in Machine Learning (ML) and its applications in various fields. One such application is in the diagnosis of faults in electronic circuits and systems, which has become a critical aspect of ensuring the reliability and efficiency of modern technology. In their book, "Machine Learning Support for Fault Diagnosis of System-on-Chip the authors provide a comprehensive guide to the use of ML-based techniques for diagnosing failures in electronic systems, highlighting their potential to revolutionize the field of electronics manufacturing and design. This review will delve into the key aspects of the book and explore how it can benefit readers. Background on Fault Diagnosis and Machine Learning: Before diving into the details of the book, it is important to understand the significance of fault diagnosis in electronic systems and the role that ML plays in this process. Fault diagnosis refers to the identification and isolation of defects or malfunctions in electronic circuits and systems, which can be caused by various factors such as manufacturing defects, wear and tear, or environmental conditions. Traditional methods of fault diagnosis often rely on manual testing and inspection, which can be time-consuming and costly.
Machine arning Support for Fault Diagnosis of System-on-Chip Введение: В современном быстро развивающемся технологическом мире важно быть в курсе последних достижений в области машинного обучения (ML) и его приложений в различных областях. Одним из таких применений является диагностика неисправностей в электронных схемах и системах, что стало критическим аспектом обеспечения надежности и эффективности современных технологий. В своей книге «Machine arning Support for Fault Diagnosis of System-on-Chip» авторы дают исчерпывающее руководство по использованию ML-основанных техник для диагностики отказов в электронных системах, подчеркивая их потенциал, чтобы совершить революцию в области производства и проектирования электроники. Этот обзор углубится в ключевые аспекты книги и изучит, как она может принести пользу читателям. Справочная информация по диагностике неисправностей и машинному обучению. Прежде чем углубляться в детали книги, важно понять значение диагностики неисправностей в электронных системах и роль, которую ML играет в этом процессе. Диагностика неисправностей относится к выявлению и изоляции дефектов или неисправностей в электронных схемах и системах, которые могут быть вызваны различными факторами, такими как производственные дефекты, износ или условия окружающей среды. Традиционные методы диагностики неисправностей часто основаны на ручном тестировании и проверке, которые могут быть трудоемкими и дорогостоящими.
Machine arning Support for Fault Diagnossis of System-on-Chip Introduction : Dans le monde technologique en évolution rapide d'aujourd'hui, il est important de se tenir au courant des dernières avancées dans le domaine de l'apprentissage automatique (ML) et de ses applications dans divers domaines. L'une de ces applications est le diagnostic des défauts dans les circuits et systèmes électroniques, qui est devenu un aspect critique de la fiabilité et de l'efficacité des technologies modernes. Dans leur livre « Machine arning Support for Fault Diagnossis of System-on-Chip », les auteurs fournissent un guide complet sur l'utilisation de techniques basées sur ML pour diagnostiquer les défaillances dans les systèmes électroniques, soulignant leur potentiel pour révolutionner la production et la conception de l'électronique. Cet examen va approfondir les aspects clés du livre et examiner comment il peut être bénéfique pour les lecteurs. Informations générales sur le diagnostic des défauts et l'apprentissage automatique. Avant d'approfondir les détails du livre, il est important de comprendre l'importance du diagnostic des défauts dans les systèmes électroniques et le rôle que le ML joue dans ce processus. diagnostic des défauts se réfère à la détection et à l'isolement de défauts ou de défauts dans les circuits et systèmes électroniques qui peuvent être causés par différents facteurs tels que les défauts de fabrication, l'usure ou les conditions environnementales. s méthodes traditionnelles de diagnostic des défauts sont souvent basées sur des tests et des contrôles manuels qui peuvent être longs et coûteux.
Soporte Técnico de Máquina para Diagnóstico de Fallas del stema-en-Chip Introducción: En el mundo tecnológico en rápida evolución de hoy en día, es importante estar al tanto de los últimos avances en el aprendizaje automático (ML) y sus aplicaciones en diversos campos. Una de estas aplicaciones es el diagnóstico de fallas en circuitos y sistemas electrónicos, que se ha convertido en un aspecto crítico para garantizar la fiabilidad y eficiencia de las tecnologías modernas. En su libro «Machine Arning Support for Fault Diagnostics of System-on-Chip», los autores proporcionan una guía exhaustiva sobre el uso de técnicas basadas en ML para diagnosticar fallas en sistemas electrónicos, destacando su potencial para revolucionar la fabricación y el diseño de electrónica. Esta revisión profundizará en aspectos clave del libro y explorará cómo puede beneficiar a los lectores. Información de referencia sobre diagnóstico de fallas y aprendizaje automático. Antes de profundizar en los detalles del libro, es importante comprender el significado del diagnóstico de fallas en los sistemas electrónicos y el papel que juega ML en este proceso. diagnóstico de fallas se refiere a la detección y aislamiento de defectos o fallas en circuitos y sistemas electrónicos que pueden ser causados por diversos factores como defectos de producción, desgaste o condiciones ambientales. métodos tradicionales de diagnóstico de fallas a menudo se basan en pruebas y validaciones manuales que pueden ser laboriosas y costosas.
Máquina arning Service for Fault Diagnoses of System-on-Chip Introdução: No mundo tecnológico em rápido desenvolvimento, é importante estar informado sobre os avanços recentes na aprendizagem de máquinas (ML) e suas aplicações em várias áreas. Uma dessas aplicações é o diagnóstico de falhas nos circuitos e sistemas eletrônicos, o que se tornou um aspecto crítico para garantir a confiabilidade e a eficiência das tecnologias modernas. Em seu livro «Machine arning Suporte for Fault Diagnoses of System-on-Chip», os autores fornecem uma guia abrangente sobre o uso de técnicas baseadas em ML para diagnosticar falhas em sistemas eletrônicos, enfatizando seu potencial para revolucionar a produção e a engenharia de eletrônicos. Esta revisão vai aprofundar-se nos principais aspectos do livro e estudar como ele pode beneficiar os leitores. Informações de referência sobre diagnóstico de problemas e treinamento de máquina. Antes de se aprofundar nos detalhes do livro, é importante compreender o significado do diagnóstico de falhas nos sistemas eletrônicos e o papel que a ML desempenha neste processo. O diagnóstico de problemas refere-se à detecção e isolamento de defeitos ou falhas nos circuitos eletrônicos e sistemas que podem ser causados por vários fatores, tais como defeitos de produção, desgaste ou condições ambientais. Os métodos tradicionais de diagnóstico de falhas são muitas vezes baseados em testes manuais e verificação, que podem ser trabalhosos e dispendiosos.
Buchbesprechung: Machine arning Support for Fault Diagnose of System-on-Chip Einleitung: In der heutigen schnelllebigen technologischen Welt ist es wichtig, über die neuesten Fortschritte im Bereich des maschinellen rnens (ML) und seiner Anwendungen in verschiedenen Bereichen auf dem Laufenden zu bleiben. Eine dieser Anwendungen ist die Fehlerdiagnose in elektronischen Schaltungen und Systemen, die zu einem kritischen Aspekt geworden ist, um die Zuverlässigkeit und Effizienz moderner Technologien zu gewährleisten. In ihrem Buch „Machine arning Support for Fault Diagnosis of System-on-Chip“ geben die Autoren eine umfassende Anleitung zur Verwendung von ML-basierten Techniken zur Fehlerdiagnose in elektronischen Systemen und betonen ihr Potenzial, die Elektronikfertigung und das Design zu revolutionieren. Diese Rezension wird sich mit den wichtigsten Aspekten des Buches befassen und untersuchen, wie es den sern zugute kommen kann. Hintergrundinformationen zur Fehlerdiagnose und zum maschinellen rnen. Bevor e auf die Details des Buches eingehen, ist es wichtig, die Bedeutung der Fehlerdiagnose in elektronischen Systemen und die Rolle, die ML in diesem Prozess spielt, zu verstehen. Fehlerdiagnose bezieht sich auf die Identifizierung und Isolierung von Defekten oder Fehlern in elektronischen Schaltungen und Systemen, die durch verschiedene Faktoren wie Produktionsfehler, Verschleiß oder Umweltbedingungen verursacht werden können. Herkömmliche Fehlerdiagnosemethoden basieren oft auf manuellen Tests und Inspektionen, die zeitaufwendig und teuer sein können.
Recenzja książki: Machine arning Support for Fault Diagnosis of System-on-Chip Wprowadzenie: W dzisiejszym szybko rozwijającym się świecie technologicznym, ważne jest, aby pamiętać o najnowszych osiągnięciach w nauce maszyn (ML) i jego zastosowaniach w różnych dziedzinach. Jedną z takich aplikacji jest diagnostyka błędów w obwodach i systemach elektronicznych, która stała się krytycznym aspektem zapewnienia niezawodności i efektywności nowoczesnej technologii. W książce „Machine arning Support for Fault Diagnosis of System-on-Chip” autorzy przedstawiają kompleksowy przewodnik po wykorzystaniu technik opartych na ML do diagnozowania awarii w systemach elektronicznych, podkreślając ich potencjał do rewolucjonizacji produkcji i projektowania elektroniki. Recenzja ta skupi się na kluczowych aspektach książki i zbada, jak może przynieść korzyści czytelnikom. Rozwiązywanie problemów i odniesienie do uczenia maszynowego. Przed zagłębieniem się w szczegóły książki, ważne jest, aby zrozumieć znaczenie rozwiązywania problemów z systemami elektronicznymi oraz rolę, jaką ML odgrywa w tym procesie. Diagnoza błędów odnosi się do identyfikacji i izolacji wad lub usterek w obwodach i systemach elektronicznych, które mogą być spowodowane przez różne czynniki, takie jak wady produkcji, zużycie lub warunki środowiskowe. Tradycyjne metody diagnozowania usterek często polegają na ręcznych testach i walidacji, które mogą być czasochłonne i drogie.
Machine arning Support for Fault Diagnose of System-on-Chip Introduction: בעולם הטכנולוגי המתפתח במהירות, חשוב להקפיד על ההתקדמות האחרונה בלימוד מכונה (ML) ועל יישומיה בתחומים שונים. יישום אחד כזה הוא אבחון פגמים במעגלים אלקטרוניים ובמערכות, שהפך להיבט קריטי בהבטחת אמינות ויעילות הטכנולוגיה המודרנית. בספרם Machine arning Support for Fault Diagnose of System-on-Chip, המחברים מספקים מדריך מקיף לשימוש בטכניקות מבוססות ML כדי לאבחן כשלים במערכות אלקטרוניות, תוך הדגשת הפוטנציאל שלהם לחולל מהפכה בייצור ובעיצוב אלקטרוניקה. סקירה זו תתעמק בהיבטים מרכזיים של הספר ותחקור כיצד הוא יכול להועיל לקוראים. צרות והפניה למידת מכונה. לפני שמתעמקים בפרטי הספר, חשוב להבין את המשמעות של הצרת מערכות אלקטרוניות ואת התפקיד שממלאת ML בתהליך זה. אבחון פגמים מתייחס לזיהוי ולבידוד של פגמים או פגמים במעגלים אלקטרוניים ובמערכות העלולות להיגרם מגורמים שונים, כגון ליקויי ייצור, שחיקה או תנאי סביבה. שיטות מסורתיות לאבחון פגמים מסתמכות בדרך כלל על בדיקות ידניות ואימות, שיכולות להיות צורכות זמן ויקרות.''
Machine arning Support for Fault Diagnosis of System-on-Chip Giriş: Günümüzün hızla gelişen teknolojik dünyasında, makine öğrenimi (ML) ve çeşitli alanlardaki uygulamalarındaki en son gelişmelerden haberdar olmak önemlidir. Böyle bir uygulama, modern teknolojinin güvenilirliğini ve verimliliğini sağlamanın kritik bir yönü haline gelen elektronik devrelerde ve sistemlerde arıza teşhisidir. "Machine arning Support for Fault Diagnosis of System-on-Chip'adlı kitaplarında, yazarlar elektronik sistemlerdeki arızaları teşhis etmek için ML tabanlı teknikleri kullanmak için kapsamlı bir rehber sunmakta ve elektronik üretiminde ve tasarımında devrim yaratma potansiyellerini vurgulamaktadır. Bu derleme, kitabın temel yönlerini inceleyecek ve okuyuculara nasıl fayda sağlayabileceğini araştıracaktır. Sorun giderme ve makine öğrenimi referansı. Kitabın ayrıntılarına girmeden önce, elektronik sistemlerde sorun gidermenin önemini ve ML'nin bu süreçte oynadığı rolü anlamak önemlidir. Arıza teşhisi, elektronik devrelerde ve sistemlerde, üretim kusurları, aşınma veya çevre koşulları gibi çeşitli faktörlerin neden olabileceği kusurların veya arızaların tanımlanması ve izolasyonu anlamına gelir. Hataları teşhis etmek için geleneksel yöntemler genellikle zaman alıcı ve pahalı olabilen manuel test ve doğrulamaya dayanır.
مراجعة الكتاب |: دعم التعلم الآلي لتشخيص الأخطاء في مقدمة النظام على الرقاقة: في العالم التكنولوجي سريع التطور اليوم، من المهم مواكبة أحدث التطورات في التعلم الآلي (ML) وتطبيقاته في مختلف المجالات. أحد هذه التطبيقات هو تشخيص الأخطاء في الدوائر والأنظمة الإلكترونية، والذي أصبح جانبًا حاسمًا لضمان موثوقية وكفاءة التكنولوجيا الحديثة. في كتابهم «دعم التعلم الآلي لتشخيص الأخطاء في النظام على الرقاقة»، يقدم المؤلفون دليلًا شاملاً لاستخدام التقنيات القائمة على ML لتشخيص الإخفاقات في الأنظمة الإلكترونية، مما يسلط الضوء على قدرتها على إحداث ثورة في تصنيع وتصميم الإلكترونيات. ستبحث هذه المراجعة في الجوانب الرئيسية للكتاب وتستكشف كيف يمكن أن يفيد القراء. استكشاف الأخطاء وإشارة التعلم الآلي. قبل الخوض في تفاصيل الكتاب، من المهم فهم أهمية استكشاف الأخطاء والأنظمة الإلكترونية والدور الذي تلعبه ML في هذه العملية. يشير تشخيص الأخطاء إلى تحديد وعزل العيوب أو العيوب في الدوائر والأنظمة الإلكترونية التي قد تكون ناجمة عن عوامل مختلفة، مثل عيوب التصنيع أو التآكل أو الظروف البيئية. غالبًا ما تعتمد الطرق التقليدية لتشخيص العيوب على الاختبار اليدوي والتحقق من الصحة، والذي يمكن أن يستغرق وقتًا طويلاً ومكلفًا.
도서 검토: System-on-Chip 소개의 결함 진단을위한 기계 학습 지원: 오늘날의 빠르게 진화하는 기술 세계에서 기계 학습 (ML) 및 다양한 분야의 응용 프로그램의 최신 발전을 유지하는 것이 중요합니다. 이러한 응용 분야 중 하나는 전자 회로 및 시스템의 결함 진단으로, 현대 기술의 신뢰성과 효율성을 보장하는 데 중요한 측면이되었습니다. 저자 "System-on-Chip의 결함 진단을위한 기계 학습 지원" 에서 저자는 ML 기반 기술을 사용하여 전자 시스템의 고장을 진단하여 전자 제품 제조 및 설계에 혁명을 일으킬 수있는 잠재력을 강조하는 포괄적 인 가이드를 제공합니다. 이 검토는 책의 주요 측면을 탐구하고 독자에게 어떻게 혜택을 줄 수 있는지 탐구합니다. 문제 해결 및 기계 학습 참조. 이 책의 세부 사항을 조사하기 전에 전자 시스템 문제 해결의 중요성과이 과정에서 ML의 역할을 이해하는 것이 중요합니다. 결함 진단은 제조 결함, 마모 또는 환경 조건과 같은 다양한 요인으로 인해 발생할 수있는 전자 회로 및 시스템에서 결함 또는 결함의 식별 및 격리를 말합니다. 결함을 진단하는 전통적인 방법은 종종 시간이 많이 걸리고 비쌀 수있는 수동 테스트 및 검증에 의존합니다.
Book Review:システムオンチップの故障診断のための機械学習サポートはじめに:今日急速に進化している技術の世界では、機械学習(ML)の最新の進歩と様々な分野のアプリケーションを常に把握することが重要です。このようなアプリケーションの1つは、電子回路やシステムにおける障害診断です。これは、現代の技術の信頼性と効率性を確保するための重要な側面となっています。著者らは著書「System-on-Chipの故障診断のための機械学習サポート」で、電子システムの故障を診断するためにMLベースの技術を使用するための包括的なガイドを提供し、電子機器の製造と設計に革命を起こす可能性を強調している。このレビューでは、本の主要な側面を掘り下げ、読者にどのように利益をもたらすかを検討します。トラブルシューティングと機械学習の参照。本の詳細を掘り下げる前に、電子システムのトラブルシューティングの重要性と、このプロセスでMLが果たす役割を理解することが重要です。故障診断とは、製造上の欠陥、摩耗、環境条件など、さまざまな要因によって引き起こされる可能性のある電子回路やシステムの欠陥または欠陥の識別と分離を指します。欠陥を診断するための従来の方法は、多くの場合、手動テストと検証に依存しています。
Book Review:針對芯片上系統故障診斷的機器學習支持介紹:在當今快速發展的技術世界中,跟上機器學習(ML)及其在各個領域的應用的最新進展非常重要。其中一種應用是電子電路和系統故障診斷,這已成為確保現代技術可靠性和有效性的關鍵方面。在他們的著作《芯片上系統故障診斷的機器學習支持》中,作者為使用ML基礎技術診斷電子系統故障提供了詳盡的指導,強調了他們在電子制造和設計領域進行革命的潛力。這篇評論將深入研究這本書的關鍵方面,並探討它如何使讀者受益。故障診斷和機器學習背景。在深入研究本書的細節之前,重要的是要了解電子系統故障診斷的重要性以及ML在此過程中發揮的作用。故障診斷是指檢測和隔離電子電路和系統中可能由各種因素(例如制造缺陷,磨損或環境條件)引起的缺陷或故障。傳統的故障診斷方法通常基於手動測試和驗證,這可能是耗時且昂貴的。
