
BOOKS - Metaheuristics for Enterprise Data Intelligence

Metaheuristics for Enterprise Data Intelligence
Author: Kaustubh Vaman Sakhare, Vibha Vyas, Apoorva S Shastri
Year: 2025
Pages: 159
Format: PDF
File size: 10.1 MB
Language: ENG
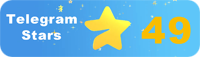
Year: 2025
Pages: 159
Format: PDF
File size: 10.1 MB
Language: ENG
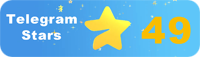
Metaheuristics for Enterprise Data Intelligence: Unlocking the Power of Data-Driven Decision Making In today's digital age, information has become an integral part of business excellence, and every enterprise, regardless of its domain of interest, generates and processes vast amounts of data in their day-to-day activities. However, converting these massive datasets into insightful information remains a significant challenge for organizations. The sheer volume and complexity of data make it difficult to analyze and draw meaningful conclusions, making data intelligence and its analysis crucial for developing better business solutions. The Emergence of the Data Economy With the emergence of the data economy, the amount of data being generated is unprecedented, and this trend is expected to continue in the future. As a result, enterprises need to adapt to this new reality by leveraging data intelligence and analytics to gain a competitive edge. The ability to process, analyze, and interpret large datasets is becoming increasingly important for businesses to remain relevant and achieve their goals. Challenges in Data Representation One of the primary challenges in data representation is building knowledge systems that can handle diverse data types and sources. This requires the development of sophisticated algorithms and techniques to manage and analyze the data effectively. Moreover, resolving issues related to data quality, accuracy, and reliability is essential to ensure that the insights gained from data analysis are reliable and actionable. The Need for Metaheuristics To overcome these challenges, metaheuristics have become an essential tool for optimizing data-driven decision-making processes.
Метаэвристика для корпоративной аналитики данных: раскрытие возможностей принятия решений на основе данных В современную цифровую эпоху информация стала неотъемлемой частью совершенства бизнеса, и каждое предприятие, независимо от области его интересов, генерирует и обрабатывает огромные объемы данных в своей повседневной деятельности. Однако преобразование этих массивных наборов данных в проницательную информацию остается серьезной проблемой для организаций. Огромный объем и сложность данных затрудняют анализ и вывод значимых выводов, что делает интеллектуальный анализ данных и его анализ критически важными для разработки более эффективных бизнес-решений. Появление экономики данных С появлением экономики данных объем генерируемых данных беспрецедентен, и ожидается, что эта тенденция сохранится в будущем. В результате предприятия должны адаптироваться к этой новой реальности, используя интеллектуальный анализ данных и аналитику для получения конкурентного преимущества. Способность обрабатывать, анализировать и интерпретировать большие наборы данных становится все более важной для бизнеса, чтобы оставаться актуальными и достигать своих целей. Проблемы в представлении данных Одной из основных проблем в представлении данных является создание систем знаний, которые могут обрабатывать различные типы данных и источники. Это требует разработки сложных алгоритмов и методов для эффективного управления и анализа данных. Кроме того, решение проблем, связанных с качеством, точностью и надежностью данных, имеет важное значение для обеспечения надежности и действенности результатов анализа данных. Потребность в метаэвристике Чтобы преодолеть эти проблемы, метаэвристика стала важным инструментом для оптимизации процессов принятия решений на основе данных.
Metavristica for Corporate Data Analysis : Découvrez les possibilités de prise de décisions basées sur les données À l'ère numérique moderne, l'information est devenue une partie intégrante de l'excellence de l'entreprise, et chaque entreprise, quel que soit son domaine d'intérêt, génère et traite d'énormes quantités de données dans ses activités quotidiennes. Toutefois, la conversion de ces ensembles de données massifs en informations perspicaces demeure un défi majeur pour les organisations. La grande quantité et la complexité des données rendent difficile l'analyse et la conclusion de résultats significatifs, ce qui rend l'exploration et l'analyse des données essentielles pour développer de meilleures solutions commerciales. L'émergence de l'économie des données Avec l'émergence de l'économie des données, le volume de données générées est sans précédent et cette tendance devrait se poursuivre à l'avenir. En conséquence, les entreprises doivent s'adapter à cette nouvelle réalité en utilisant l'exploration de données et l'analyse pour obtenir un avantage concurrentiel. La capacité de traiter, d'analyser et d'interpréter de grands ensembles de données devient de plus en plus importante pour les entreprises afin de rester pertinentes et d'atteindre leurs objectifs. Problèmes dans la présentation des données L'un des principaux problèmes dans la présentation des données est la création de systèmes de connaissances qui peuvent traiter différents types de données et sources. Cela nécessite le développement d'algorithmes et de méthodes complexes pour une gestion et une analyse efficaces des données. En outre, la résolution des problèmes de qualité, de précision et de fiabilité des données est essentielle pour garantir la fiabilité et l'efficacité des résultats de l'analyse des données. Besoin de métavrisme Pour surmonter ces défis, la métavristique est devenue un outil essentiel pour optimiser les processus décisionnels fondés sur les données.
Metaevrística para la analítica de datos corporativos: exponer las capacidades de toma de decisiones basadas en datos En la era digital actual, la información se ha convertido en una parte integral de la excelencia empresarial, y cada empresa, independientemente de su área de interés, genera y procesa enormes cantidades de datos en sus operaciones diarias. n embargo, convertir estos conjuntos de datos masivos en información perspicaz sigue siendo un gran desafío para las organizaciones. La enorme cantidad y complejidad de los datos hace que sea difícil analizar y extraer conclusiones significativas, lo que hace que la minería de datos y su análisis sean cruciales para desarrollar soluciones empresariales más eficientes. Aparición de la economía de datos Con la aparición de la economía de datos, la cantidad de datos generados no tiene precedentes y se espera que esta tendencia continúe en el futuro. Como resultado, las empresas deben adaptarse a esta nueva realidad, utilizando la minería de datos y la analítica para obtener una ventaja competitiva. La capacidad de procesar, analizar e interpretar grandes conjuntos de datos es cada vez más importante para que las empresas permanezcan actualizadas y alcancen sus objetivos. Problemas en la presentación de datos Uno de los principales problemas en la presentación de datos es la creación de sistemas de conocimiento que pueden manejar diferentes tipos de datos y fuentes. Esto requiere el desarrollo de sofisticados algoritmos y métodos para gestionar y analizar los datos de manera eficiente. Además, la solución de los problemas relacionados con la calidad, la exactitud y la fiabilidad de los datos es esencial para garantizar la fiabilidad y la eficacia de los resultados del análisis de datos. Necesidad de metaevrística Para superar estos desafíos, la metahevrística se ha convertido en una herramienta importante para optimizar los procesos de toma de decisiones basados en datos.
Meta-Auristik für Enterprise Data Analytics: Die Möglichkeiten datenbasierter Entscheidungsfindung erschließen Im heutigen digitalen Zeitalter sind Informationen zu einem integralen Bestandteil der Business Excellence geworden und jedes Unternehmen, unabhängig von seinem Interessengebiet, generiert und verarbeitet im Tagesgeschäft enorme Datenmengen. Die Umwandlung dieser massiven Datensätze in aufschlussreiche Informationen bleibt jedoch eine große Herausforderung für Unternehmen. Die schiere Menge und Komplexität der Daten macht es schwierig, aussagekräftige Schlussfolgerungen zu analysieren und zu ziehen, was Data Mining und seine Analyse entscheidend für die Entwicklung effizienterer Geschäftslösungen macht. Die Entstehung der Datenökonomie Mit dem Aufkommen der Datenökonomie ist die Menge der erzeugten Daten beispiellos, und es wird erwartet, dass sich dieser Trend in Zukunft fortsetzen wird. Infolgedessen müssen sich Unternehmen an diese neue Realität anpassen, indem sie Data Mining und Analytics nutzen, um Wettbewerbsvorteile zu erzielen. Die Fähigkeit, große Datensätze zu verarbeiten, zu analysieren und zu interpretieren, wird für Unternehmen immer wichtiger, um relevant zu bleiben und ihre Ziele zu erreichen. Herausforderungen in der Datenansicht Eine der größten Herausforderungen in der Datenansicht ist die Schaffung von Wissenssystemen, die verschiedene Arten von Daten und Quellen verarbeiten können. Dies erfordert die Entwicklung komplexer Algorithmen und Methoden zur effizienten Verwaltung und Analyse von Daten. Darüber hinaus ist die Lösung von Problemen in Bezug auf Datenqualität, Genauigkeit und Zuverlässigkeit unerlässlich, um die Zuverlässigkeit und Wirksamkeit der Datenanalyseergebnisse sicherzustellen. Die Notwendigkeit der Meta-Auristik Um diese Herausforderungen zu meistern, ist die Meta-Auristik zu einem wichtigen Instrument für die Optimierung datenbasierter Entscheidungsprozesse geworden.
''
Kurumsal Veri Analitiği için Metaheuristics: Veriye Dayalı Karar Vermenin Kilidini Açma Günümüzün dijital çağında, bilgi iş mükemmelliğinin ayrılmaz bir parçası haline geldi ve her işletme, ilgi alanı ne olursa olsun, günlük operasyonlarında büyük miktarda veri üretiyor ve işliyor. Bununla birlikte, bu büyük veri kümelerini anlayışlı bilgilere dönüştürmek, kuruluşlar için büyük bir zorluk olmaya devam etmektedir. Verilerin hacmi ve karmaşıklığı, anlamlı sonuçların analiz edilmesini ve çıkarılmasını zorlaştırır ve veri madenciliğini ve analizini daha iyi iş çözümleri geliştirmek için kritik hale getirir. Veri ekonomisinin ortaya çıkışı Veri ekonomisinin ortaya çıkmasıyla birlikte, üretilen veri hacminin eşi benzeri görülmemiştir ve bu eğilimin gelecekte de devam etmesi beklenmektedir. Sonuç olarak, işletmeler rekabet avantajı elde etmek için veri madenciliği ve analitik kullanarak bu yeni gerçekliğe uyum sağlamalıdır. Büyük veri setlerini işleme, analiz etme ve yorumlama yeteneği, işletmelerin alakalı kalmaları ve hedeflerine ulaşmaları için giderek daha önemli hale gelmektedir. Veri Sunumundaki Sorunlar Veri sunumundaki temel sorunlardan biri, farklı veri ve kaynak türlerini işleyebilecek bilgi sistemlerinin oluşturulmasıdır. Bu, verileri verimli bir şekilde yönetmek ve analiz etmek için sofistike algoritmaların ve yöntemlerin geliştirilmesini gerektirir. Ayrıca, veri kalitesi, doğruluğu ve güvenilirliği konularının ele alınması, veri analizi sonuçlarının güvenilirliğini ve etkinliğini sağlamak için gereklidir. Metaheuristik ihtiyacı Bu zorlukların üstesinden gelmek için, metaheuristik veri odaklı karar verme süreçlerini optimize etmek için önemli bir araç haline gelmiştir.
Metahuritics for Enterprise Data Analytics: فتح صنع القرار القائم على البيانات في العصر الرقمي اليوم، أصبحت المعلومات جزءًا لا يتجزأ من التميز التجاري، وكل مؤسسة، بغض النظر عن مجال اهتمامها، تولد وتعالج كميات هائلة من البيانات في عملياتها اليومية. ومع ذلك، فإن ترجمة مجموعات البيانات الضخمة هذه إلى معلومات ثاقبة لا تزال تمثل تحديًا كبيرًا للمنظمات. يجعل الحجم والتعقيد الهائل للبيانات من الصعب تحليل واستخلاص استنتاجات ذات مغزى، مما يجعل تعدين البيانات وتحليلها أمرًا بالغ الأهمية لتطوير حلول تجارية أفضل. مع ظهور اقتصاد البيانات، يكون حجم البيانات المتولدة غير مسبوق، ومن المتوقع أن يستمر هذا الاتجاه في المستقبل. نتيجة لذلك، يجب على الشركات التكيف مع هذا الواقع الجديد باستخدام التنقيب عن البيانات والتحليلات للحصول على ميزة تنافسية. تزداد أهمية القدرة على معالجة مجموعات البيانات الكبيرة وتحليلها وتفسيرها بالنسبة للشركات للبقاء على صلة وتحقيق أهدافها. تتمثل إحدى المشاكل الرئيسية في عرض البيانات في إنشاء نظم معرفية يمكنها معالجة مختلف أنواع البيانات والمصادر. وهذا يتطلب تطوير خوارزميات وأساليب متطورة لإدارة البيانات وتحليلها بكفاءة. وبالإضافة إلى ذلك، فإن معالجة مسائل جودة البيانات ودقتها وموثوقيتها أمر أساسي لضمان موثوقية وفعالية نتائج تحليل البيانات. للتغلب على هذه التحديات، أصبحت الميتاهيروسيكا أداة مهمة لتحسين عمليات صنع القرار القائمة على البيانات.
