
BOOKS - OS AND DB - Statistical Methods for Recommender Systems

Statistical Methods for Recommender Systems
Author: Deepak K. Agarwal, Bee-Chung Chen
Year: 2016
Pages: 298
Format: PDF
File size: 6,6 MB
Language: ENG
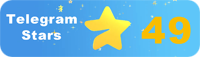
Year: 2016
Pages: 298
Format: PDF
File size: 6,6 MB
Language: ENG
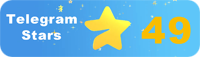
''
