
BOOKS - EQUIPMENT - Embedded Machine Learning for Cyber-Physical, IoT, and Edge Compu...

Embedded Machine Learning for Cyber-Physical, IoT, and Edge Computing Use Cases and Emerging Challenges
Author: Sudeep Pasricha, Muhammad Shafique
Year: 2024
Pages: 571
Format: PDF
File size: 28.9 MB
Language: ENG
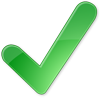
Year: 2024
Pages: 571
Format: PDF
File size: 28.9 MB
Language: ENG
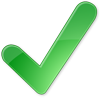
Book Embedded Machine Learning for Cyber-Physical IoT and Edge Computing - Use Cases and Emerging Challenges Introduction: In the ever-evolving world of technology, machine learning (ML) has emerged as a prominent approach for achieving state-of-the-art accuracy in various data analytic applications. From computer vision, speech recognition, healthcare diagnostics, robotics, and autonomous vehicles to business and financial analysis, the driving force behind the success of ML is the advent of neural network (NN) algorithms such as deep neural networks (DNNs), deep learning (DL), and spiking neural networks (SNNs). With the support of today's evolving computing landscape, ML accelerators have enabled better exploitation of data and thread-level parallelism, leading to more powerful abilities for solving ML tasks using embedded systems with limited compute and memory resources. This book provides a comprehensive overview of the current state of the art in embedded ML techniques and their applications in various domains, covering both the theoretical foundations and practical challenges of applying ML to resource-constrained devices. Chapter 1: The Evolution of Machine Learning The chapter begins by exploring the need to study and understand the process of technological evolution.
Book Embedded Machine arning for Cyber-Physical IoT and Edge Computing - Use Cases and Emerging Challenges Введение: В постоянно развивающемся мире технологий машинное обучение (ML) стало выдающимся подходом для достижения современной точности в различных приложениях для анализа данных. От компьютерного зрения, распознавания речи, диагностики здравоохранения, робототехники и автономных транспортных средств до делового и финансового анализа, движущей силой успеха ML является появление алгоритмов нейронных сетей (NN), таких как глубокие нейронные сети (DNN), глубокое обучение (DL) и пиковые нейронные сети (SNN). Благодаря поддержке современного развивающегося компьютерного ландшафта ускорители ML позволили лучше использовать параллелизм на уровне данных и потоков, что привело к более мощным возможностям решения задач ML с использованием встроенных систем с ограниченными вычислительными ресурсами и ресурсами памяти. В этой книге представлен всесторонний обзор современного состояния встроенных методов ML и их применения в различных областях, охватывающий как теоретические основы, так и практические проблемы применения ML к устройствам с ограниченными ресурсами. Глава 1: Эволюция машинного обучения Глава начинается с изучения необходимости изучения и понимания процесса технологической эволюции.
Book Embedded Machine arning for Cyber-Physical IoT and Edge Computing - Use Cases and Emerging Challenges Introduction : Dans un monde en constante évolution, la technologie de l'apprentissage automatique (ML) est devenue une approche exceptionnelle pour atteindre la précision moderne dans diverses applications d'analyse de données. De la vision par ordinateur, de la reconnaissance vocale, du diagnostic des soins de santé, de la robotique et des véhicules autonomes à l'analyse commerciale et financière, le moteur du succès de ML est l'émergence d'algorithmes de réseaux neuronaux (NN) tels que les réseaux neuronaux profonds (DNN), l'apprentissage profond (DL) et les réseaux neuronaux de pointe (SNN). Grâce au soutien d'un paysage informatique en évolution moderne, les accélérateurs ML ont permis de mieux exploiter le parallélisme au niveau des données et des flux, ce qui a conduit à des capacités plus puissantes pour résoudre les problèmes ML en utilisant des systèmes intégrés avec des ressources de calcul et de mémoire limitées. Ce livre présente un aperçu complet de l'état actuel des techniques de ML intégrées et de leurs applications dans différents domaines, couvrant à la fois les bases théoriques et les problèmes pratiques de l'application de ML aux appareils à ressources limitées. Chapitre 1 : L'évolution de l'apprentissage automatique chapitre commence par étudier la nécessité d'étudier et de comprendre le processus d'évolution technologique.
Book Embedded Machine arning for Cyber-Physical IoT and Edge Computing - Use Cases and Emerging Challenges Introducción: En un mundo de tecnología en constante evolución, el aprendizaje automático (ML) se ha convertido en un enfoque sobresaliente para lograr una precisión moderna en diferentes aplicaciones de análisis de datos. Desde la visión informática, el reconocimiento del habla, el diagnóstico sanitario, la robótica y los vehículos autónomos hasta el análisis empresarial y financiero, la fuerza motriz del éxito de ML es la aparición de algoritmos de redes neuronales (NN) como redes neuronales profundas (DNN), aprendizaje profundo (DL) y redes neuronales de pico (SNN) Gracias al soporte del panorama informático en evolución de hoy en día, los aceleradores ML han permitido un mejor uso del paralelismo a nivel de datos y subprocesos, lo que ha dado lugar a capacidades más potentes para resolver problemas ML utilizando sistemas integrados con recursos informáticos y de memoria limitados. Este libro ofrece una visión general completa del estado actual de las técnicas de ML incorporadas y sus aplicaciones en diferentes campos, cubriendo tanto los fundamentos teóricos como los problemas prácticos de la aplicación de ML a dispositivos con recursos limitados. Capítulo 1: Evolución del aprendizaje automático capítulo comienza con el estudio de la necesidad de estudiar y comprender el proceso de evolución tecnológica.
Book Embedded Machine arning for Cyber-Fisical IoT and Edge Computing - Use Cases and Emerging Challenges Introdução: No mundo em constante evolução da tecnologia, o aprendizado de máquinas (ML) se tornou uma abordagem extraordinária para alcançar a precisão moderna em várias aplicações de análise de dados. Desde visão de computador, reconhecimento de voz, diagnóstico de saúde, robótica e veículos autônomos até análise de negócios e finanças, o motor do sucesso da ML é o surgimento de algoritmos de redes neurais (NN), tais como redes neurais profundas (DNN), treinamento profundo (DL) e redes neurais de pico (SNN). Com o suporte da paisagem moderna em desenvolvimento do computador, os aceleradores ML permitiram uma melhor utilização do paralelismo ao nível dos dados e dos fluxos, resultando em uma capacidade mais poderosa para lidar com as tarefas ML usando sistemas integrados com recursos de processamento e memória limitados. Este livro apresenta uma revisão completa do estado atual dos métodos de ML incorporados e suas aplicações em várias áreas, que abrange tanto os fundamentos teóricos quanto os problemas práticos da aplicação da ML a dispositivos com recursos limitados. Capítulo 1: Evolução da aprendizagem de máquinas O capítulo começa com o estudo da necessidade de estudar e compreender o processo de evolução tecnológica.
Book Embedded Machine arning for Cyber- IoT ical ed Edge Computing - Use Cases and Emerging Challenges Introduzione: In un mondo in continua evoluzione della tecnologia, l'apprendimento automatico (ML) è stato un approccio eccezionale per ottenere l'accuratezza moderna in diverse applicazioni di analisi dei dati. Dalla visione informatica, al riconoscimento vocale, alla diagnosi sanitaria, alla robotica e ai veicoli autonomi fino all'analisi aziendale e finanziaria, il motore del successo di ML è la nascita di algoritmi di rete neurale (NN), quali reti neurali profonde (DNN), formazione profonda (DL) e reti neurali di picco (SNN). Grazie al supporto di un panorama informatico in continua evoluzione, gli acceleratori ML sono stati in grado di sfruttare al meglio il parallelismo a livello di dati e di thread, con la conseguente maggiore capacità di risolvere le sfide ML utilizzando sistemi integrati con risorse di elaborazione e memoria limitate. Questo libro fornisce una panoramica completa dello stato attuale dei metodi ML incorporati e della loro applicazione in diversi ambiti, che comprende sia le basi teoriche che i problemi pratici relativi all'applicazione di ML ai dispositivi con risorse limitate. Capitolo 1: Evoluzione dell'apprendimento automatico Il capitolo inizia studiando la necessità di studiare e comprendere l'evoluzione tecnologica.
Buch Embedded Machine arning for Cyber-Physical IoT and Edge Computing - Use Cases and Emerging Challenges Einführung: In der sich ständig weiterentwickelnden Welt der Technologie hat sich Machine arning (ML) zu einem herausragenden Ansatz entwickelt, um moderne Präzision in verschiedenen Anwendungen der Datenanalyse zu erreichen. Von Computer Vision, Spracherkennung, Gesundheitsdiagnostik, Robotik und autonomen Fahrzeugen bis hin zu Geschäfts- und Finanzanalysen ist die treibende Kraft hinter dem Erfolg von ML das Aufkommen neuronaler Netzwerkalgorithmen (NN) wie Deep Neural Networks (DNN), Deep arning (DL) und Peak Neural Networks (SNN). Durch die Unterstützung der sich ständig weiterentwickelnden Computerlandschaft haben ML-Beschleuniger eine bessere Nutzung der Parallelität auf Daten- und Thread-Ebene ermöglicht, was zu leistungsfähigeren ML-Problemlösungsfähigkeiten unter Verwendung eingebetteter Systeme mit begrenzten Computer- und Speicherressourcen geführt hat. Dieses Buch bietet einen umfassenden Überblick über den aktuellen Stand der eingebetteten ML-Methoden und ihre Anwendung in verschiedenen Bereichen und deckt sowohl die theoretischen Grundlagen als auch die praktischen Herausforderungen bei der Anwendung von ML auf Geräte mit begrenzten Ressourcen ab. Kapitel 1: Die Evolution des maschinellen rnens Das Kapitel beginnt mit der Untersuchung der Notwendigkeit, den Prozess der technologischen Evolution zu studieren und zu verstehen.
Book Embedded Machine arning for Cyber-Physical IoT and Edge Computing - Use Cases and Emerging Challenges Wprowadzenie: W stale rozwijającym się świecie technologii uczenie maszynowe (ML) stało się doskonałym podejściem do osiągnięcia nowoczesnej dokładności w różnych aplikacjach do analizy danych. Od wizji komputerowej, rozpoznawania mowy, diagnostyki opieki zdrowotnej, robotyki i autonomicznych pojazdów po analizę biznesową i finansową, siłą napędową sukcesu ML jest pojawienie się algorytmów sieci neuronowej (NN), takich jak głębokie sieci neuronowe (DNN), głębokie uczenie się (DL) i szczytowe sieci neuronowe (SNN N s). Wspierając obecnie rozwijający się krajobraz obliczeniowy, akceleratory ML umożliwiły lepsze wykorzystanie równoległości na poziomie danych i nici, co skutkowało silniejszym rozwiązaniem problemów ML przy użyciu wbudowanych systemów z ograniczonymi zasobami obliczeniowymi i pamięciowymi. Książka zawiera kompleksowy przegląd aktualnego stanu wbudowanych metod ML i ich zastosowania w różnych dziedzinach, obejmujący zarówno fundamenty teoretyczne, jak i praktyczne problemy z zastosowaniem ML do urządzeń o ograniczonych zasobach. Rozdział 1: Ewolucja uczenia maszynowego Rozdział rozpoczyna się od zbadania potrzeby badania i zrozumienia procesu ewolucji technologicznej.
Book Metted Machine arning for Cyber-Physical IOTT and Edge Computing - Use Cases and Emprogress Exchange Intelligence: בעולם המתפתח של טכנולוגיה, למידת מכונה (ML) הפכה לגישה יוצאת דופן להשגת דיוק במגוון יישומים. מראייה ממוחשבת, זיהוי דיבור, אבחון בריאות, רובוטיקה וכלי רכב אוטונומיים לניתוח עסקי ופיננסי, הכוח המניע מאחורי ההצלחה של ML הוא הופעת אלגוריתמים של רשת עצבית (NN) כגון רשתות עצביות עמוקות (DNNs), למידה עמוקה (DLs) ורשתות עצביות שיא (SNs). על ידי תמיכה בנוף המחשוב המתפתח של היום, מאיצי ML אפשרו שימוש טוב יותר במקביליות ברמת נתונים ורמת חוטים, וכתוצאה מכך יכולות פתירת בעיות ML חזקות יותר באמצעות מערכות משובצות עם משאבי מחשוב וזיכרון מוגבלים. ספר זה מספק סקירה מקיפה של המצב הנוכחי של שיטות ML מובנות ויישומן בתחומים שונים, המכסה הן יסודות תאורטיים והן בעיות מעשיות של יישום ML במכשירים בעלי משאבים מוגבלים. פרק 1: האבולוציה של למידת המכונה מתחילה בבחינת הצורך ללמוד ולהבין את תהליך האבולוציה הטכנולוגית.''
Book Embedded Machine arning for Cyber-Physical IoT and Edge Computing - Use Cases and Emerging Challenges Giriş: Sürekli gelişen teknoloji dünyasında, makine öğrenimi (ML), çeşitli veri analizi uygulamalarında modern doğruluk elde etmek için olağanüstü bir yaklaşım haline gelmiştir. Bilgisayar görüşü, konuşma tanıma, sağlık teşhisi, robotik ve otonom araçlardan iş ve finansal analizlere kadar, ML'nin başarısının arkasındaki itici güç, derin sinir ağları (DNN'ler), derin öğrenme (DL'ler) ve tepe sinir ağları (SNN'ler) gibi sinir ağı (NN) algoritmalarının ortaya çıkmasıdır. Günümüzün gelişen bilgi işlem ortamını destekleyerek, ML hızlandırıcıları veri düzeyinde ve iş parçacığı düzeyinde paralelliğin daha iyi kullanılmasını sağlayarak, sınırlı bilgi işlem ve bellek kaynaklarına sahip gömülü sistemleri kullanarak daha güçlü ML problem çözme yetenekleri elde edilmesini sağlamıştır. Bu kitap, yerleşik ML yöntemlerinin mevcut durumuna ve çeşitli alanlardaki uygulamalarına, hem teorik temelleri hem de sınırlı kaynaklara sahip cihazlara ML uygulamasının pratik sorunlarını kapsayan kapsamlı bir genel bakış sunmaktadır. Bölüm 1: Makine Öğreniminin Evrimi Bölüm, teknolojik evrim sürecini inceleme ve anlama ihtiyacını inceleyerek başlar.
كتاب التعلم الآلي المضمّن لإنترنت الأشياء السيبراني الفيزيائي وحوسبة الحافة - حالات الاستخدام والتحديات الناشئة مقدمة: في عالم التكنولوجيا المتطور باستمرار، أصبح التعلم الآلي (ML) نهجًا رائعًا لتحقيق الدقة الحديثة في مجموعة متنوعة من تطبيقات تحليل البيانات. من رؤية الكمبيوتر والتعرف على الكلام وتشخيص الرعاية الصحية والروبوتات والمركبات المستقلة إلى الأعمال والتحليل المالي، فإن القوة الدافعة وراء نجاح ML هي ظهور خوارزميات الشبكة العصبية (NN) مثل الشبكات العصبية العميقة (DNNs) والتعلم العميق (DLs) وذروة الشبكات العصبية (SNNs)). من خلال دعم مشهد الحوسبة المتطور اليوم، مكنت مسرعات ML من استخدام أفضل للتوازي على مستوى البيانات والخيوط، مما أدى إلى قدرات أكثر قوة لحل مشكلات ML باستخدام أنظمة مضمنة ذات موارد حوسبة وذاكرة محدودة. يقدم هذا الكتاب لمحة عامة شاملة عن الحالة الحالية لطرق ML المدمجة وتطبيقها في مختلف المجالات، والتي تغطي الأسس النظرية والمشاكل العملية لتطبيق ML على الأجهزة ذات الموارد المحدودة. الفصل 1: تطور التعلم الآلي يبدأ الفصل بفحص الحاجة إلى دراسة وفهم عملية التطور التكنولوجي.
사이버 물리적 IoT 및 엣지 컴퓨팅을위한 예약 임베디드 머신 러닝-사용 사례 및 신흥 챌린지 소개: 끊임없이 진화하는 기술 세계에서 머신 러닝 (ML) 은 다양한 데이터 분석 응용 프로그램에서 현대적인 정확성을 달성하기위한 뛰어난 접근. 컴퓨터 비전, 음성 인식, 의료 진단, 로봇 공학 및 자율 주행 차량에서 비즈니스 및 재무 분석에 이르기까지 ML의 성공의 원동력은 심층 신경망 (DNN), 딥 러닝 (DL) 및 최고 신경망 (SNN). 오늘날의 진화하는 컴퓨팅 환경을 지원함으로써 ML 가속기는 데이터 레벨 및 스레드 레벨 병렬 처리를 더 잘 사용할 수있게되었으며, 제한된 컴퓨팅 및 메모리 리소스를 갖춘 임베디드 시스템을 사용하여보다 강력한 ML 문제 해결 기능을 제공했 이 책은 현재 내장 ML 방법의 상태와 다양한 분야에서의 적용에 대한 포괄적 인 개요를 제공하며, 이론적 기초와 자원이 제한된 장치에 ML을 적용하는 실제 문제를 모두 다룹니다. 1 장: 기계 학습의 진화 장은 기술 진화 과정을 연구하고 이해해야 할 필요성을 조사하는 것으로 시작됩니다.
Book Embedded Machine arning for Cyber-Physical IoT and Edge Computing-ユースケースと新たな課題はじめに:進化するテクノロジーの世界では、機械学習(ML)は、さまざまなデータ分析アプリケーションで現代的な精度を達成するための優れたアプローチとなっています。コンピュータビジョン、音声認識、ヘルスケア診断、ロボット、自動運転車からビジネスおよび財務分析まで、MLの成功の原動力は、ディープニューラルネットワーク(DNN)、ディープラーニング(DL)、ピークニューラルネットワーク(SNN)などのニューラルネットワーク(NN)アルゴリズムの出現です。今日の進化しているコンピューティング環境をサポートすることで、MLアクセラレータはデータレベルとスレッドレベルの並列性を向上させ、コンピューティングとメモリリソースが限られた組み込みシステムを使用して、より強力なML問題解決機能を実現しました。本書では、MLを限られたリソースを持つデバイスに適用する際の理論的基礎と実践的な問題の両方を網羅した、組み込みML手法の現状とその応用について、包括的に概説する。第1章:機械学習の進化この章は、技術進化の過程を研究し理解する必要性を検討することから始まります。
網絡物理物理IoT和邊緣計算-使用案例和新興挑戰書籍嵌入式機器學習介紹:在不斷發展的技術世界中,機器學習(ML)已成為在各種數據分析應用程序中實現現代準確性的傑出方法。從計算機視覺,語音識別,健康診斷,機器人技術和自動駕駛汽車到商業和金融分析,ML成功的驅動力是神經網絡(NN)算法的出現,例如深度神經網絡(DNN),深度學習(DL)和峰值神經網絡(SNN)。通過支持當今不斷發展的計算機格局,ML加速器可以更好地利用數據和線程級別的並發性,從而通過使用具有有限計算和內存資源的嵌入式系統來實現更強大的ML任務解決能力。本書全面概述了嵌入式ML方法的現狀及其在各個領域的應用,涵蓋了將ML應用於資源有限的設備的理論基礎和實際挑戰。第一章:機器學習的演變第一章首先研究學習和理解技術演變過程的必要性。
