
BOOKS - Fundamental Mathematical Concepts for Machine Learning in Science

Fundamental Mathematical Concepts for Machine Learning in Science
Author: Umberto Michelucci
Year: 2024
Pages: 259
Format: PDF | EPUB
File size: 10.1 MB
Language: ENG
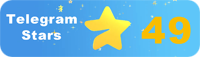
Year: 2024
Pages: 259
Format: PDF | EPUB
File size: 10.1 MB
Language: ENG
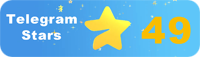
The book "Fundamental Mathematical Concepts for Machine Learning in Science" is a comprehensive guide to understanding the mathematical foundations of machine learning and its applications in various scientific fields. The author, a renowned expert in the field, provides a thorough explanation of the underlying principles and techniques that govern the development of machine learning algorithms and their implementation in real-world scenarios. The book covers a wide range of topics, from linear algebra and calculus to probability theory and statistical inference, all of which are essential for mastering machine learning concepts. The author emphasizes the importance of studying and understanding the process of technological evolution, highlighting the need for a personal paradigm for perceiving the technological process of developing modern knowledge as the basis for the survival of humanity and the survival of the unification of people in a warring state. This perspective is crucial for recognizing the potential of machine learning in addressing complex problems and shaping the future of science and society. The book begins by introducing the fundamental concepts of machine learning, including supervised and unsupervised learning, neural networks, and deep learning. It then delves into the mathematical underpinnings of these concepts, providing readers with a solid foundation for understanding the more advanced topics covered later in the book.
Книга «Фундаментальные математические концепции машинного обучения в науке» представляет собой всеобъемлющее руководство по пониманию математических основ машинного обучения и его приложений в различных научных областях. Автор, известный эксперт в этой области, дает подробное объяснение основополагающих принципов и методов, которые управляют разработкой алгоритмов машинного обучения и их реализацией в реальных сценариях. Книга охватывает широкий круг тем, от линейной алгебры и исчисления до теории вероятностей и статистического вывода, все из которых необходимы для освоения концепций машинного обучения. Автор подчеркивает важность изучения и понимания процесса технологической эволюции, подчеркивая необходимость личностной парадигмы восприятия технологического процесса развития современного знания как основы выживания человечества и выживания объединения людей в воюющем государстве. Эта перспектива имеет решающее значение для признания потенциала машинного обучения в решении сложных проблем и формировании будущего науки и общества. Книга начинается с введения фундаментальных концепций машинного обучения, включая обучение с учителем и без учителя, нейронные сети и глубокое обучение. Затем он углубляется в математические основы этих концепций, предоставляя читателям прочную основу для понимания более продвинутых тем, затронутых позже в книге.
''
