
BOOKS - MLOps with Ray Best Practices and Strategies for Adopting Machine Learning Op...

MLOps with Ray Best Practices and Strategies for Adopting Machine Learning Operations
Author: Hien Luu, Max Pumperla, Zhe Zhang
Year: 2024
Pages: 342
Format: PDF | EPUB
File size: 11.8 MB
Language: ENG
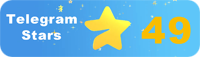
Year: 2024
Pages: 342
Format: PDF | EPUB
File size: 11.8 MB
Language: ENG
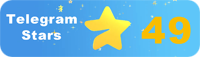
The book "MLOps with Ray Best Practices and Strategies for Adopting Machine Learning Operations" provides a comprehensive guide to implementing machine learning operations (MLOps) in organizations, focusing on the use of Ray, an open-source framework for building, deploying, and managing machine learning models. The book covers the entire lifecycle of MLOps, from data preparation and model training to deployment and maintenance, and offers practical strategies for overcoming common challenges and obstacles. The book begins by highlighting the importance of understanding the technology evolution process and its impact on society. As technology continues to advance at an unprecedented pace, it is essential to develop a personal paradigm for perceiving the technological process of developing modern knowledge. This involves recognizing the interconnectedness of technology and its role in shaping our worldview, values, and beliefs. By doing so, we can better understand the implications of technology on humanity and make informed decisions about its adoption and application. The book then delves into the concept of MLOps and its significance in the field of machine learning. MLOps refers to the practice of integrating machine learning into DevOps processes, enabling organizations to streamline their workflows and improve the efficiency of their machine learning projects. With the increasing demand for AI and machine learning applications, MLOps has become a crucial aspect of software development, particularly in industries such as healthcare, finance, and marketing. The authors emphasize the need for a holistic approach to MLOps, considering factors such as data management, model training, deployment, and monitoring. They provide practical strategies for each stage of the MLOps lifecycle, including data preparation, model selection, training and validation, deployment, and maintenance.
В книге «MLOps with Ray Best Practices and Strategies for Adopting Machine arning Operations» представлено всеобъемлющее руководство по внедрению операций машинного обучения (MLOps) в организациях с акцентом на использование Ray, инфраструктуры с открытым исходным кодом для построения, развертывания и управления моделями машинного обучения. Книга охватывает весь жизненный цикл MLOps, от подготовки данных и обучения модели до развертывания и обслуживания, и предлагает практические стратегии для преодоления общих проблем и препятствий. Книга начинается с того, что подчеркивается важность понимания процесса эволюции технологий и его влияния на общество. Поскольку технологии продолжают развиваться беспрецедентными темпами, важно разработать личную парадигму восприятия технологического процесса развития современных знаний. Это предполагает признание взаимосвязанности технологий и их роли в формировании нашего мировоззрения, ценностей и убеждений. Тем самым мы сможем лучше понять последствия технологии для человечества и принять обоснованные решения о ее принятии и применении. Затем книга углубляется в понятие MLOps и его значение в области машинного обучения. MLOps относится к практике интеграции машинного обучения в процессы DevOps, что позволяет организациям оптимизировать свои рабочие процессы и повысить эффективность своих проектов в области машинного обучения. С ростом спроса на приложения для искусственного интеллекта и машинного обучения MLOps стал важнейшим аспектом разработки программного обеспечения, особенно в таких отраслях, как здравоохранение, финансы и маркетинг. Авторы подчеркивают необходимость целостного подхода к MLOps с учетом таких факторов, как управление данными, обучение моделей, развертывание и мониторинг. Они предоставляют практические стратегии для каждого этапа жизненного цикла MLOps, включая подготовку данных, выбор модели, обучение и проверку, развертывание и обслуживание.
''
