
BOOKS - Just Enough Data Science and Machine Learning Essential Tools and Techniques

Just Enough Data Science and Machine Learning Essential Tools and Techniques
Author: Mark Levene, Martyn Harris
Year: 2025
Pages: 224
Format: EPUB
File size: 10.1 MB
Language: ENG
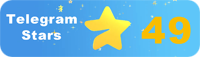
Year: 2025
Pages: 224
Format: EPUB
File size: 10.1 MB
Language: ENG
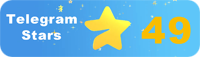
Book Description: The book "Just Enough Data Science and Machine Learning Essential Tools and Techniques" provides readers with a comprehensive overview of data science and machine learning techniques that can be applied in real-world scenarios. The book covers topics such as data preprocessing, feature selection, supervised and unsupervised learning algorithms, and model evaluation. It also discusses the importance of understanding the underlying principles of machine learning and data science to effectively apply these techniques in various industries. The author emphasizes the need to develop a personal paradigm for understanding the technological process of developing modern knowledge, which is essential for the survival of humanity and the unity of people in a warring state. This paradigm involves recognizing the interconnectedness of technology and society and the need to adapt to changing circumstances. The book begins by introducing the concept of data science and its importance in today's world. It highlights the role of data science in shaping our understanding of the world and how it has revolutionized various industries. The author then delves into the basics of data preprocessing, explaining the different types of data and their sources, as well as the methods used to clean and prepare them for analysis. The next chapter focuses on feature selection, which is critical in ensuring that the right features are selected for analysis. The author provides examples of different feature selection techniques and their applications in various industries. The chapter also covers the importance of feature engineering and how it can improve the accuracy of machine learning models. The book then moves on to supervised and unsupervised learning algorithms, providing readers with an in-depth understanding of these techniques and their applications. The author explains the differences between these two types of algorithms and their uses in different scenarios.
Книга «Достаточно основных инструментов и методов Data Science и Machine arning» предоставляет читателям всесторонний обзор методов Data Science и Machine arning, которые могут быть применены в реальных сценариях. Книга охватывает такие темы, как предварительная обработка данных, выбор признаков, алгоритмы обучения под наблюдением и без присмотра, а также оценка модели. В нем также обсуждается важность понимания основополагающих принципов машинного обучения и науки о данных для эффективного применения этих методов в различных отраслях. Автор подчеркивает необходимость выработки личностной парадигмы понимания технологического процесса развития современного знания, необходимого для выживания человечества и единства людей в воюющем государстве. Эта парадигма предполагает признание взаимосвязанности технологий и общества и необходимость адаптации к меняющимся обстоятельствам. Книга начинается с введения концепции науки о данных и её важности в современном мире. В нем подчеркивается роль науки о данных в формировании нашего понимания мира и того, как она произвела революцию в различных отраслях. Затем автор углубляется в основы предварительной обработки данных, объясняя различные типы данных и их источники, а также методы, используемые для их очистки и подготовки к анализу. Следующая глава посвящена выбору элементов, что очень важно для обеспечения правильного выбора элементов для анализа. Автор приводит примеры различных техник выбора признаков и их применения в различных отраслях. Глава также освещает важность feature engineering и то, как она может повысить точность моделей машинного обучения. Затем книга переходит к контролируемым и неконтролируемым алгоритмам обучения, предоставляя читателям глубокое понимание этих методов и их приложений. Автор объясняет различия между этими двумя типами алгоритмов и их использование в разных сценариях.
''
