
BOOKS - Graph Neural Networks in Action

Graph Neural Networks in Action
Author: Keita Broadwater
Year: June 25, 2024
Format: PDF
File size: PDF 30 MB
Language: English
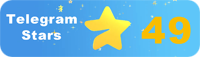
Year: June 25, 2024
Format: PDF
File size: PDF 30 MB
Language: English
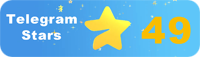
The following is a list of some of the most common graph neural networks (GNNs) architectures, along with their strengths and weaknesses: 1. Graph Convolutional Networks (GCNs): GCNs are a type of neural network designed for graph-structured data. They use a convolutional layer to aggregate node features based on the graph structure. Strengths: effective for dense graphs, can capture long-range dependencies. Weaknesses: less effective for sparse graphs, can be computationally expensive. 2. Graph Attention Networks (GATs): GATs are a type of GNN that use attention mechanisms to learn the importance of different nodes in the graph. Strengths: can handle large-scale graphs, better performance on sparse graphs. Weaknesses: require more parameters and computations than GCNs. 3. Graph Isomorphism Networks (GINs): GINs are a type of GNN that learn a representation of the graph structure. Strengths: can handle large-scale graphs, invariant to graph isomorphisms. Weaknesses: less effective for tasks that require node features. 4.
Ниже приведен список некоторых наиболее распространенных архитектур нейронных сетей графов (GNN), а также их сильные и слабые стороны: 1. Графовые сверточные сети (англ. Graph Convolutional Networks, GCN): GCN - тип нейронных сетей, предназначенных для графоструктурированных данных. Они используют сверточный слой для агрегирования узловых элементов на основе структуры графа. Сильные стороны: эффективен для плотных графов, может улавливать дальнодействующие зависимости. Слабые стороны: менее эффективен для разреженных графов, может быть вычислительно затратен. 2. Graph Attention Networks (GAT): GAT - это тип GNN, который использует механизмы внимания, чтобы узнать важность различных узлов в графе. Сильные стороны: может обрабатывать крупномасштабные графы, лучшая производительность на разреженных графах. Слабые стороны: требуется больше параметров и вычислений, чем GCN. 3. Сети изоморфизма графов (GIN): GIN - это тип GNN, которые изучают представление структуры графа. Сильные стороны: может обрабатывать крупномасштабные графы, инвариантные к изоморфизмам графов. Слабые стороны: менее эффективен для задач, требующих особенностей узла. 4.
Voici une liste de certaines des architectures les plus courantes des réseaux neuronaux de graphes (GNN), ainsi que leurs forces et faiblesses : 1. Réseaux convolutifs graphiques (GCN) : GCN est un type de réseaux neuronaux conçus pour les données graphos..... Ils utilisent une couche convolutive pour agréger les éléments nodaux à partir de la structure du graphe. Points forts : efficace pour les graphes denses, peut capter les dépendances à longue distance. Faiblesses : moins efficace pour les graphes dilués, peut être un coût de calcul. 2. GAT (GAT) : GAT est un type de GNN qui utilise des mécanismes d'attention pour apprendre l'importance des différents nœuds dans le graphique. Points forts : peut traiter les graphes à grande échelle, la meilleure performance sur les graphes dilués. Faiblesses : plus de paramètres et de calculs sont nécessaires que le GCN. 3. Réseaux d'isomorphisme de graphe (GIN) : GIN est un type de GNN qui étudie la représentation de la structure du graphe. Points forts : peut traiter les graphes à grande échelle invariants aux isomorphismes des graphes. Faiblesses : moins efficace pour les tâches nécessitant des caractéristiques nodales. 4.
A continuación se muestra una lista de algunas de las arquitecturas más comunes de las redes neuronales de grafos (GNN), así como sus fortalezas y debilidades: 1. Graph Convolutional Networks (GCN): GCN es un tipo de red neuronal diseñada para datos de estructura gráfica. Utilizan una capa de taladro para agregar nodos basados en la estructura del gráfico. Fortalezas: eficaz para grafos densos, puede capturar dependencias de largo alcance. Debilidades: menos eficientes para grafos dispersos, puede ser computacionalmente costoso. 2. Graph Atention Networks (GAT): GAT es un tipo de GNN que utiliza mecanismos de atención para aprender la importancia de los diferentes nodos en un grafo. Fortalezas: puede manejar grafos a gran escala, el mejor rendimiento en gráficos cortados. Debilidades: se requieren más parámetros y cálculos que GCN. 3. Redes de isomorfismo de grafos (GIN): GIN es un tipo de GNN que estudia la representación de la estructura de un gráfico. Fortalezas: puede tratar grafos a gran escala invariantes a isomorfismos de grafos. Debilidades: menos eficaces para tareas que requieren características del nodo. 4.
Veja abaixo algumas das arquiteturas mais comuns de redes neurais de gráficos (GNN) e seus pontos fortes e fracos: 1. Graph Conversional Networks (GCN): GCN é um tipo de rede neural projetada para dados gráficos. Eles usam uma camada comprimida para agregação de nódulos com base na estrutura do gráfico. Pontos fortes: eficaz para gráficos densos, capaz de capturar dependências de longo alcance. Fraquezas: menos eficaz para gráficos dilacerados, pode ser calculado. 2. Graph Action Networks (GAT): O GAT é um tipo de GNN que usa mecanismos de atenção para saber a importância de vários nós na grafia. Pontos fortes: pode processar gráficos em larga escala, melhor desempenho em gráficos dilacerados. Pontos fracos: necessita de mais parâmetros e cálculos do que GCN. 3. Redes de isomorfismo de grafos (GIN): GIN é um tipo de GNN que estuda a representação da estrutura do grafo. Pontos fortes: pode processar grafos em larga escala invariantes a isomorfismos de grafos. Fraquezas: menos eficaz para tarefas que requerem características de nó. 4.
Di seguito sono riportate alcune delle architetture più comuni delle reti di grafica neurale (GNN) e i loro punti di forza e debolezza: 1. Reti grafiche compresse (Graph Convertional Networks GCN): GCN è un tipo di rete neurale progettata per i dati grafoststrutturati. Utilizzano un livello compresso per aggregare i nodi in base alla struttura del grafico. Punti di forza: è efficace per grafici densi, può catturare dipendenze a lungo raggio. Punti deboli: meno efficace per i grafici dilatati, più costoso per i calcoli. 2. Graph Attraction Networks (GAT): GAT è un tipo di GNN che utilizza meccanismi di attenzione per conoscere l'importanza dei vari nodi nella casella. Punti di forza: è in grado di elaborare grafici su larga scala, migliori prestazioni su grafici ridotti. Punti deboli: sono necessari più parametri e calcoli di GCN. 3. Reti isomorfismo grafico (GIN): GIN è un tipo di GNN che esamina la visualizzazione della struttura del grafico. Punti di forza: può elaborare grafici su larga scala invarianti a isomorfismi grafici. Punti deboli: meno efficace per le attività che richiedono le caratteristiche del sito. 4.
Nachfolgend finden e eine Liste einiger der gängigsten Architekturen neuronaler Graphennetze (GNN) sowie deren Stärken und Schwächen: 1. Graph Convolutional Networks (GCN): Ein GCN ist eine Art neuronales Netzwerk, das für graphostrukturierte Daten entwickelt wurde. e verwenden eine Faltungsschicht, um Knotenelemente basierend auf der Graphenstruktur zu aggregieren. Stärken: wirksam für dichte Graphen, kann langwirksame Abhängigkeiten erfassen. Schwächen: weniger effizient für spärliche Graphen, kann rechnerisch teuer sein. 2. Graph Attention Networks (GAT): GAT ist eine Art von GNN, die Aufmerksamkeitsmechanismen verwendet, um die Bedeutung der verschiedenen Knoten in einem Graph zu lernen. Stärken: Kann große Graphen verarbeiten, bessere istung auf spärlichen Graphen. Schwächen: erfordert mehr Parameter und Berechnungen als GCN. 3. Graph Isomorphism Networks (GIN): GIN ist ein GNN-Typ, der die Darstellung der Graphenstruktur untersucht. Stärken: kann großformatige Graphen verarbeiten, die gegenüber Graphisomorphismen invariant sind. Schwächen: weniger effektiv für Aufgaben, die Knotenmerkmale erfordern. 4.
Poniżej znajduje się lista niektórych najczęstszych architektur sieci neuronowych wykresów (GNN), a także ich mocnych i słabych stron: 1. Graph Convolutional Networks (GCN): GCN jest rodzajem sieci neuronowej dla danych ustrukturyzowanych na wykresie. Stosują warstwę konwolucyjną do agregowania elementów węzłowych w oparciu o strukturę wykresu. Mocne strony: Skuteczne dla gęstych wykresów, może przechwytywać zależności dalekiego zasięgu. Słabości: mniej wydajne dla słabych wykresów, mogą być kosztowne obliczeniowo. 2. Graph Attention Networks (GAT): GAT jest rodzajem GNN, który wykorzystuje mechanizmy uwagi do poznawania znaczenia różnych węzłów na wykresie. Mocne strony: może obsługiwać wykresy na dużą skalę, lepszą wydajność na słabych wykresach. Niedociągnięcia: Potrzebne są więcej parametrów i obliczeń niż GCN. 3. eci izomorfizmu grafu (GIN): GIN jest rodzajem GNN, który bada reprezentację struktury wykresu. Mocne strony: potrafi obsługiwać wykresy na dużą skalę niezmienne do izomorfizmów wykresu. Słabości: Mniej skuteczne w przypadku zadań wymagających funkcji węzła. 4.
להלן היא רשימה של כמה מהרשת העצבית הגרפית הנפוצה ביותר (GNN) ארכיטקטורה, כמו גם החוזקות והחולשות שלהם: 1. Graph Convolutional Networks (GCN): GCN הוא סוג של רשת עצבית המיועדת לנתונים מובנים בגרף. הם משתמשים בשכבה קונבולוציונית כדי לצבור אלמנטים נודליים המבוססים על מבנה גרף. חזקות: יעיל עבור גרפים צפופים, יכול ללכוד תלויות ארוכות טווח. חולשות: פחות יעילות עבור גרפים דלים, יכולות להיות יקרות מבחינה חישובית. 2. GATT הוא סוג של GNN המשתמש במנגנוני קשב כדי ללמוד את חשיבותם של צמתים שונים בגרף. חוזק: יכול להתמודד עם גרפים בקנה מידה גדול, ביצועים טובים יותר על גרפים דלילים. חולשות: יש צורך ביותר פרמטרים וחישובים מאשר GCN. 3. GIN הוא סוג של GNN החוקר את ייצוג מבנה הגרף. חוזק: יכול להתמודד עם גרפים בקנה מידה גדול אינווריאנטים לגרף איזומורפיזמים. חולשות: פחות יעילות למשימות הדורשות מאפייני צומת. 4.''
Aşağıda, en yaygın grafik sinir ağı (GNN) mimarilerinden bazılarının yanı sıra güçlü ve zayıf yönlerinin bir listesi bulunmaktadır: 1. Graph Convolutional Networks (GCN): GCN, grafik yapılandırılmış veriler için bir tür sinir ağıdır. Grafik yapısına dayalı düğüm elemanlarını toplamak için evrişimli bir katman kullanırlar. Güçlü Yönleri: Yoğun grafikler için etkilidir, uzun menzilli bağımlılıkları yakalayabilir. Zayıf yönleri: Seyrek grafikler için daha az verimli, hesaplama açısından maliyetli olabilir. 2. Grafik Dikkat Ağları (GAT): Bir GAT, bir grafikteki farklı düğümlerin önemini öğrenmek için dikkat mekanizmalarını kullanan bir GNN türüdür. Güçlü Yönler: Büyük ölçekli grafikleri, seyrek grafiklerde daha iyi performansı işleyebilir. Zayıf Yönleri: GCN'den daha fazla parametre ve hesaplama gereklidir. 3. Grafik izomorfizm ağları (GIN): GIN, grafik yapısının temsilini inceleyen bir GNN türüdür. Strengths: izomorfizmleri çizmek için değişmeyen büyük ölçekli grafikleri işleyebilir. Zayıf yönleri: Düğüm özellikleri gerektiren görevler için daha az etkilidir. 4.
فيما يلي قائمة ببعض بنى الشبكة العصبية للرسم البياني الأكثر شيوعًا (GNN)، بالإضافة إلى نقاط قوتها وضعفها: 1. الرسم البياني الشبكات التلافيفية (GCN): GCN هو نوع من الشبكات العصبية للبيانات المنظمة للرسم البياني. يستخدمون طبقة تلافيفية لتجميع العناصر العقدية بناءً على بنية الرسم البياني. نقاط القوة: فعالة للرسوم البيانية الكثيفة، يمكنها التقاط التبعيات بعيدة المدى. نقاط الضعف: أقل كفاءة للرسوم البيانية المتناثرة، يمكن أن تكون مكلفة من الناحية الحسابية. 2. شبكات الانتباه البياني (GAT): GAT هو نوع من GNN يستخدم آليات الانتباه لمعرفة أهمية العقد المختلفة في الرسم البياني. نقاط القوة: يمكنها التعامل مع الرسوم البيانية واسعة النطاق، وأداء أفضل على الرسوم البيانية المتناثرة. نقاط الضعف: هناك حاجة إلى معايير وحسابات أكثر من GCN. 3. GIN هو نوع من GNN يدرس تمثيل بنية الرسم البياني. نقاط القوة: يمكنها التعامل مع الرسوم البيانية واسعة النطاق الثابتة لرسم تماثل الأشكال. نقاط الضعف: أقل فعالية للمهام التي تتطلب ميزات العقدة. 4.
아래는 가장 일반적인 그래프 신경망 (GNN) 아키텍처 목록과 강점 및 약점 목록입니다. GCN (Graph Convolutional Networks): GCN은 그래프 구조 데이터를위한 일종의 신경망입니다. 그들은 컨볼 루션 레이어를 사용하여 그래프 구조를 기반으로 노드 요소를 집계합니다. 강점: 밀도가 높은 그래프에 효과적으로 장거리 종속성을 포착 할 수 있습니다. 약점: 드문 드문 그래프의 효율성이 떨어지면 계산 비용이 많이들 수 있습니다. 2. GAT (Graph Attention Networks): GAT는주의 메커니즘을 사용하여 그래프에서 다른 노드의 중요성을 배우는 GNN 유형입니다. 강점: 대규모 그래프를 처리하고 희소 그래프의 성능을 향상시킬 수 있습니다. 약점: GCN보다 더 많은 매개 변수 및 계산이 필요합니다. 3. 그래프 동형 네트워크 (GIN): GIN은 그래프 구조의 표현을 연구하는 GNN 유형입니다. 강점: 그래프 동형에 변하지 않는 대규모 그래프를 처리 할 수 있습니다. 약점: 노드 기능이 필요한 작업에는 덜 효과적입니다. 4.
以下は、最も一般的なグラフニューラルネットワーク(GNN)アーキテクチャの一覧です。Graph Convolutional Networks (GCN): GCNはグラフ構造化データのニューラルネットワークの一種である。これらは畳み込み層を使用して、グラフ構造に基づいてノード要素を集約します。強み:高密度グラフに効果的で、長距離依存をキャプチャできます。弱点:まばらなグラフの効率が低く、計算コストがかかる可能性があります。2.グラフ注意ネットワーク(GAT): GATは、注意メカニズムを使用してグラフ内のさまざまなノードの重要性を学習するGNNの一種です。強み:大規模なグラフ、まばらなグラフでのパフォーマンスを向上させることができます。弱点:GCNよりも多くのパラメータと計算が必要です。3.グラフ同型ネットワーク(GIN): GINはグラフ構造の表現を研究するGNNの一種である。強み:グラフ同型に不変な大規模グラフを扱うことができます。弱点:ノード機能を必要とするタスクに対して効果が低くなります。4.
以下是一些最常見的圖神經網絡(GNN)架構及其優缺點列表:1。圖形卷積網絡(GCN):GCN是為圖形結構化數據設計的一種神經網絡。他們使用卷積層根據圖的結構聚合節點元素。強度:對密集圖有效,可以捕捉到遠程作用的依賴性。弱點:對稀疏圖效率較低,計算成本較高.2.圖形註意網絡(GAT):GAT是一種使用GNN的註意力機制來了解圖中不同節點的重要性的GNN。優點:可以處理大型圖,在稀疏圖上性能更好。弱點:需要比GCN更多的參數和計算。3.圖同構網絡(GIN):GIN是研究圖結構表示的GNN類型。強度:可以處理與圖同構不變的大規模圖。弱點:對於需要節點功能的任務,效果較差。4.
