
BOOKS - PROGRAMMING - Privacy-Preserving Machine Learning

Privacy-Preserving Machine Learning
Year: 2022 MEAP V8
Pages: 323
Format: PDF
File size: 13,2 MB
Language: ENG
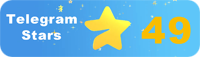
Pages: 323
Format: PDF
File size: 13,2 MB
Language: ENG
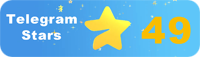
PrivacyPreserving Machine Learning: A Practical Guide to Keeping ML Data Anonymous and Secure = The world we live in today is vastly different from the one our parents or grandparents grew up in. With the rapid advancement of technology, especially in the field of machine learning (ML), it's becoming increasingly difficult to maintain our privacy. Our personal information is being collected, stored, and analyzed at an unprecedented rate, leaving us vulnerable to identity theft, cyber attacks, and other forms of exploitation. As a result, there is a growing need for privacy-preserving technologies that can protect our sensitive data while still allowing us to benefit from the advantages of ML. In this book, we will delve into the complex world of privacy-enhancing technologies and explore real-world use cases for facial recognition, cloud data storage, and more. We will demystify these technologies and provide practical skills for technical implementation, ensuring that your ML systems are both effective and secure. You'll learn about current and future machine learning privacy challenges and how to adapt technologies to your specific needs. By the end of this guide, you'll be able to create ML systems that preserve user privacy without sacrificing data quality and model performance. Understanding the Evolution of Technology Before diving into the nitty-gritty of privacy-preserving technologies, it's essential to understand the evolution of technology and its impact on society.
КонфиденциальностьСохранение машинного обучения: Практическое руководство по сохранению анонимности и безопасности данных ML = Мир, в котором мы живем сегодня, значительно отличается от того, в котором выросли наши родители или бабушки и дедушки. С быстрым развитием технологий, особенно в области машинного обучения (ML), становится все труднее сохранять конфиденциальность. Наша личная информация собирается, хранится и анализируется с беспрецедентной скоростью, что делает нас уязвимыми для кражи личных данных, кибератак и других форм эксплуатации. В результате растет потребность в технологиях, сохраняющих конфиденциальность, которые могут защитить наши конфиденциальные данные, сохраняя при этом преимущества ML. В этой книге мы познакомимся со сложным миром технологий, повышающих конфиденциальность, и рассмотрим реальные сценарии использования распознавания лиц, облачного хранения данных и многого другого. Мы проведем демистификацию этих технологий и предоставим практические навыки для технического внедрения, гарантируя, что ваши системы ML будут эффективными и безопасными. Вы узнаете о текущих и будущих проблемах конфиденциальности машинного обучения и о том, как адаптировать технологии к вашим конкретным потребностям. К концу этого руководства вы сможете создавать ML-системы, сохраняющие конфиденциальность пользователей без ущерба для качества данных и производительности модели. Понимание эволюции технологий Прежде чем погружаться в мелочь технологий, сохраняющих конфиденциальность, важно понять эволюцию технологий и их влияние на общество.
ConfidentialitéPréserver l'apprentissage automatique : guide pratique pour préserver l'anonymat et la sécurité des données ML = monde dans lequel nous vivons aujourd'hui est très différent de celui dans lequel nos parents ou grands-parents ont grandi. Avec le développement rapide de la technologie, en particulier dans le domaine de l'apprentissage automatique (ML), il devient de plus en plus difficile de préserver la confidentialité. Nos renseignements personnels sont recueillis, stockés et analysés à une vitesse sans précédent, ce qui nous rend vulnérables au vol d'identité, aux cyberattaques et à d'autres formes d'exploitation. Il en résulte un besoin croissant de technologies de protection de la vie privée qui peuvent protéger nos données sensibles tout en préservant les avantages de ML. Dans ce livre, nous nous familiariserons avec le monde complexe des technologies qui augmentent la confidentialité et examinerons des scénarios réels d'utilisation de la reconnaissance faciale, du stockage cloud et bien plus encore. Nous allons démystifier ces technologies et fournir des compétences pratiques pour la mise en œuvre technique, en veillant à ce que vos systèmes ML soient efficaces et sécurisés. Vous en apprendrez plus sur les défis actuels et futurs de la confidentialité de l'apprentissage automatique et sur la façon d'adapter la technologie à vos besoins spécifiques. À la fin de ce guide, vous pourrez créer des systèmes ML qui gardent la vie privée des utilisateurs sans compromettre la qualité des données et les performances du modèle. Comprendre l'évolution des technologies Avant de s'immerger dans les petites technologies qui préservent la confidentialité, il est important de comprendre l'évolution des technologies et leur impact sur la société.
PrivacidadPreservación del aprendizaje automático: Guía práctica para preservar el anonimato y la seguridad de los datos ML = mundo en el que vivimos hoy es significativamente diferente del en el que crecieron nuestros padres o abuelos. Con el rápido desarrollo de la tecnología, especialmente en el campo del aprendizaje automático (ML), es cada vez más difícil mantener la privacidad. Nuestra información personal se recopila, almacena y analiza a una velocidad sin precedentes, lo que nos hace vulnerables al robo de identidad, ciberataques y otras formas de explotación. Como resultado, hay una creciente necesidad de tecnologías que mantengan la privacidad, que puedan proteger nuestros datos confidenciales, al tiempo que preservan los beneficios de ML. En este libro, conoceremos el complejo mundo de las tecnologías que mejoran la privacidad y analizaremos escenarios reales sobre el uso del reconocimiento facial, el almacenamiento en la nube y más. Desmitificaremos estas tecnologías y proporcionaremos habilidades prácticas para la implementación técnica, asegurando que sus sistemas ML sean eficientes y seguros. Aprenderá sobre los desafíos actuales y futuros de la privacidad del aprendizaje automático y cómo adaptar la tecnología a sus necesidades específicas. Al final de esta guía, podrá crear sistemas ML que mantengan la privacidad de los usuarios sin comprometer la calidad de los datos y el rendimiento del modelo. Comprender la evolución de la tecnología Antes de sumergirse en una pequeña cosa de la tecnología que mantiene la privacidad, es importante comprender la evolución de la tecnología y su impacto en la sociedad.
A preservação do aprendizado de máquina: Guia prático para preservar o anonimato e a segurança dos dados ML = O mundo em que vivemos hoje é muito diferente do que os nossos pais ou avós cresceram. Com o rápido desenvolvimento da tecnologia, especialmente na área de aprendizagem de máquinas (ML), torna-se cada vez mais difícil manter a privacidade. Nossas informações pessoais são coletadas, armazenadas e analisadas a uma velocidade sem precedentes, o que nos torna vulneráveis ao roubo de identidade, ciberataques e outras formas de exploração. Como resultado, há uma necessidade crescente de tecnologias de privacidade que podem proteger nossos dados confidenciais, mantendo os benefícios do ML. Neste livro, conheceremos o complexo mundo da tecnologia que aumenta a privacidade e examinaremos cenários reais de reconhecimento facial, armazenamento de dados em nuvem e muito mais. Vamos desmistificar essas tecnologias e fornecer habilidades práticas para a implementação técnica, garantindo que seus sistemas ML sejam eficientes e seguros. Você vai aprender sobre os desafios atuais e futuros da privacidade do aprendizado de máquinas e como adaptar a tecnologia às suas necessidades específicas. Ao final deste manual, você poderá criar sistemas ML que preservem a privacidade dos usuários sem comprometer a qualidade dos dados e o desempenho do modelo. Compreender a evolução da tecnologia Antes de mergulhar em pequenas tecnologias de privacidade, é importante compreender a evolução da tecnologia e seus efeitos na sociedade.
Conservazione dell'apprendimento automatico: Manuale pratico per mantenere l'anonimato e la sicurezza dei dati ML = Il mondo in cui viviamo oggi è molto diverso da quello in cui i nostri genitori o nonni sono cresciuti. Con il rapido sviluppo della tecnologia, soprattutto nel campo dell'apprendimento automatico (ML), diventa sempre più difficile mantenere la privacy. nostre informazioni personali vengono raccolte, memorizzate e analizzate a velocità senza precedenti, rendendoci vulnerabili a furti di identità, attacchi informatici e altre forme di sfruttamento. Di conseguenza, le tecnologie che mantengono la privacy sono sempre più necessarie per proteggere i nostri dati sensibili, mantenendo al contempo i vantaggi di ML. In questo libro scopriremo il complesso mondo della tecnologia che migliora la privacy e esamineremo gli scenari reali per il riconoscimento facciale, lo storage cloud e altro ancora. Demistetizzeremo queste tecnologie e forniremo competenze pratiche per l'implementazione tecnica, assicurandoci che i vostri sistemi ML siano efficienti e sicuri. Scoprirete i problemi attuali e futuri relativi alla privacy dell'apprendimento automatico e come adattare la tecnologia alle vostre esigenze specifiche. Al termine di questa guida sarà possibile creare sistemi ML che mantengano la privacy degli utenti senza compromettere la qualità dei dati e le prestazioni del modello. Capire l'evoluzione della tecnologia Prima di immergersi in piccole tecnologie che mantengono la privacy, è importante comprendere l'evoluzione della tecnologia e il loro impatto sulla società.
DatenschutzMaschinelles rnen bewahren: Ein praktischer itfaden zur Wahrung der Anonymität und Datensicherheit ML = Die Welt, in der wir heute leben, unterscheidet sich erheblich von der Welt, in der unsere Eltern oder Großeltern aufgewachsen sind. Mit der rasanten Entwicklung der Technologie, insbesondere im Bereich des maschinellen rnens (ML), wird es immer schwieriger, die Privatsphäre zu wahren. Unsere persönlichen Daten werden mit beispielloser Geschwindigkeit gesammelt, gespeichert und analysiert, was uns anfällig für Identitätsdiebstahl, Cyberangriffe und andere Formen der Ausbeutung macht. Infolgedessen besteht ein wachsender Bedarf an Technologien zur Wahrung der Privatsphäre, die unsere sensiblen Daten schützen und gleichzeitig die Vorteile von ML erhalten können. In diesem Buch werden wir uns mit der komplexen Welt der datenschutzfördernden Technologien vertraut machen und reale Anwendungsfälle für Gesichtserkennung, Cloud-Speicher und mehr untersuchen. Wir werden diese Technologien entmystifizieren und praktische Fähigkeiten für die technische Implementierung bereitstellen, um sicherzustellen, dass Ihre ML-Systeme effizient und sicher sind. e lernen aktuelle und zukünftige Datenschutzherausforderungen des maschinellen rnens kennen und erfahren, wie e Technologien an Ihre spezifischen Bedürfnisse anpassen können. Am Ende dieses Handbuchs können e ML-Systeme erstellen, die die Privatsphäre der Benutzer wahren, ohne die Datenqualität und die istung des Modells zu beeinträchtigen. Verstehen e die Entwicklung der Technologie Bevor e in die Kleinigkeiten der Technologie eintauchen, die die Privatsphäre bewahrt, ist es wichtig, die Entwicklung der Technologie und ihre Auswirkungen auf die Gesellschaft zu verstehen.
PrywatneUczenie maszynowe: Praktyczny przewodnik po zachowaniu anonimowości i bezpieczeństwa danych ML = Świat, w którym żyjemy dzisiaj, różni się znacznie od tego, w którym dorastali nasi rodzice lub dziadkowie. Wraz z szybkim rozwojem technologii, zwłaszcza w dziedzinie uczenia maszynowego (ML), coraz trudniej jest zachować poufność. Nasze dane osobowe są gromadzone, przechowywane i analizowane w bezprecedensowym tempie, dzięki czemu jesteśmy podatni na kradzież tożsamości, cyberataki i inne formy eksploatacji. W rezultacie rośnie zapotrzebowanie na technologie chroniące prywatność, które mogą chronić nasze wrażliwe dane przy jednoczesnym zachowaniu korzyści ML. W tej książce przyjrzymy się złożonemu światu technologii zwiększających prywatność i spojrzymy na przypadki użycia w świecie rzeczywistym do rozpoznawania twarzy, przechowywania w chmurze i nie tylko. Będziemy demystalizować te technologie i zapewniać praktyczne umiejętności w zakresie wdrażania technicznego, zapewniając wydajność i bezpieczeństwo Twoich systemów ML. Dowiesz się o aktualnych i przyszłych zagadnieniach związanych z prywatnością oraz jak dostosować technologię do Twoich specyficznych potrzeb. Pod koniec tego samouczka możesz stworzyć systemy ML, które zachowują prywatność użytkownika bez naruszania jakości danych lub wydajności modelu. Zrozumienie ewolucji technologii Przed nurkowaniem w minutiae technologii ochrony prywatności, ważne jest, aby zrozumieć ewolucję technologii i jej wpływ na społeczeństwo.
Looking Preserving Machine arning: A Practical Guide to Preserving Anonymity and Data Security ML) העולם בו אנו חיים כיום שונה באופן משמעותי מזה שבו גדלו הורינו או סבנו. עם ההתפתחות המהירה של הטכנולוגיה, במיוחד בתחום למידת המכונה (ML), קשה יותר ויותר לשמור על סודיות. המידע האישי שלנו נאסף, מאוחסן ומנותח בקצב חסר תקדים, משאיר אותנו פגיעים לגניבת זהות, תקיפות סייבר וצורות אחרות של ניצול. כתוצאה מכך, יש צורך הולך וגדל בטכנולוגיות לשימור פרטיות שיכולות להגן על המידע הרגיש שלנו תוך שימור היתרונות של ML. בספר הזה, אנחנו מסתכלים על העולם המורכב של טכנולוגיות משפרות פרטיות ומסתכלים על שימוש בעולם האמיתי במקרים לזיהוי פנים, אחסון ענן ועוד. אנחנו נהרוס את הטכנולוגיות האלה ונספק כישורים מעשיים ליישום טכני, ונבטיח שמערכות ה-ML שלכם יעילות ובטוחות. תלמדו על עכשווית ועתידית, על בעיות פרטיות, ואיך להתאים את הטכנולוגיה לצרכים הספציפיים שלכם. עד סוף הדרכה זו, ניתן ליצור מערכות ML אשר משמרות את פרטיות המשתמש מבלי לסכן את איכות המידע או את ביצועי המודל. הבנת האבולוציה של הטכנולוגיה לפני הצלילה למינוטיה של טכנולוגיה לשמירת פרטיות, חשוב להבין את התפתחות הטכנולוגיה ואת השפעתה על החברה.''
PrivacyPreserving Machine arning: A Practical Guide to Preservation Anonimlik ve Veri Güvenliği ML = Bugün yaşadığımız dünya, ebeveynlerimizin veya büyükanne ve büyükbabalarımızın büyüdüğü dünyadan önemli ölçüde farklıdır. Teknolojinin hızla gelişmesiyle, özellikle makine öğrenimi (ML) alanında, gizliliği korumak giderek zorlaşmaktadır. Kişisel bilgilerimiz benzeri görülmemiş bir oranda toplanır, saklanır ve analiz edilir, bu da bizi kimlik hırsızlığına, siber saldırılara ve diğer sömürü biçimlerine karşı savunmasız bırakır. Sonuç olarak, ML'nin faydalarını korurken hassas verilerimizi koruyabilen gizlilik koruma teknolojilerine artan bir ihtiyaç vardır. Bu kitapta, gizlilik artırıcı teknolojilerin karmaşık dünyasına bir göz atıyoruz ve yüz tanıma, bulut depolama ve daha fazlası için gerçek dünyadaki kullanım durumlarına bakıyoruz. Bu teknolojileri açıklığa kavuşturacağız ve teknik uygulama için pratik beceriler sağlayarak ML sistemlerinizin verimli ve güvenli olmasını sağlayacağız. Mevcut ve gelecekteki makine öğrenimi gizlilik sorunlarını ve teknolojiyi özel ihtiyaçlarınıza göre nasıl uyarlayacağınızı öğreneceksiniz. Bu eğiticinin sonunda, veri kalitesinden veya model performansından ödün vermeden kullanıcı gizliliğini koruyan ML sistemleri oluşturabilirsiniz. Teknolojinin evrimini anlamak Mahremiyeti koruyan teknolojinin ayrıntılarına dalmadan önce, teknolojinin evrimini ve toplum üzerindeki etkisini anlamak önemlidir.
PrivacyPreserving Machine arning: دليل عملي للحفاظ على إخفاء الهوية وأمن البيانات ML = يختلف العالم الذي نعيش فيه اليوم اختلافًا كبيرًا عن العالم الذي نشأ فيه آباؤنا أو أجدادنا. مع التطور السريع للتكنولوجيا، خاصة في مجال التعلم الآلي (ML)، يصبح من الصعب بشكل متزايد الحفاظ على السرية. يتم جمع معلوماتنا الشخصية وتخزينها وتحليلها بمعدل غير مسبوق، مما يجعلنا عرضة لسرقة الهوية والهجمات الإلكترونية وغيرها من أشكال الاستغلال. نتيجة لذلك، هناك حاجة متزايدة لتقنيات الحفاظ على الخصوصية التي يمكن أن تحمي بياناتنا الحساسة مع الحفاظ على فوائد ML. في هذا الكتاب، نلقي نظرة على العالم المعقد لتقنيات تعزيز الخصوصية ونلقي نظرة على حالات الاستخدام الواقعية للتعرف على الوجه والتخزين السحابي والمزيد. سنزيل الغموض عن هذه التقنيات ونوفر المهارات العملية للتنفيذ التقني، مما يضمن أن أنظمة ML الخاصة بك فعالة وآمنة. ستتعرف على مشكلات خصوصية التعلم الآلي الحالية والمستقبلية وكيفية تكييف التكنولوجيا مع احتياجاتك المحددة. بحلول نهاية هذا البرنامج التعليمي، يمكنك إنشاء أنظمة ML التي تحافظ على خصوصية المستخدم دون المساس بجودة البيانات أو أداء الطراز. فهم تطور التكنولوجيا قبل الغوص في التفاصيل الدقيقة لتكنولوجيا الحفاظ على الخصوصية، من المهم فهم تطور التكنولوجيا وتأثيرها على المجتمع.
PrivacyPreserving Machine arning: 익명 및 데이터 보안 ML 보존을위한 실용적인 안내서 = 오늘날 우리가 살고있는 세상은 부모 나 조부모가 자란 세상과 크게 다릅니다. 특히 기계 학습 (ML) 분야에서 기술의 빠른 개발로 인해 기밀 유지가 점점 어려워지고 있습니다. 우리의 개인 정보는 전례없는 속도로 수집, 저장 및 분석되어 신원 도용, 사이버 공격 및 기타 형태의 착취에 취약합니다. 결과적으로 ML의 이점을 유지하면서 민감한 데이터를 보호 할 수있는 개인 정보 보호 기술이 점점 더 필요합니다. 이 책에서 우리는 복잡한 개인 정보 보호 기술 세계를 살펴보고 얼굴 인식, 클라우드 스토리지 등을위한 실제 사용 사례를 살펴 봅니다. 우리는 이러한 기술을 해체하고 기술 구현을위한 실용적인 기술을 제공하여 ML 시스템이 효율적이고 안전하도록 할 것입니다. 현재 및 미래의 머신 러닝 개인 정보 보호 문제와 특정 요구에 맞는 기술을 조정하는 방법에 대해 배울 수 있습니 이 튜토리얼이 끝나면 데이터 품질이나 모델 성능을 손상시키지 않으면 서 사용자 개인 정보를 유지하는 ML 시스템을 만들 수 있습니다 기술의 진화를 이해하기 전에 개인 정보 보호 기술의 축소에 뛰어 들기 전에 기술의 진화와 사회에 미치는 영향을 이해하는 것이 중요합니다.
PrivacyPreserving Machine arning:匿名性とデータセキュリティを維持するための実用的なガイドML=私たちが今日住んでいる世界は、両親や祖父母が育ったものとは大きく異なります。特に機械学習(ML)分野における技術の急速な発展に伴い、機密性を維持することはますます困難になります。当社の個人情報は、これまでにない速度で収集、保存、分析され、個人情報の盗難、サイバー攻撃、その他の形態の搾取に対して脆弱性があります。その結果、MLの利点を維持しながら機密データを保護できるプライバシー保護技術のニーズが高まっています。この本では、プライバシーを強化する技術の複雑な世界を見て、顔認識、クラウドストレージなどの実際のユースケースを見てみましょう。当社は、これらの技術を分解し、技術実装のための実践的なスキルを提供し、お客様のMLシステムが効率的かつ安全であることを保証します。現在および将来の機械学習のプライバシーに関する問題と、特定のニーズに合わせてテクノロジーを調整する方法について学びます。このチュートリアルの最後までに、データ品質やモデルパフォーマンスを損なうことなく、ユーザーのプライバシーを維持するMLシステムを作成できます。技術の進化を理解するプライバシー保護技術のminutiaeに飛び込む前に、技術の進化と社会への影響を理解することが重要です。
隱私保護機器學習:保持匿名性和數據安全的實用指南ML=我們今天生活的世界與我們父母或祖父母長大的世界截然不同。隨著技術的飛速發展,特別是在機器學習(ML)領域,隱私變得越來越困難。我們的個人信息以前所未有的速度收集、存儲和分析,使我們容易受到身份盜竊、網絡攻擊和其他形式的剝削。因此,人們越來越需要能夠保護我們敏感數據的保密技術,同時保持ML的優勢。在本書中,我們將了解一個復雜的技術世界,提高隱私,並考慮使用面部識別、雲存儲等的真實情況。我們將對這些技術進行消毒,並為技術實施提供實用技能,確保您的ML系統高效安全。您將了解當前和未來的機器學習隱私問題,以及如何根據您的特定需求調整技術。在此指南的結尾,您可以創建可保護用戶隱私的ML系統,而不影響數據質量和模型性能。了解技術進化在深入研究小型保密技術之前,了解技術的進化及其對社會的影響非常重要。
