
BOOKS - PROGRAMMING - Handbook of Evolutionary Machine Learning

Handbook of Evolutionary Machine Learning
Author: Wolfgang Banzhaf, Penousal Machado
Year: 2024
Pages: 764
Format: PDF
File size: 15.6 MB
Language: ENG
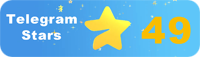
Year: 2024
Pages: 764
Format: PDF
File size: 15.6 MB
Language: ENG
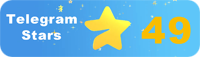
Handbook of Evolutionary Machine Learning Introduction: The Handbook of Evolutionary Machine Learning is a comprehensive guide to the application of evolutionary approaches in the field of machine learning. This book, written by leading international researchers, explores the various ways in which evolution can be used to address machine learning problems and improve current methods. The book is divided into five parts, each focusing on a different aspect of evolutionary machine learning. Part I: Fundamentals and Overviews This part introduces the reader to some fundamental concepts and overviews of evolutionary approaches to the three different classes of learning employed in machine learning. These include supervised learning, unsupervised learning, and reinforcement learning. Each chapter provides an in-depth look at the underlying principles of these learning methods and how evolutionary techniques can be applied to improve their performance. Part II: Evolutionary Computation as a Machine Learning Technique In this part, the authors describe methodological improvements for evolutionary clustering, classification, regression, and ensemble learning. These chapters provide a detailed explanation of how evolutionary computation can be used to optimize machine learning algorithms and achieve better results. Part III: Connection between Evolution and Neural Networks This part delves into the connection between evolution and neural networks, specifically exploring the relationship between deep learning, generative, and adversarial models. The authors also discuss the exciting potential of evolution with large language models, providing insights into the future of machine learning.
Handbook of Evolutionary Machine arning Introduction: The Handbook of Evolutionary Machine arning - всеобъемлющее руководство по применению эволюционных подходов в области машинного обучения. Эта книга, написанная ведущими международными исследователями, исследует различные способы, с помощью которых эволюция может быть использована для решения проблем машинного обучения и улучшения современных методов. Книга разделена на пять частей, каждая из которых посвящена различным аспектам эволюционного машинного обучения. Часть I: Основы и обзоры Эта часть знакомит читателя с некоторыми фундаментальными концепциями и обзорами эволюционных подходов к трем различным классам обучения, используемым в машинном обучении. К ним относятся обучение с учителем, обучение без учителя и обучение с подкреплением. В каждой главе подробно рассматриваются основные принципы этих методов обучения и способы применения эволюционных методов для повышения их эффективности. Часть II: Эволюционные вычисления как техника машинного обучения В этой части авторы описывают методологические улучшения для эволюционной кластеризации, классификации, регрессии и обучения ансамблей. Эти главы дают подробное объяснение того, как эволюционные вычисления могут быть использованы для оптимизации алгоритмов машинного обучения и достижения лучших результатов. Часть III: Связь между эволюцией и нейронными сетями Эта часть углубляется в связь между эволюцией и нейронными сетями, в частности исследуя связь между глубоким обучением, генеративными и состязательными моделями. Авторы также обсуждают захватывающий потенциал эволюции с большими языковыми моделями, предоставляя представление о будущем машинного обучения.
Handbook of Evolutionary Machine arning Introduction : The Handbook of Evolutionary Machine arning est un guide complet pour l'application d'approches évolutionnaires dans le domaine de l'apprentissage automatique. Ce livre, écrit par des chercheurs internationaux de premier plan, explore les différentes façons dont l'évolution peut être utilisée pour résoudre les problèmes de l'apprentissage automatique et améliorer les méthodes modernes. livre est divisé en cinq parties, chacune traitant de différents aspects de l'apprentissage automatique évolutionnaire. Partie I : Bases et revues Cette partie présente au lecteur certains concepts fondamentaux et des revues des approches évolutionnaires des trois différentes classes d'apprentissage utilisées dans l'apprentissage automatique. Il s'agit notamment d'un enseignement avec un enseignant, d'un enseignement sans professeur et d'un enseignement renforcé. Chaque chapitre traite en détail des principes fondamentaux de ces méthodes d'apprentissage et de la façon dont les méthodes évolutionnaires sont appliquées pour améliorer leur efficacité. Partie II : calcul évolutif en tant que technique d'apprentissage automatique Dans cette partie, les auteurs décrivent des améliorations méthodologiques pour le regroupement évolutionnaire, la classification, la régression et l'apprentissage des ensembles. Ces chapitres fournissent une explication détaillée de la façon dont les calculs évolutionnaires peuvent être utilisés pour optimiser les algorithmes d'apprentissage automatique et obtenir de meilleurs résultats. Partie III : lien entre l'évolution et les réseaux neuronaux Cette partie s'approfondit dans le lien entre l'évolution et les réseaux neuronaux, notamment en explorant le lien entre l'apprentissage profond, les modèles génératifs et compétitifs. s auteurs discutent également du potentiel passionnant de l'évolution avec de grands modèles linguistiques, donnant une idée de l'avenir de l'apprentissage automatique.
Handbook of Evolutionary Machine arning Introduction: The Handbook of Evolutionary Machine arning es una guía completa para la aplicación de enfoques evolutivos en el aprendizaje automático. Este libro, escrito por destacados investigadores internacionales, explora las diferentes formas en las que se puede utilizar la evolución para resolver los problemas del aprendizaje automático y mejorar los métodos modernos. libro se divide en cinco partes, cada una dedicada a diferentes aspectos del aprendizaje automático evolutivo. Parte I: Fundamentos y Reseñas Esta parte presenta al lector algunos conceptos fundamentales y reseñas de los enfoques evolutivos de las tres clases diferentes de aprendizaje utilizadas en el aprendizaje automático. Entre ellas, la formación con el profesor, la formación sin profesor y la formación con refuerzos. Cada capítulo examina en detalle los principios básicos de estos métodos de enseñanza y cómo aplicar métodos evolutivos para mejorar su eficacia. Parte II: Computación evolutiva como técnica de aprendizaje automático En esta parte, los autores describen mejoras metodológicas para la agrupación evolutiva, clasificación, regresión y aprendizaje de conjuntos. Estos capítulos proporcionan una explicación detallada de cómo se pueden utilizar los cálculos evolutivos para optimizar los algoritmos de aprendizaje automático y lograr mejores resultados. Parte III: La relación entre la evolución y las redes neuronales Esta parte profundiza en la relación entre la evolución y las redes neuronales, en particular investigando la relación entre el aprendizaje profundo, los modelos generativos y competitivos. autores también discuten el emocionante potencial de la evolución con grandes modelos lingüísticos, proporcionando una visión del futuro del aprendizaje automático.
Handbook of Evolutionary Machine arning Intrudation: The Handbook of Evolutionary Machine arning é um guia abrangente para a aplicação de abordagens evolucionárias de aprendizagem automática. Este livro, escrito pelos principais pesquisadores internacionais, explora as diferentes formas pelas quais a evolução pode ser usada para resolver problemas de aprendizagem de máquinas e melhorar os métodos modernos. O livro é dividido em cinco partes, cada uma delas sobre diferentes aspectos do aprendizado da máquina evolucionária. Parte I: Fundamentos e revisões Esta parte apresenta ao leitor alguns conceitos fundamentais e revisões de abordagens evolutivas para três diferentes classes de aprendizagem usadas na aprendizagem de máquinas. Eles incluem a formação com um professor, a formação sem professor e a formação com reforços. Cada capítulo aborda em detalhe os princípios básicos desses métodos de aprendizagem e as formas de aplicar métodos evolucionários para aumentar a sua eficácia. Parte II: Computação evolucionária como técnica de aprendizado de máquina Nesta parte, os autores descrevem melhorias metodológicas para clusterização evolucionária, classificação, regressão e treinamento dos conjuntos. Estes capítulos fornecem uma explicação detalhada de como a computação evolutiva pode ser usada para otimizar algoritmos de aprendizagem automática e alcançar melhores resultados. Parte III: A relação entre a evolução e as redes neurais Esta parte aprofunda-se na relação entre a evolução e as redes neurais, especialmente explorando a relação entre o aprendizado profundo, os modelos genéricos e concorrentes. Os autores também discutem o potencial emocionante da evolução com grandes modelos linguísticos, fornecendo uma visão do futuro da aprendizagem de máquinas.
Handbook of Evolutionary Machine arning Introduction: The Handbook of Evolutionary Machine arning è una guida completa per l'applicazione degli approcci evolutivi nell'apprendimento automatico. Questo libro, scritto da ricercatori internazionali di primo piano, esamina diversi modi in cui l'evoluzione può essere utilizzata per risolvere i problemi dell'apprendimento automatico e migliorare i metodi moderni. Il libro è suddiviso in cinque parti, ognuna dedicata a diversi aspetti dell'apprendimento automatico evolutivo. Parte I: Basi e recensioni Questa parte presenta al lettore alcuni concetti fondamentali e le recensioni degli approcci evolutivi alle tre diverse classi di apprendimento utilizzate nell'apprendimento automatico. Questi includono l'apprendimento con un insegnante, l'apprendimento senza un insegnante e l'apprendimento con rinforzi. Ogni capitolo descrive in dettaglio i principi fondamentali di questi metodi di apprendimento e le modalità di applicazione delle tecniche evolutive per migliorarne l'efficacia. Parte II: il calcolo evolutivo come tecnica di apprendimento automatico In questa parte, gli autori descrivono i miglioramenti metodologici per il clustering evolutivo, la classificazione, la regressione e l'apprendimento dei gruppi. Questi capitoli forniscono una spiegazione dettagliata di come i calcoli evolutivi possano essere utilizzati per ottimizzare gli algoritmi di apprendimento automatico e ottenere risultati migliori. Parte III: La connessione tra l'evoluzione e le reti neurali Questa parte si approfondisce nel legame tra l'evoluzione e le reti neurali, in particolare esplorando il legame tra l'apprendimento profondo, i modelli generali e quelli competitivi. Gli autori discutono anche del potenziale eccitante dell'evoluzione con modelli linguistici di grandi dimensioni, fornendo un'idea del futuro dell'apprendimento automatico.
Handbuch des evolutionären maschinellen rnens Einführung: Das Handbuch des evolutionären maschinellen rnens ist eine umfassende Anleitung zur Anwendung evolutionärer Ansätze im Bereich des maschinellen rnens. Dieses Buch, das von führenden internationalen Forschern geschrieben wurde, untersucht verschiedene Möglichkeiten, wie die Evolution genutzt werden kann, um Probleme des maschinellen rnens zu lösen und moderne Techniken zu verbessern. Das Buch ist in fünf Teile unterteilt, die sich jeweils mit verschiedenen Aspekten des evolutionären maschinellen rnens befassen. Teil I: Grundlagen und Übersichten Dieser Teil führt den ser in einige grundlegende Konzepte und Übersichten evolutionärer Ansätze zu drei verschiedenen rnklassen ein, die im maschinellen rnen verwendet werden. Dazu gehören das rnen mit dem hrer, das rnen ohne hrer und das rnen mit Verstärkung. In jedem Kapitel werden die Grundprinzipien dieser hrmethoden und die Art und Weise, wie evolutionäre Methoden angewendet werden, um ihre Wirksamkeit zu verbessern, ausführlich behandelt. Teil II: Evolutionäres Rechnen als maschinelle rntechnik In diesem Teil beschreiben die Autoren methodische Verbesserungen für evolutionäres Clustering, Klassifikation, Regression und Ensembletraining. Diese Kapitel bieten eine detaillierte Erklärung, wie evolutionäre Berechnungen verwendet werden können, um Algorithmen für maschinelles rnen zu optimieren und bessere Ergebnisse zu erzielen. Teil III: Die Verbindung zwischen Evolution und neuronalen Netzen Dieser Teil vertieft die Verbindung zwischen Evolution und neuronalen Netzen und untersucht insbesondere die Verbindung zwischen Deep arning, generativen und kontradiktorischen Modellen. Die Autoren diskutieren auch das spannende Potenzial der Evolution mit großen Sprachmodellen und geben einen Einblick in die Zukunft des maschinellen rnens.
Podręcznik ewolucyjnego uczenia maszynowego Wprowadzenie: Podręcznik ewolucyjnego uczenia maszynowego jest kompleksowym przewodnikiem do stosowania ewolucyjnych podejść do uczenia się maszynowego. Książka ta, napisana przez czołowych międzynarodowych naukowców, bada różne sposoby ewolucji, które można wykorzystać do rozwiązywania problemów z nauką maszynową i doskonalenia obecnych metod. Książka podzielona jest na pięć części, z których każda skupia się na różnych aspektach ewolucyjnego uczenia się maszynowego. Część I: Podstawy i recenzje Ta część wprowadza czytelnika do niektórych podstawowych koncepcji i recenzji ewolucyjnych podejść do trzech różnych klas uczenia się stosowanych w nauce maszynowej. Obejmują one nadzorowane uczenie się, uczenie się bez nadzoru i uczenie się wzmacniające. Każdy rozdział wyszczególnia podstawowe zasady tych metod nauczania oraz sposób stosowania metod ewolucyjnych w celu poprawy ich skuteczności. Część II: Informatyka ewolucyjna jako technika uczenia maszynowego W tej części autorzy opisują ulepszenia metodologiczne dla klastrowania ewolucyjnego, klasyfikacji, regresji i uczenia się zespołu. Rozdziały te zawierają szczegółowe wyjaśnienie, w jaki sposób można wykorzystać komputery ewolucyjne do optymalizacji algorytmów uczenia maszynowego i osiągania lepszych wyników. Część III: Związek między ewolucją a sieciami neuronowymi Ta część odkrywa związek między ewolucją a sieciami neuronowymi, w szczególności badając związek między modelami głębokiego uczenia się, generatywnymi i przeciwnymi. Autorzy omawiają również ekscytujący potencjał ewolucji z dużymi modelami językowymi, zapewniając wgląd w przyszłość nauki maszynowej.
Handbook of Evolutionary Machine arning Introduction: The Handbook of Evolutionary Machine arning הוא מדריך מקיף ליישום גישות אבולוציוניות ללימוד מכונה. ספר זה, שנכתב על ידי חוקרים בינלאומיים מובילים, בוחן את הדרכים השונות בהן ניתן להשתמש באבולוציה כדי לפתור בעיות למידה של מכונות ולשפר שיטות עכשוויות. הספר מחולק לחמישה חלקים, וכל אחד מהם מתמקד בהיבטים שונים של למידת מכונה אבולוציונית. חלק I: יסודות וביקורות חלק זה מציג לקורא כמה מושגים בסיסיים וביקורות על גישות אבולוציוניות הם כוללים למידה מפוקחת, למידה ללא השגחה ולימוד חיזוק. כל פרק מפרט את העקרונות הבסיסיים של שיטות הוראה אלה וכיצד ניתן ליישם שיטות אבולוציוניות כדי לשפר את יעילותן. חלק II: מחשוב אבולוציוני כטכניקת למידת מכונה בחלק זה, המחברים מתארים שיפורים מתודולוגיים לקיבוצים אבולוציוניים, סיווג, רגרסיה ולימוד אנסמבל. פרקים אלה מספקים הסבר מפורט כיצד ניתן להשתמש במחשוב אבולוציוני כדי לייעל אלגוריתמי למידת מכונה ולהשיג תוצאות טובות יותר. חלק III: הקשר בין אבולוציה לרשתות עצביות חלק זה מתעמק בקשר בין אבולוציה ורשתות עצביות, במיוחד לחקור את הקשר בין למידה עמוקה, מודלים מחוללים ויריבים. המחברים גם דנים בפוטנציאל המרגש של האבולוציה עם מודלים גדולים בשפה, ומספקים תובנה לגבי העתיד של למידת מכונה.''
Handbook of Evolutionary Machine arning Giriş: The Handbook of Evolutionary Machine arning, evrimsel yaklaşımları makine öğrenimine uygulamak için kapsamlı bir kılavuzdur. Önde gelen uluslararası araştırmacılar tarafından yazılan bu kitap, evrimin makine öğrenme problemlerini çözmek ve mevcut yöntemleri geliştirmek için kullanılabileceği farklı yolları araştırıyor. Kitap, her biri evrimsel makine öğreniminin farklı yönlerine odaklanan beş bölüme ayrılmıştır. Bölüm I: Temel Bilgiler ve İncelemeler Bu bölüm, okuyucuyu, makine öğrenmesinde kullanılan üç farklı öğrenme sınıfına evrimsel yaklaşımların bazı temel kavramlarına ve incelemelerine tanıtır. Bunlar denetimli öğrenme, denetimsiz öğrenme ve takviye öğrenmeyi içerir. Her bölüm, bu öğretim yöntemlerinin temel ilkelerini ve bunların etkinliğini artırmak için evrimsel yöntemlerin nasıl uygulanabileceğini detaylandırmaktadır. Bölüm II: Bir Makine Öğrenme Tekniği Olarak Evrimsel Hesaplama Bu bölümde, yazarlar evrimsel kümeleme, sınıflandırma, regresyon ve topluluk öğrenimi için metodolojik gelişmeleri tanımlamaktadır. Bu bölümler, makine öğrenimi algoritmalarını optimize etmek ve daha iyi sonuçlar elde etmek için evrimsel hesaplamanın nasıl kullanılabileceğine dair ayrıntılı bir açıklama sunmaktadır. Bölüm III: Evrim ve sinir ağları arasındaki bağlantı Bu bölüm, evrim ve sinir ağları arasındaki bağlantıyı, özellikle derin öğrenme, üretici ve rakip modeller arasındaki bağlantıyı araştırır. Yazarlar ayrıca, evrimin heyecan verici potansiyelini büyük dil modelleriyle tartışarak, makine öğreniminin geleceği hakkında fikir veriyor.
Handbook of Evolutionary Machine arning Introduction: The Handbook of Evolutionary Machine arning هو دليل شامل لتطبيق الأساليب التطورية على التعلم الآلي. يستكشف هذا الكتاب، الذي كتبه باحثون دوليون بارزون، الطرق المختلفة التي يمكن بها استخدام التطور لحل مشاكل التعلم الآلي وتحسين الأساليب الحالية. ينقسم الكتاب إلى خمسة أجزاء، يركز كل منها على جوانب مختلفة من التعلم الآلي التطوري. الجزء الأول: الأساسيات والمراجعات يقدم هذا الجزء للقارئ بعض المفاهيم والمراجعات الأساسية للمناهج التطورية لفئات التعلم الثلاث المختلفة المستخدمة في التعلم الآلي. وتشمل هذه التعلم تحت الإشراف والتعلم بدون إشراف والتعلم المعزز. يوضح كل فصل المبادئ الأساسية لطرق التدريس هذه وكيف يمكن تطبيق الأساليب التطورية لتحسين فعاليتها. الجزء الثاني: الحوسبة التطورية كتقنية للتعلم الآلي في هذا الجزء، يصف المؤلفون التحسينات المنهجية للتجميع التطوري والتصنيف والانحدار والتعلم الجماعي. تقدم هذه الفصول شرحًا مفصلاً لكيفية استخدام الحوسبة التطورية لتحسين خوارزميات التعلم الآلي وتحقيق نتائج أفضل. الجزء الثالث: الصلة بين التطور والشبكات العصبية يتعمق هذا الجزء في الصلة بين التطور والشبكات العصبية، وتحديداً استكشاف الصلة بين التعلم العميق والنماذج التوليدية والخصومية. يناقش المؤلفون أيضًا الإمكانات المثيرة للتطور مع نماذج لغوية كبيرة، مما يوفر نظرة ثاقبة لمستقبل التعلم الآلي.
진화론 적 머신 러닝 소개 핸드북: 진화론 적 머신 러닝 핸드북은 머신 러닝에 진화 적 접근 방식을 적용하는 포괄적 인 가이드입니다. 주요 국제 연구자들이 저술 한이 책은 진화가 기계 학습 문제를 해결하고 현재의 방법을 개선하는 데 사용될 수있는 다양한 방법을 탐구합니 이 책은 진화 기계 학습의 여러 측면에 중점을 둔 다섯 부분으로 나뉩니다. 파트 I: 기본 및 리뷰 이 부분은 기계 학습에 사용되는 세 가지 다른 학습 클래스에 대한 진화 적 접근 방식에 대한 몇 가지 기본 개념과 리뷰를 독자에게 소개합니다. 여기에는 감독 학습, 감독되지 않은 학습 및 강화 학습이 포함됩니다. 각 장에서는 이러한 교수법의 기본 원리와 효과를 향상시키기 위해 진화 방법을 적용하는 방법에 대해 자세히 설명합니다. 파트 II: 기계 학습 기술로서의 진화 컴퓨팅 이 부분에서 저자는 진화 클러스터링, 분류, 회귀 및 앙상블 학습에 대한 방법 론적 개선을 설명합니다. 이 장은 진화 컴퓨팅을 사용하여 머신 러닝 알고리즘을 최적화하고 더 나은 결과를 얻는 방법에 대한 자세한 설명을 제공합니다. 파트 III: 진화와 신경망 사이의 연결 이 부분은 진화와 신경망 사이의 연결, 특히 딥 러닝, 생성 및 적대적 모델 사이의 연결을 탐구합니다. 저자는 또한 큰 언어 모델로 진화의 흥미로운 잠재력에 대해 논의하여 머신 러닝의 미래에 대한 통찰력을 제공합니다.
Handbook of Evolutionary Machine arningはじめに:The Handbook of Evolutionary Machine arningは、進化的アプローチを機械学習に適用するための包括的なガイドです。世界をリードする研究者によって書かれたこの本は、機械学習の問題を解決し、現在の方法を改善するために進化が使用できるさまざまな方法を探求しています。本は5つの部分に分かれており、それぞれ進化的機械学習のさまざまな側面に焦点を当てています。パートI:基礎とレビューこのパートでは、機械学習で使用される3つの異なるクラスの学習に対する進化的アプローチの基本的な概念とレビューを読者に紹介します。これらには、監督された学習、監視されていない学習、強化学習が含まれます。各章では、これらの教授法の基本的な原則と、その有効性を向上させるためにどのように進化的な方法を適用できるかについて詳述しています。Part II: Evolutionary Computing as a Machine arning Techniqueこのパートでは、進化クラスタリング、分類、回帰、アンサンブル学習の方法論的改善について説明している。これらの章では、機械学習アルゴリズムを最適化し、より良い結果を得るために進化的コンピューティングをどのように使用できるかについて詳しく説明します。Part III:進化とニューラルネットワークのつながりこの部分は、進化とニューラルネットワークのつながりを掘り下げ、特にディープラーニング、生成モデル、敵対モデルのつながりを探求します。また、大きな言語モデルを用いて、進化のエキサイティングな可能性について議論し、機械学習の将来についての洞察を提供した。
