
BOOKS - Machine Learning Approaches in Financial Analytics

Machine Learning Approaches in Financial Analytics
Author: Leandros A. Maglaras, Sonali Das, Naliniprava Tripathy, Srikanta Patnaik
Year: 2024
Pages: 485
Format: PDF | EPUB
File size: 53.1 MB
Language: ENG
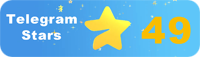
Year: 2024
Pages: 485
Format: PDF | EPUB
File size: 53.1 MB
Language: ENG
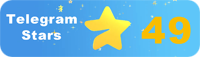
The book "Machine Learning Approaches in Financial Analytics" explores the use of machine learning techniques in financial analytics, providing insights into how these methods can be applied to real-world problems. The book covers topics such as supervised and unsupervised learning, deep learning, natural language processing, and reinforcement learning, and their applications in finance. It also discusses the challenges and limitations of these approaches and provides practical examples of their implementation in financial institutions. The author emphasizes the importance of understanding the process of technological evolution and its impact on society, arguing that this understanding is essential for the survival of humanity and the unity of people in a world torn apart by conflict. He suggests that developing a personal paradigm for perceiving the technological process of developing modern knowledge is crucial for navigating the complex and rapidly changing landscape of technology. The book begins with an introduction to machine learning and its relevance to financial analytics, highlighting the need for a comprehensive understanding of the field. The author then delves into the various machine learning approaches and their applications in finance, including predictive modeling, risk management, and portfolio optimization. The book also covers the challenges associated with implementing machine learning in finance, such as data quality issues and the need for domain expertise.
Книга «Подходы машинного обучения в финансовой аналитике» исследует использование методов машинного обучения в финансовой аналитике, предоставляя понимание того, как эти методы могут быть применены к реальным проблемам. Книга охватывает такие темы, как контролируемое и неконтролируемое обучение, глубокое обучение, обработка естественного языка и обучение с подкреплением, а также их применение в финансах. В нем также обсуждаются проблемы и ограничения этих подходов и приводятся практические примеры их реализации в финансовых учреждениях. Автор подчеркивает важность понимания процесса технологической эволюции и его влияния на общество, утверждая, что это понимание необходимо для выживания человечества и единства людей в мире, раздираемом конфликтами. Он предполагает, что разработка личной парадигмы восприятия технологического процесса развития современных знаний имеет решающее значение для навигации по сложному и быстро меняющемуся ландшафту технологий. Книга начинается с введения в машинное обучение и его соответствия финансовой аналитике, подчеркивая необходимость всестороннего понимания этой области. Затем автор углубляется в различные подходы машинного обучения и их применения в финансах, включая прогнозное моделирование, управление рисками и оптимизацию портфеля. Книга также охватывает проблемы, связанные с внедрением машинного обучения в финансах, такие как вопросы качества данных и необходимость экспертизы в области.
''
