
BOOKS - Effective XGBoost Optimizing, Tuning, Understanding, and Deploying Classifica...

Effective XGBoost Optimizing, Tuning, Understanding, and Deploying Classification Models
Author: Matt Harrison
Year: 2023
Pages: 221
Format: PDF
File size: 28.9 MB
Language: ENG
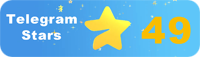
Year: 2023
Pages: 221
Format: PDF
File size: 28.9 MB
Language: ENG
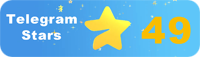
Book Description: 'Effective XGBoost Optimizing Tuning Understanding and Deploying Classification Models' is a comprehensive guide to optimizing and deploying XGBoost models for classification tasks. The book covers the entire process of XGBoost model development, from data preparation to model deployment, and provides practical tips and techniques for improving model performance. It also discusses the importance of understanding the underlying principles of XGBoost and how to apply them in real-world applications. The book begins by introducing the concept of XGBoost and its advantages over other machine learning algorithms. It then delves into the details of data preparation, feature selection, and model training, providing readers with a solid foundation in the basics of XGBoost model development. From there, the book moves on to more advanced topics such as hyperparameter tuning, model evaluation, and deployment strategies. Throughout the book, the author emphasizes the importance of understanding the underlying principles of XGBoost and how to apply them in real-world applications. The book also includes numerous examples and case studies to illustrate the concepts discussed. The book concludes with a discussion on the future of XGBoost and its potential applications in emerging technologies such as deep learning and big data analytics. Book Outline: I.
'Effective XGBoost Optimizing Tuning Understanding and Deployment Classification Models'- всеобъемлющее руководство по оптимизации и развертыванию моделей XGBoost для задач классификации. Книга охватывает весь процесс разработки модели XGBoost, от подготовки данных до развертывания модели, и содержит практические советы и методы для повышения производительности модели. Также обсуждается важность понимания основных принципов XGBoost и их применения в реальных приложениях. Книга начинается с введения понятия XGBoost и его преимуществ перед другими алгоритмами машинного обучения. Затем он углубляется в детали подготовки данных, выбора функций и обучения моделей, предоставляя читателям прочную основу в основах разработки моделей XGBoost. Оттуда книга переходит к более продвинутым темам, таким как настройка гиперпараметров, оценка моделей и стратегии развертывания. На протяжении всей книги автор подчеркивает важность понимания основополагающих принципов XGBoost и того, как применять их в реальных приложениях. Книга также включает многочисленные примеры и тематические исследования для иллюстрации обсуждаемых концепций. Книга завершается обсуждением будущего XGBoost и его потенциальных приложений в развивающихся технологиях, таких как глубокое обучение и аналитика больших данных. Очерк книги: И.
''
